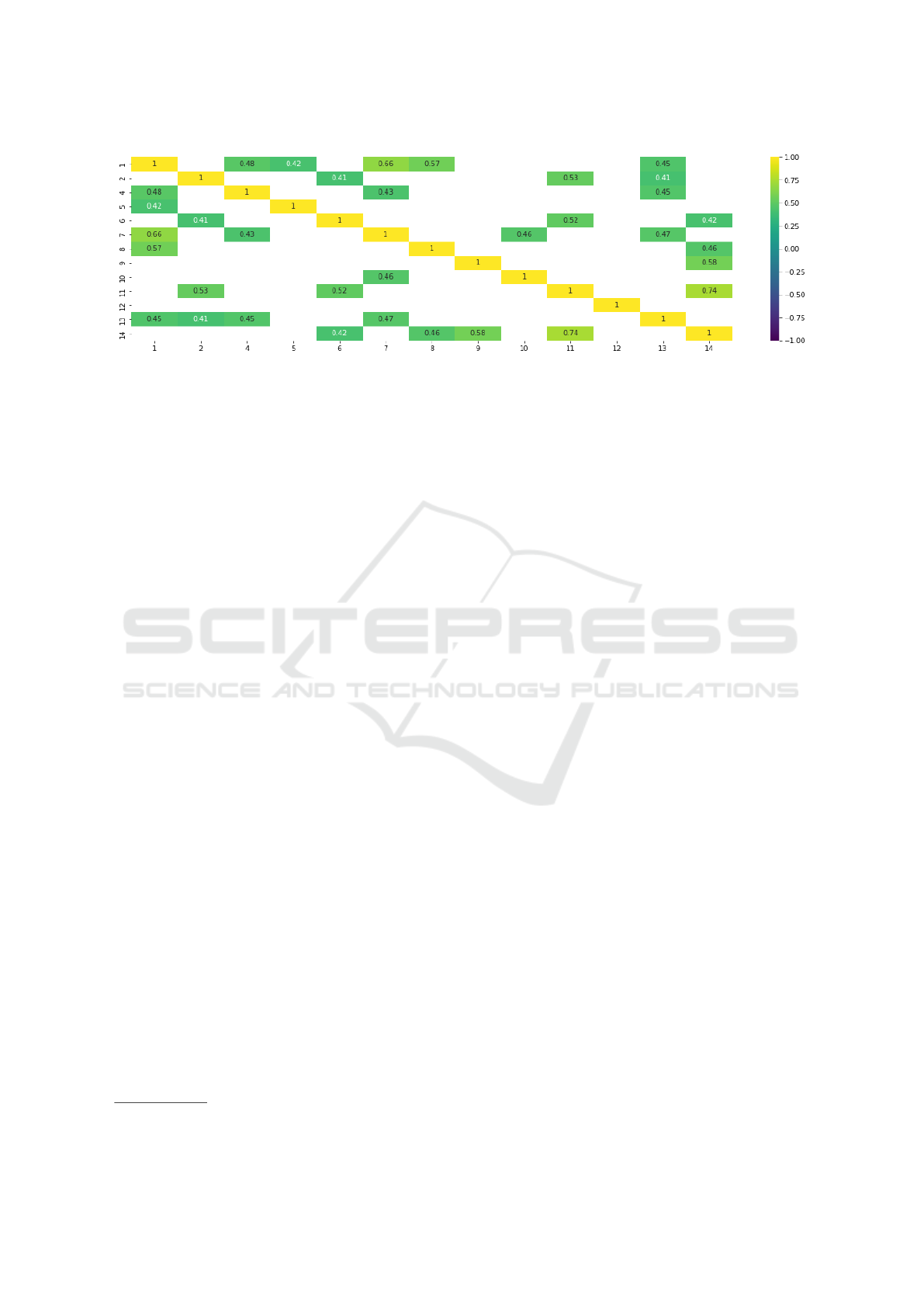
Figure 7: Filtered p-value< 0.05 Spearman correlation.
5 CONCLUSIONS
In this paper, we presented a smart glass applica-
tion that assists industrial employees understanding
human-object interactions. To avoid the challenge re-
lated to 3D object annotation, we proposed a system
that uses a 2D object detector to find and identify the
objects in the scene and common features available
on AR devices such as plane detector, virtual object
anchoring, and hand tracking to predict how a human
would interact with the objects. For qualitative evalu-
ation purpose, we set up a test campaign for the appli-
cation, in which the 25 volunteers tested the applica-
tion and responded to a survey on the app’s function-
ality and usability. The results suggest that approach
presented in this work can be useful to develop appli-
cations helpful in manufacturing environments.
