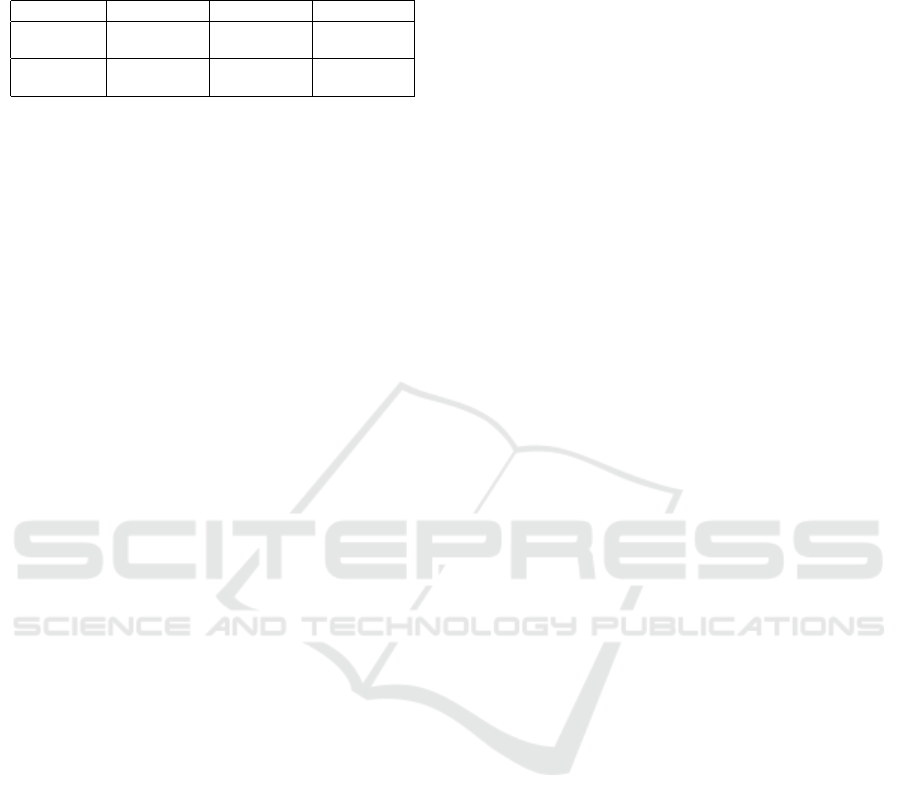
Table 1: RMSE of miss-SNF ONE and ZERO for the differ-
ent amputed (Amp.) datasets with respect to SNF applied
on the complete dataset.
Amp. 10% Amp. 20% Amp. 30%
miss-SNF
ONE 0.0020 0.0021 0.0022
miss-SNF
ZERO 0.0022 0.0025 0.0028
or more completely missing data sources. Many ap-
proaches able to integrate PSN computed from differ-
ent sources stem from the algorithm SNF (see (Ma
and Zhang, 2017; Liu and Shang, 2018; Jiang et al.,
2019; Ruan et al., 2019; Rappoport and Shamir, 2019;
Liu et al., 2021; Li et al., 2022; Wu et al., 2021)), but
only NEMO (Rappoport and Shamir, 2019) modified
the original method to take into account the presence
of partial samples, which is a largely overlooked prob-
lem in literature. Of note, NEMO requires that each
pair of patients should have at least one common data
source to be integrated. This assumption is absent in
miss-SNF. To the best of our knowledge, miss-SNF is
the first “SNF-based approch” (Gliozzo et al., 2022)
able to handle partial samples without such constraint.
We showed on a breast cancer multi-omic dataset that
miss-SNF can achieve comparable or even better per-
formance with respect to SNF considering different
percentages of partial samples present in the dataset.
Moreover, we showed that SNF is able to reconstruct
missing data. In future works, we plan to extensively
test miss-SNF on other multi-omics cancer datasets
of different sample size and on non-cancer datasets.
Moreover, we will compare miss-SNF with state-of-
the-art methods able to integrate multiple data sources
and handle the presence of completely missing sam-
ples in the dataset (Rappoport and Shamir, 2019; Xu
et al., 2021).
REFERENCES
Akhoon, N. (2021). Precision medicine: a new paradigm
in therapeutics. International Journal of Preventive
Medicine, 12.
