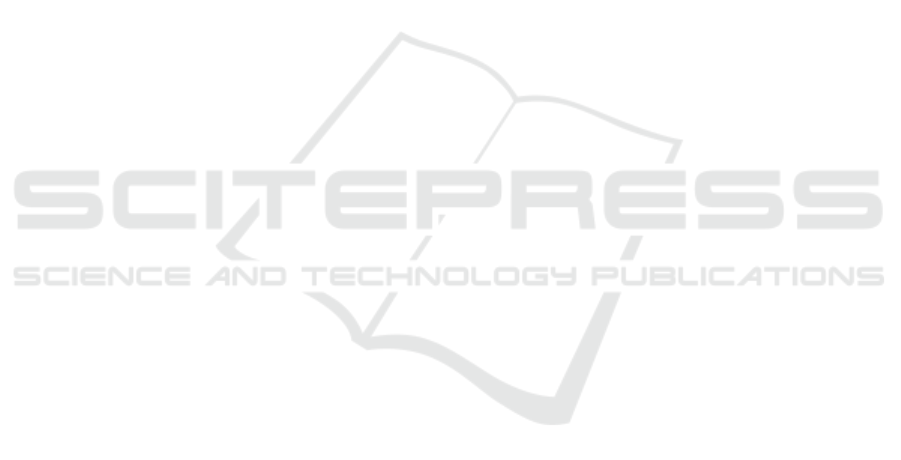
Hewitt, J., Ethayarajh, K., Liang, P., and Manning, C. D.
(2021). Conditional probing: measuring usable infor-
mation beyond a baseline. In Moens, M., Huang, X.,
Specia, L., and Yih, S. W., editors, Proceedings of
the 2021 Conference on Empirical Methods in Natu-
ral Language Processing, EMNLP 2021, Virtual Event
/ Punta Cana, Dominican Republic, 7-11 November,
2021, pages 1626–1639. Association for Computa-
tional Linguistics.
Hewitt, J. and Manning, C. D. (2019). A structural probe for
finding syntax in word representations. In Proc. Con-
ference of the North American Chapter of the Associa-
tion for Computational Linguistics: Human Language
Technologies, Vol. 1, pages 4129–4138.
Hu, J., Gauthier, J., Qian, P., Wilcox, E., and Levy, R. (2020).
A systematic assessment of syntactic generalization
in neural language models. In Jurafsky, D., Chai, J.,
Schluter, N., and Tetreault, J. R., editors, Proceed-
ings of the 58th Annual Meeting of the Association for
Computational Linguistics, ACL 2020, Online, July
5-10, 2020, pages 1725–1744. Association for Compu-
tational Linguistics.
Ivanova, A. A., Hewitt, J., and Zaslavsky, N. (2021). Probing
artificial neural networks: insights from neuroscience.
CoRR, abs/2104.08197.
Kirkpatrick, J., Pascanu, R., Rabinowitz, N., Veness, J., Des-
jardins, G., Rusu, A. A., Milan, K., Quan, J., Ramalho,
T., Grabska-Barwinska, A., et al. (2017). Overcoming
catastrophic forgetting in neural networks. Proc. of the
National Academy of Sciences, 114(13):3521–3526.
Kriegeskorte, N. and Douglas, P. K. (2019). Interpreting
encoding and decoding models. Current opinion in
neurobiology, 55:167–179.
Kuncoro, A., Dyer, C., Rimell, L., Clark, S., and Blunsom,
P. (2019). Scalable syntax-aware language models
using knowledge distillation. In Korhonen, A., Traum,
D. R., and Màrquez, L., editors, Proceedings of the
57th Conference of the Association for Computational
Linguistics, ACL 2019, Florence, Italy, July 28- August
2, 2019, Volume 1: Long Papers, pages 3472–3484.
Association for Computational Linguistics.
Limisiewicz, T. and Marecek, D. (2020). Syntax represen-
tation in word embeddings and neural networks - A
survey. In Holena, M., Horváth, T., Kelemenová, A.,
Mráz, F., Pardubská, D., Plátek, M., and Sosík, P., edi-
tors, Proceedings of the 20th Conference Information
Technologies - Applications and Theory (ITAT 2020),
Hotel Tyrapol, Oravská Lesná, Slovakia, September
18-22, 2020, volume 2718 of CEUR Workshop Pro-
ceedings, pages 40–50. CEUR-WS.org.