ACKNOWLEDGEMENTS
This research has been supported by Next Vision
9
s.r.l., by the project MISE - PON I&C 2014-2020
- Progetto ENIGMA - Prog n. F/190050/02/X44 –
CUP: B61B19000520008 and by Research Program
Pia.ce.ri. 2020/2022 Linea 2 - University of Catania.
REFERENCES
Chao, Y.-W., Liu, Y., Liu, X., Zeng, H., and Deng, J. (2017).
Learning to detect human-object interactions.
Colombo, S., Lim, Y., and Casalegno, F. (2019). Deep vi-
sion shield: Assessing the use of hmd and wearable
sensors in a smart safety device. PETRA ’19, page
402–410, New York, NY, USA. Association for Com-
puting Machinery.
9
Next Vision: https://www.nextvisionlab.it/
Cucchiara, R. and Del Bimbo, A. (2014). Visions for aug-
mented cultural heritage experience. IEEE Multime-
dia, 21.
Damen, D., Leelasawassuk, T., Haines, O., Calway, A., and
Mayol-Cuevas, W. (2014). You-do, i-learn: Discover-
ing task relevant objects and their modes of interaction
from multi-user egocentric video. In Proceedings of
the British Machine Vision Conference. BMVA Press.
Farinella, G., Signorello, G., Battiato, S., Furnari, A., Ra-
gusa, F., Leonardi, R., Ragusa, E., Scuderi, E., Lopes,
A., Santo, L., and Samarotto, M. (2019). VEDI: Vision
Exploitation for Data Interpretation, pages 753–763.
Geiger, A., Lenz, P., and Urtasun, R. (2012). Are we ready
for autonomous driving? the kitti vision benchmark
suite. In Conference on Computer Vision and Pattern
Recognition (CVPR).
Gkioxari, G., Girshick, R., Doll
´
ar, P., and He, K. (2017).
Detecting and recognizing human-object interactions.
Grauman, K., Westbury, A., Byrne, E., Chavis, Z., Furnari,
A., Girdhar, R., Hamburger, J., Jiang, H., Liu, M.,
Liu, X., Martin, M., Nagarajan, T., Radosavovic, I.,
Ramakrishnan, S. K., Ryan, F., Sharma, J., Wray, M.,
Xu, M., Xu, E. Z., Zhao, C., Bansal, S., Batra, D., Car-
tillier, V., Crane, S., Do, T., Doulaty, M., Erapalli, A.,
Feichtenhofer, C., Fragomeni, A., Fu, Q., Fuegen, C.,
Gebreselasie, A., Gonzalez, C., Hillis, J., Huang, X.,
Huang, Y., Jia, W., Khoo, W., Kolar, J., Kottur, S., Ku-
mar, A., Landini, F., Li, C., Li, Y., Li, Z., Mangalam,
K., Modhugu, R., Munro, J., Murrell, T., Nishiyasu,
T., Price, W., Puentes, P. R., Ramazanova, M., Sari,
L., Somasundaram, K., Southerland, A., Sugano, Y.,
Tao, R., Vo, M., Wang, Y., Wu, X., Yagi, T., Zhu,
Y., Arbelaez, P., Crandall, D., Damen, D., Farinella,
G. M., Ghanem, B., Ithapu, V. K., Jawahar, C. V., Joo,
H., Kitani, K., Li, H., Newcombe, R., Oliva, A., Park,
H. S., Rehg, J. M., Sato, Y., Shi, J., Shou, M. Z., Tor-
ralba, A., Torresani, L., Yan, M., and Malik, J. (2022).
Ego4d: Around the World in 3,000 Hours of Egocen-
tric Video. In IEEE/CVF Computer Vision and Pattern
Recognition (CVPR).
Gupta, S. and Malik, J. (2015). Visual semantic role label-
ing.
Leonardi, R., Ragusa, F., Furnari, A., and Farinella, G. M.
(2022). Egocentric human-object interaction detection
exploiting synthetic data.
VISAPP 2023 - 18th International Conference on Computer Vision Theory and Applications
670