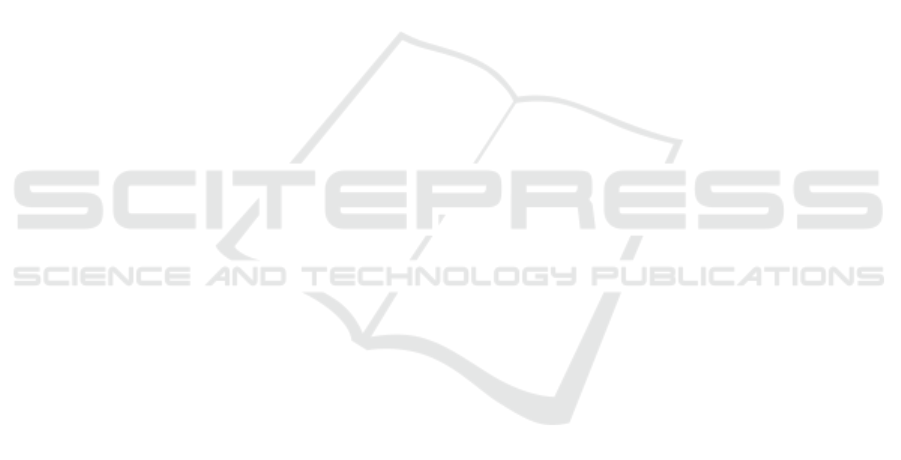
6 CONCLUSIONS
This work outlined a generic approach for a collabo-
rative validation of locally detected incidents in sim-
ple urban road networks. The network topology is
taken into account as well varying degrees of knowl-
edge about the detected incidents. Integrated into the
OTC, the approaches showed potential to improve the
accuracy and resulting success of local incident detec-
tion. As a limitation, the evaluation is based only on
assumptions about the underlying AID.
This is a first attempt at an improved detection in
OTC and next steps can be outlined: An evaluation
with real (simulated) traffic and incident situations.
Also, the AID must be carried out by the OTC as out-
lined in Section 2. Finally, the various threshold must
be optimised, e. g., by using machine learning tech-
niques. All this will multiply the test cases and possi-
ble conclusions dramatically which make a complete
test scenario unfeasible. Finally, the reinforcement
learning capabilities of the OTC should be applied,
to ensure the SASO capabilities of the system.
ACKNOWLEDGEMENTS
This research was supported by the Deutsche
Forschungsgemeinschaft, DFG, in the context of the
project “Zwischenfall-bewusstes resilientes Verkehrs-
management f
¨
ur urbane Straßennetze (InTURN)” un-
der grant TO 843/5-1. We acknowledge this support.
REFERENCES
Ahmed, S. and Cook, A. (1980). Time series models for
freeway incident detection. Transp. Eng. J of the Am.
Soc. of Civ. Eng., 106(6):731–745.
Aimsun (2021). Aimsun Next 20 User’s Manual, Aimsunm
Next 20.0.3 edition. Accessed on: October, 22, 2021.
Ester, M., Kriegel, H.-P., Sander, J., and Xu, X. (1996).
A density-based algorithm for discovering clusters in
large spatial databases with noise. In AAAI Press,
pages 226—-231.
Gokulan, B. and Srinivasan, D. (2010). Distributed geomet-
ric fuzzy multiagent urban traffic signal control. IEEE
Trans. on Int. Transportation Sys., 11(3):714–727.
Jenelius, E. and Koutsopoulos, H. (2013). Travel time esti-
mation for urban road networks using low frequency
probe vehicle data. Transp. Res. Part B: Methodolog-
ical, 53:64–81.
Lin, W. and Daganzo, C. (1997). A simple detection scheme
for delay-inducing freeway incidents. Transp. Res.
Part A: Policy and Practice, 31(2):141–155.
Mauro, V. and Taranto, C. D. (1990). Utopia. Control,
computers, communications in transportation.
M
¨
uller-Schloer, C. and Tomforde, S. (2017). Organic
Computing-Technical Systems for Survival in the Real
World. Springer.
Oliveira, L. D. and Camponogara, E. (2010). Multi-agent
model predictive control of signaling split in urban
traffic networks. Transp. Res. Part C: Emerging Tech.,
18(1):120–139.
Payne, H. and Tignor, S. (1978). Freeway incident-
detection algorithms based on decision trees with
states. Transportation Research Record.
Payne, H. J. (1975). Freeway incident detection based upon
pattern classification. In Proc. of IEEE Conf. on Deci-
sion and Control, volume 14, pages 688–692. IEEE.
Prothmann, H., Branke, J., Schmeck, H., Tomforde, S.,
Rochner, F., H
¨
ahner, J., and M
¨
uller-Schloer, C.
(2009). Organic traffic light control for urban road net-
works. Int. J. Auton. Adapt. Commun. Syst., 2(3):203–
225.
Robertson, D. and Bretherton, D. (1991). Optimizing net-
works of traffic signals in real time – the SCOOT
method. IEEE Trans. on Veh. Tech., 40(1):11–15.
Sims, A. and Dobinson, K. (1980). The Sydney coordinated
adaptive traffic (SCAT) system – Philosophy and ben-
efits. IEEE Trans. on Veh. Tech., 29(2):130–137.
Sommer, M., Tomforde, S., and H
¨
ahner, J. (2016). Forecast-
augmented route guidance in urban traffic networks
based on infrastructure observations. In Proceedings
of the International Conference on Vehicle Technol-
ogy and Intelligent Transport Systems, VEHITS 2016,
Rome, Italy, April 23-24, 2016, pages 177–186.
Stephanedes, Y. and Chassiakos, A. (1993). Freeway inci-
dent detection through filtering. Transp. Res. Part C:
Emerging Technologies, 1(3):219–233.
Thomsen, I., Zapfe, Y., and Tomforde, S. (2021a). Urban
traffic incident detection for organic traffic control: A
density-based clustering approach. In Vehits, pages
152–160.
Thomsen, I., Zapfe, Y., and Tomforde, S. (2021b). Urban
traffic incident detection for organic traffic control: A
density-based clustering approach. In Proceedings of
the 7th International Conference on Vehicle Technol-
ogy and Intelligent Transport Systems, VEHITS 2021,
Online Streaming, April 28-30, 2021, pages 152–160.
Tomforde, S., Prothmann, H., Branke, J., H
¨
ahner, J., Mnif,
M., M
¨
uller-Schloer, C., Richter, U., and Schmeck, H.
(2011). Observation and control of organic systems.
In Organic Computing—A Paradigm Shift for Com-
plex Systems, pages 325–338. Springer.
Tomforde, S. and Thomsen, I. (2022). A concept for col-
laborative incident validation in a self-organised traf-
fic management system. In Proceedings of the 8th
International Conference on Vehicle Technology and
Intelligent Transport Systems, VEHITS 2022, Online
Streaming, April 27-29, 2022, pages 316–323.
Vincent, R., Peirce, J., and Webb, P. (1990). Mova traffic
control manual. MOVA reports.
Wilson, S. W. (1995). Classifier Fitness Based on Accuracy.
Evolutionary Computation, 3(2):149–175.
Distributed Collaborative Incident Validation in a Self-Organised Traffic Control System
159