Liu, N. F., Gardner, M., Belinkov, Y., Peters, M. E., and
Smith, N. A. (2019). Linguistic knowledge and trans-
ferability of contextual representations. In Burstein,
J., Doran, C., and Solorio, T., editors, Proceedings of
the 2019 Conference of the North American Chapter
of the Association for Computational Linguistics: Hu-
man Language Technologies, NAACL-HLT 2019, Min-
neapolis, MN, USA, June 2-7, 2019, Volume 1 (Long
and Short Papers), pages 1073–1094. Association for
Computational Linguistics.
Merchant, A., Rahimtoroghi, E., Pavlick, E., and Tenney, I.
(2020). What happens to BERT embeddings during
fine-tuning? In Proceedings of the Third BlackboxNLP
Workshop on Analyzing and Interpreting Neural Net-
works for NLP, pages 33–44, Online. Association for
Computational Linguistics.
Mosbach, M., Khokhlova, A., Hedderich, M. A., and
Klakow, D. (2020). On the interplay between fine-
tuning and sentence-level probing for linguistic knowl-
edge in pre-trained transformers. In Alishahi, A., Be-
linkov, Y., Chrupala, G., Hupkes, D., Pinter, Y., and
Sajjad, H., editors, Proceedings of the Third Black-
boxNLP Workshop on Analyzing and Interpreting Neu-
ral Networks for NLP, BlackboxNLP@EMNLP 2020,
Online, November 2020, pages 68–82. Association for
Computational Linguistics.
Nikoulina, V., Tezekbayev, M., Kozhakhmet, N.,
Babazhanova, M., Gallé, M., and Assylbekov, Z.
(2021). The rediscovery hypothesis: Language models
need to meet linguistics. Journal of Artificial Intelli-
gence Research, 72:1343–1384.
Nivre, J., de Marneffe, M., Ginter, F., Hajic, J., Manning,
C. D., Pyysalo, S., Schuster, S., Tyers, F. M., and
Zeman, D. (2020). Universal dependencies v2: An
evergrowing multilingual treebank collection. In Cal-
zolari, N., Béchet, F., Blache, P., Choukri, K., Cieri,
C., Declerck, T., Goggi, S., Isahara, H., Maegaard,
B., Mariani, J., Mazo, H., Moreno, A., Odijk, J., and
Piperidis, S., editors, Proceedings of The 12th Lan-
guage Resources and Evaluation Conference, LREC
2020, Marseille, France, May 11-16, 2020, pages 4034–
4043. European Language Resources Association.
Pérez-Mayos, L., Ballesteros, M., and Wanner, L. (2021).
How much pretraining data do language models need to
learn syntax? In Moens, M., Huang, X., Specia, L., and
Yih, S. W., editors, Proceedings of the 2021 Conference
on Empirical Methods in Natural Language Processing,
EMNLP 2021, Virtual Event / Punta Cana, Dominican
Republic, 7-11 November, 2021, pages 1571–1582. As-
sociation for Computational Linguistics.
Pimentel, T., Valvoda, J., Maudslay, R. H., Zmigrod, R.,
Williams, A., and Cotterell, R. (2020). Information-
theoretic probing for linguistic structure. In Jurafsky,
D., Chai, J., Schluter, N., and Tetreault, J. R., editors,
Proceedings of the 58th Annual Meeting of the Associa-
tion for Computational Linguistics, ACL 2020, Online,
July 5-10, 2020, pages 4609–4622. Association for
Computational Linguistics.
Sowa
´
nski, M. and Janicki, A. (2020). Leyzer: A dataset for
multilingual virtual assistants. In Sojka, P., Kope
ˇ
cek,
I., Pala, K., and Horák, A., editors, Proc. Conference
on Text, Speech, and Dialogue (TSD 2020), pages 477–
486, Brno, Czechia. Springer International Publishing.
Tenney, I., Xia, P., Chen, B., Wang, A., Poliak, A., McCoy,
R. T., Kim, N., Durme, B. V., Bowman, S. R., Das,
D., and Pavlick, E. (2019). What do you learn from
context? probing for sentence structure in contextual-
ized word representations. In 7th International Con-
ference on Learning Representations, ICLR 2019, New
Orleans, LA, USA, May 6-9, 2019. OpenReview.net.
Can We Use Probing to Better Understand Fine-Tuning and Knowledge Distillation of the BERT NLU?
631