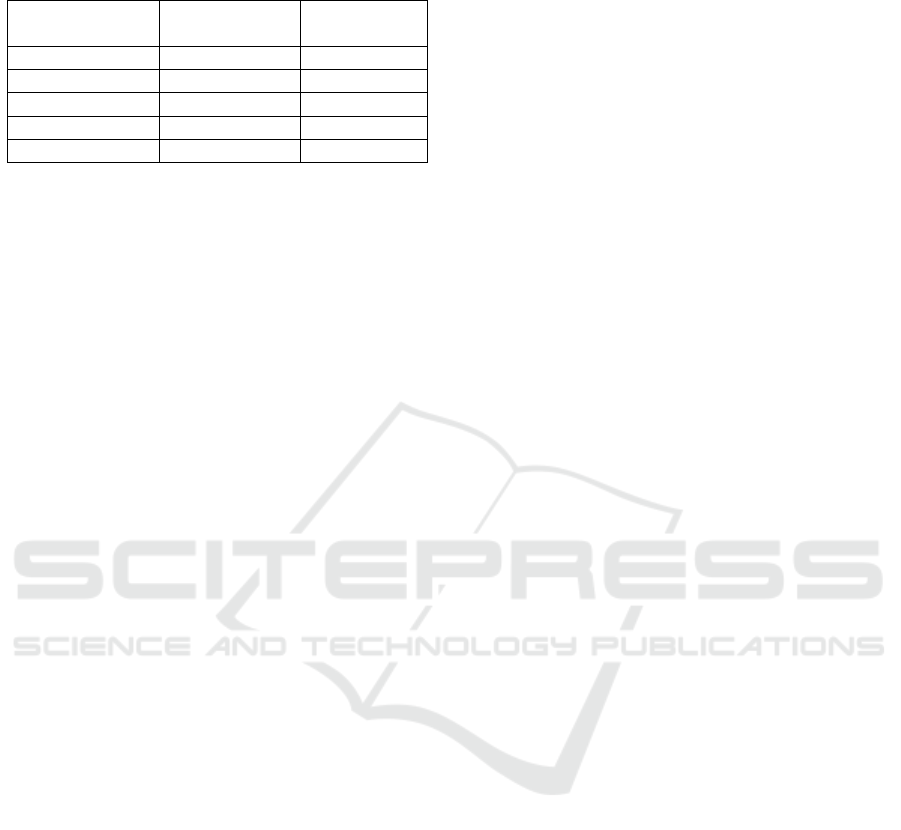
Table 1: Comparison of restoration accuracy (RMSE) by
removal of specular reflection component
before removal after removal
(m) (m)
Smooth surface 4.759 1.555
Rough surface 8.068 1.716
Lattice surface 9.325 2.474
Mirror surface 7.633 1.853
Concave surface 7.412 1.989
smooth surface, rough surface, lattice surface, sur-
face with strong specular reflection component (mir-
ror surface), and concave surface. For removing the
specular components by using the method shown in
section 4, network training was performed with 720
training data and 180 test data.
Fig. 6 (b) and (c) show the observed images be-
fore the specular component removal, and Fig. 6 (d)
shows the estimated results of the 3D light source
positions before and after light source motion. The
points and arrows show the position and motion of the
light sources. Light and dark colors represent the first
and second light sources respectively. The green ar-
rows represent the ground truth light source motions,
the blue arrows represent the light source motions re-
covered from the images before the specular compo-
nent removal, and the red arrows represent the light
source motions recovered from the images after the
specular component removal. As shown in this fig-
ure, the proposed method can recover the occluded
light source positions and motions from the indirect
intensity on many different types of walls. This is
because the proposed method uses the reflectance in-
variant for estimating the occluded light sources. In
particular, the red arrows are closer to the green ar-
rows, so we find that the specular component removal
is effective in our method.
Table 1 compares the RMSE of the results recov-
ered from the images before and after the specular
component removal for each intermediate observation
surface. From this table, we find that the accuracy
of the estimation is drastically improved by removing
the specular components using the method shown in
section 4.
6 CONCLUSIONS
In this paper, we proposed a method for recovering
the 3D structure and luminance distribution of lumi-
nous objects that cannot be directly observed from the
camera. For this objective, we modeled the observa-
tion process of the light emitted from a luminous ob-
ject, reflecting off walls and floors and reaching the
camera. Then, we showed that 3D shape and lumi-
nance distribution can be estimated simultaneously by
using images obtained at multiple time instants. Ex-
periments with synthetic and real images confirmed
that the proposed method works under many different
types of intermediate walls.
REFERENCES
Baradad, M., Ye, V., Yedidia, A. B., Durand, F., Freeman,
W. T., Wornell, G. W., and Torralba, A. (2018). In-
ferring light fields from shadows. In Proceedings of
the IEEE Conference on Computer Vision and Pattern
Recognition, pages 6267–6275.
Bouman, K. L., Ye, V., Yedidia, A. B., Durand, F., Wornell,
G. W., Torralba, A., and Freeman, W. T. (2017). Turn-
ing corners into cameras: Principles and methods. In
Proceedings of the IEEE International Conference on
Computer Vision, pages 2270–2278.
Chen, W., Daneau, S., Mannan, F., and Heide, F. (2019).
Steady-state non-line-of-sight imaging. In Proceed-
ings of the IEEE/CVF Conference on Computer Vision
and Pattern Recognition, pages 6790–6799.
Isola, P., Zhu, J.-Y., Zhou, T., and Efros, A. A. (2017).
Image-to-image translation with conditional adversar-
ial networks. In Proceedings of the IEEE conference
on computer vision and pattern recognition, pages
1125–1134.
Kaga, M., Kushida, T., Takatani, T., Tanaka, K., Funatomi,
T., and Mukaigawa, Y. (2019). Thermal non-line-of-
sight imaging from specular and diffuse reflections.
IPSJ Transactions on Computer Vision and Applica-
tions, 11(1):1–6.
Maeda, T., Wang, Y., Raskar, R., and Kadambi, A. (2019).
Thermal non-line-of-sight imaging. In 2019 IEEE In-
ternational Conference on Computational Photogra-
phy (ICCP), pages 1–11. IEEE.
Mirza, M. and Osindero, S. (2014). Conditional generative
adversarial nets. arXiv preprint arXiv:1411.1784.
Saunders, C., Murray-Bruce, J., and Goyal, V. K. (2019).
Computational periscopy with an ordinary digital
camera. Nature, 565(7740):472–475.
Velten, A., Willwacher, T., Gupta, O., Veeraraghavan, A.,
Bawendi, M. G., and Raskar, R. (2012). Recovering
three-dimensional shape around a corner using ultra-
fast time-of-flight imaging. Nature communications,
3(1):1–8.
Yedidia, A. B., Baradad, M., Thrampoulidis, C., Freeman,
W. T., and Wornell, G. W. (2019). Using unknown
occluders to recover hidden scenes. In Proceedings
of the IEEE/CVF Conference on Computer Vision and
Pattern Recognition, pages 12231–12239.
VISAPP 2023 - 18th International Conference on Computer Vision Theory and Applications
996