Conesa, A. and Beck, S. (2019). Making multi-omics data
accessible to researchers. Scientific data, 6(1):1–4.
Dianatinasab, M., Mohammadianpanah, M., Daneshi,
N., Zare-Bandamiri, M., Rezaeianzadeh, A., and
Fararouei, M. (2018). Socioeconomic factors, health
behavior, and late-stage diagnosis of breast cancer:
considering the impact of delay in diagnosis. Clini-
cal breast cancer, 18(3):239–245.
Gliozzo, J., Mesiti, M., Notaro, M., Petrini, A., Patak, A.,
Puertas-Gallardo, A., Paccanaro, A., Valentini, G.,
and Casiraghi, E. (2022). Heterogeneous data integra-
tion methods for patient similarity networks. Briefings
in Bioinformatics, 23(4).
Hutter, C. and Zenklusen, J. (2018). The cancer genome
atlas: Creating lasting value beyond its data. Cell,
173(2):283–285.
Jiang, L., Xiao, Y., Ding, Y., Tang, J., and Guo, F. (2019).
Discovering cancer subtypes via an accurate fusion
strategy on multiple profile data. Frontiers in genetics,
10:20.
Kuhn, M. (2021). caret: Classification and Regression
Training. R package version 6.0-90.
Li, L., Wei, Y., Shi, G., Yang, H., Li, Z., Fang, R., Cao,
H., and Cui, Y. (2022). Multi-omics data integra-
tion for subtype identification of chinese lower-grade
gliomas: A joint similarity network fusion approach.
Computational and Structural Biotechnology Journal,
20:3482–3492.
Lightbody, G., Haberland, V., Browne, F., Taggart, L.,
Zheng, H., Parkes, E., and Blayney, J. K. (2019). Re-
view of applications of high-throughput sequencing
in personalized medicine: barriers and facilitators of
future progress in research and clinical application.
Briefings in bioinformatics, 20(5):1795–1811.
Liu, J., Liu, W., Cheng, Y., Ge, S., and Wang, X. (2021).
Similarity network fusion based on random walk
and relative entropy for cancer subtype prediction of
multigenomic data. Scientific Programming, 2021.
Liu, S. and Shang, X. (2018). Hierarchical similarity net-
work fusion for discovering cancer subtypes. In Inter-
national Symposium on Bioinformatics Research and
Applications, pages 125–136. Springer.
Ma, T. and Zhang, A. (2017). Integrate multi-omic data
using affinity network fusion (anf) for cancer patient
clustering. In 2017 IEEE International Conference on
Bioinformatics and Biomedicine (BIBM), pages 398–
403. IEEE.
Pai, S. and Bader, G. D. (2018). Patient similarity networks
for precision medicine. Journal of molecular biology,
430(18):2924–2938.
Ramos, M., Geistlinger, L., Oh, S., Schiffer, L., Azhar, R.,
Kodali, H., de Bruijn, I., Gao, J., Carey, V. J., Mor-
gan, M., and Waldron, L. (2020). Multiomic inte-
gration of public oncology databases in bioconduc-
tor. JCO Clinical Cancer Informatics, (4):958–971.
PMID: 33119407.
Rappoport, N. and Shamir, R. (2019). Nemo: cancer
subtyping by integration of partial multi-omic data.
Bioinformatics, 35(18):3348–3356.
Ruan, P., Wang, Y., Shen, R., and Wang, S. (2019). Us-
ing association signal annotations to boost similarity
network fusion. Bioinformatics, 35(19):3718–3726.
Tomczak, K., Czerwi
´
nska, P., and Wiznerowicz, M. (2015).
Review the cancer genome atlas (tcga): an immea-
surable source of knowledge. Contemporary Oncol-
ogy/Wsp
´
ołczesna Onkologia, 2015(1):68–77.
Wang, B., Mezlini, A., Demir, F., Fiume, M., Tu, Z.,
Brudno, M., Haibe-Kains, B., and Goldenberg, A.
(2021). SNFtool: Similarity Network Fusion. R pack-
age version 2.3.1.
Wang, B., Mezlini, A. M., Demir, F., Fiume, M., Tu, Z.,
Brudno, M., Haibe-Kains, B., and Goldenberg, A.
(2014). Similarity network fusion for aggregating data
Patient Similarity Networks Integration for Partial Multimodal Datasets
233