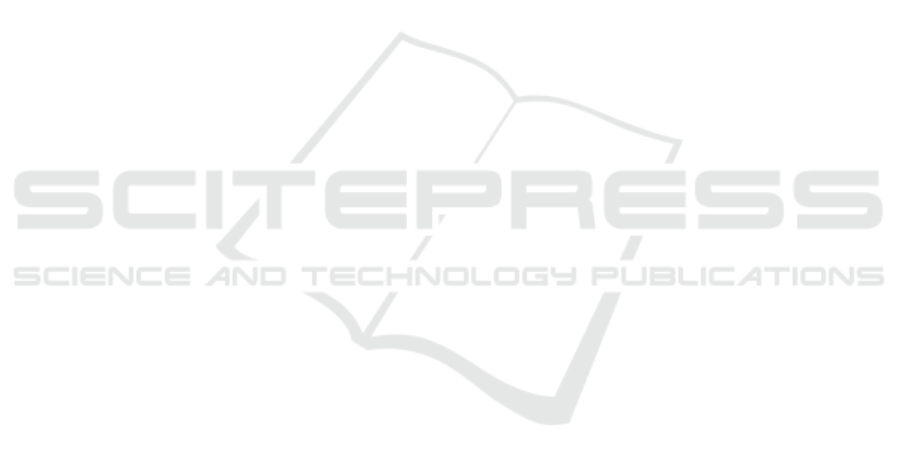
Gu, J.-C., Li, T., Liu, Q., Ling, Z.-H., Su, Z., Wei, S.,
and Zhu, X. (2020). Speaker-aware BERT for multi-
turn response selection in retrieval-based chatbots.
In Proceedings of the 29th ACM International Con-
ference on Information & Knowledge Management,
pages 2041–2044.
Haarnoja, T., Zhou, A., Abbeel, P., and Levine, S. (2018).
Soft actor-critic: Off-policy maximum entropy deep
reinforcement learning with a stochastic actor. In
International conference on machine learning, pages
1861–1870. PMLR.
Henderson, M., Casanueva, I., Mrk
ˇ
si
´
c, N., Su, P.-H., Wen,
T.-H., and Vuli
´
c, I. (2020). ConveRT: Efficient and ac-
curate conversational representations from transform-
ers. In Findings of the Association for Computational
Linguistics: EMNLP 2020, pages 2161–2174, Online.
Association for Computational Linguistics.
Jaques, N., Ghandeharioun, A., Shen, J. H., Ferguson,
C., Lapedriza,
`
A., Jones, N., Gu, S., and Picard,
R. W. (2019). Way off-policy batch deep reinforce-
ment learning of implicit human preferences in dialog.
CoRR, abs/1907.00456.
Krippendorff, K. (2011). Computing Krippendorff’s alpha-
reliability.
Lee, C.-H., Cheng, H., and Ostendorf, M. (2021). Dialogue
state tracking with a language model using schema-
driven prompting. In Proceedings of the 2021 Confer-
ence on Empirical Methods in Natural Language Pro-
cessing, pages 4937–4949, Online and Punta Cana,
Dominican Republic. Association for Computational
Linguistics.
Li, Y., Su, H., Shen, X., Li, W., Cao, Z., and Niu, S.
(2017). DailyDialog: A manually labelled multi-turn
dialogue dataset. In Proceedings of the Eighth Inter-
national Joint Conference on Natural Language Pro-
cessing (Volume 1: Long Papers), pages 986–995,
Taipei, Taiwan. Asian Federation of Natural Language
Processing.
Mehri, S. and Eskenazi, M. (2020). Unsupervised evalua-
tion of interactive dialog with DialoGPT. In Proceed-
ings of the 21th Annual Meeting of the Special Interest
Group on Discourse and Dialogue, pages 225–235.
Ni, J., Young, T., Pandelea, V., Xue, F., and Cambria, E.
(2022). Recent advances in deep learning based dia-
logue systems: A systematic survey. Artificial Intelli-
gence Review, pages 1–101.
Radford, A., Wu, J., Child, R., Luan, D., Amodei, D., and
Sutskever, I. (2019). Language models are unsuper-
vised multitask learners. OpenAI blog, 1(8), 9.
Raffel, C., Shazeer, N., Roberts, A., Lee, K., Narang, S.,
Matena, M., Zhou, Y., Li, W., Liu, P. J., et al. (2020).
Exploring the limits of transfer learning with a unified
text-to-text transformer. Journal of Machine Learning
Research, 21(140):1–67.
Rashkin, H., Smith, E. M., Li, M., and Boureau, Y.-L.
(2019). Towards empathetic open-domain conversa-
tion models: A new benchmark and dataset. In Pro-
ceedings of the 57th Annual Meeting of the Associa-
tion for Computational Linguistics, pages 5370–5381,
Florence, Italy. Association for Computational Lin-
guistics.
Reed, S., Zolna, K., Parisotto, E., Colmenarejo, S. G.,
Novikov, A., Barth-Maron, G., Gimenez, M., Sulsky,
Y., Kay, J., Springenberg, J. T., Eccles, T., Bruce, J.,
Razavi, A., Edwards, A., Heess, N., Chen, Y., Had-
sell, R., Vinyals, O., Bordbar, M., and de Freitas, N.
(2022). A generalist agent. ArXiv, abs/2205.06175.
Roller, S., Dinan, E., Goyal, N., Ju, D., Williamson, M.,
Liu, Y., Xu, J., Ott, M., Smith, E. M., Boureau, Y.-L.,
and Weston, J. (2021). Recipes for building an open-
domain chatbot. In Proceedings of the 16th Confer-
ence of the European Chapter of the Association for
Computational Linguistics: Main Volume, pages 300–
325, Online. Association for Computational Linguis-
tics.
Saleh, A., Jaques, N., Ghandeharioun, A., Shen, J., and
Picard, R. (2020). Hierarchical reinforcement learn-
ing for open-domain dialog. Proceedings of the AAAI
Conference on Artificial Intelligence, 34(05):8741–
8748.
Silver, D., Schrittwieser, J., Simonyan, K., Antonoglou, I.,
Huang, A., Guez, A., Hubert, T., Baker, L., Lai, M.,
Bolton, A., et al. (2017). Mastering the game of go
without human knowledge. nature, 550(7676):354–
359.
Unold, F. v., Wintergerst, M., Belzner, L., and Groh, G.
(2021). DYME: A dynamic metric for dialog mod-
eling learned from human conversations. In Interna-
tional Conference on Neural Information Processing,
pages 257–264. Springer.
Weston, J., Chopra, S., and Bordes, A. (2015). Memory
networks. Paper presented at 3rd International Con-
ference on Learning Representations, ICLR 2015, San
Diego, United States.
Wolf, T., Sanh, V., Chaumond, J., and Delangue, C. (2019).
TransferTransfo: A transfer learning approach for
neural network based conversational agents. CoRR,
abs/1901.08149.
Xu, C., Wu, W., and Wu, Y. (2018). Towards explain-
able and controllable open domain dialogue genera-
tion with dialogue acts. ArXiv, abs/1807.07255.
Zhang, Y., Sun, S., Galley, M., Chen, Y.-C., Brockett,
C., Gao, X., Gao, J., Liu, J., and Dolan, B. (2020).
DIALOGPT : Large-scale generative pre-training for
conversational response generation. In Proceedings of
the 58th Annual Meeting of the Association for Com-
putational Linguistics: System Demonstrations, pages
270–278, Online. Association for Computational Lin-
guistics.
Zhou, X., Dong, D., Wu, H., Zhao, S., Yu, D., Tian, H., Liu,
X., and Yan, R. (2016). Multi-view response selection
for human-computer conversation. In Proceedings of
the 2016 Conference on Empirical Methods in Natural
Language Processing, pages 372–381.
Zhou, X., Li, L., Dong, D., Liu, Y., Chen, Y., Zhao, W. X.,
Yu, D., and Wu, H. (2018). Multi-turn response se-
lection for chatbots with deep attention matching net-
work. In Proceedings of the 56th Annual Meeting of
the Association for Computational Linguistics (Vol-
ume 1: Long Papers), pages 1118–1127.
ICAART 2023 - 15th International Conference on Agents and Artificial Intelligence
640