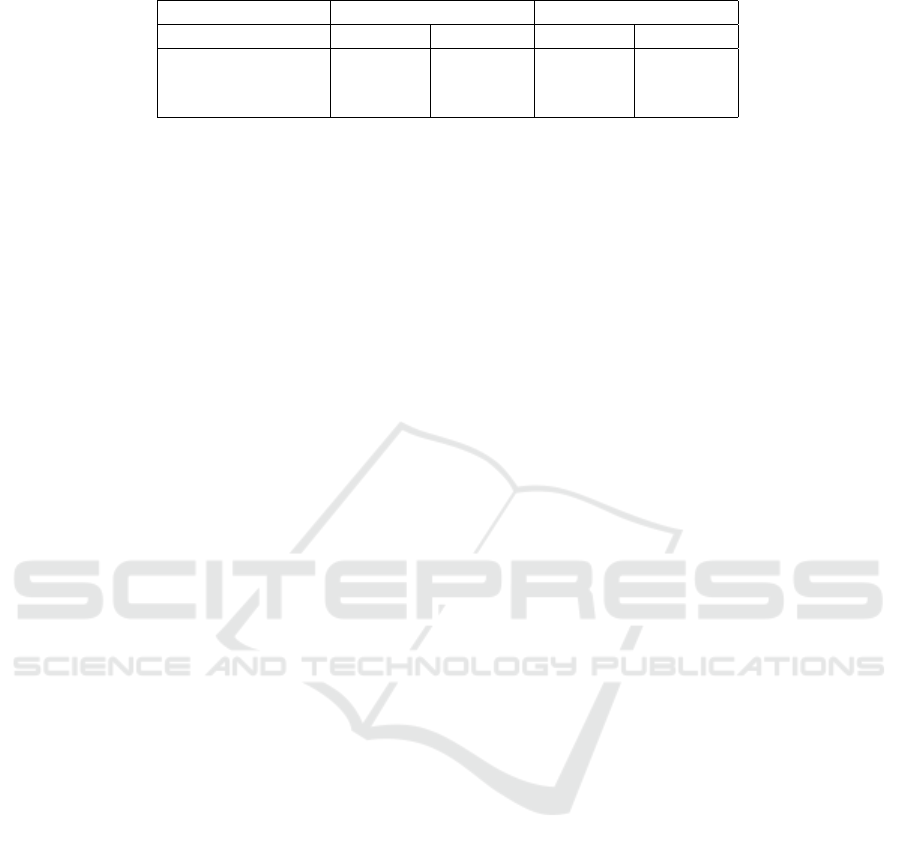
Table 3: Final model scores for the KBP task on the DWIE dataset.
Cold-start Warm-start
Model F1-Micro F1-Macro F1-Micro F1-Macro
ELROND+FUSION 83.5 76.3 82.0 72.1
ELROND 83.4 76.1 81.4 72.1
DWIE 82.8 75.6 80.3 69.9
guage understanding. In Proceedings of NAACL-HLT,
pages 4171–4186.
Khosla, P., Teterwak, P., Wang, C., Sarna, A., Tian, Y.,
Isola, P., Maschinot, A., Liu, C., and Krishnan, D.
(2020). Supervised contrastive learning. Advances
in Neural Information Processing Systems, 33:18661–
18673.
Kuhn, H. W. (1955). The hungarian method for the assign-
ment problem. Naval research logistics quarterly, 2(1-
2):83–97.
Lin, X., Li, H., Xin, H., Li, Z., and Chen, L. (2020). Kb-
pearl: a knowledge base population system supported
by joint entity and relation linking. Proceedings of the
VLDB Endowment, 13(7):1035–1049.
Liu, Y., Ott, M., Goyal, N., Du, J., Joshi, M., Chen, D.,
Levy, O., Lewis, M., Zettlemoyer, L., and Stoyanov, V.
(2019). Roberta: A robustly optimized BERT pretraining
approach. CoRR.
Luo, C., Zhan, J., Xue, X., Wang, L., Ren, R., and Yang, Q.
(2018). Cosine normalization: Using cosine similarity
instead of dot product in neural networks. In Interna-
tional Conference on Artificial Neural Networks, pages
382–391. Springer.
Mesquita, F., Cannaviccio, M., Schmidek, J., Mirza, P.,
and Barbosa, D. (2019). Knowledgenet: A benchmark
dataset for knowledge base population. In Proceedings
of the 2019 Conference on Empirical Methods in Natu-
ral Language Processing and the 9th International Joint
Conference on Natural Language Processing (EMNLP-
IJCNLP), pages 749–758.
Min, B., Freedman, M., Bock, R., and Weischedel, R.
(2018). When ace met kbp: End-to-end evaluation of
knowledge base population with component-level anno-
tation. In Proceedings of the Eleventh International Con-
ference on Language Resources and Evaluation (LREC
2018).
Shue, L.-Y., Chen, C.-W., and Shiue, W. (2009). The devel-
opment of an ontology-based expert system for corpo-
rate financial rating. Expert Systems with Applications,
36(2):2130–2142.
Soares, L. B., Fitzgerald, N., Ling, J., and Kwiatkowski, T.
(2019). Matching the blanks: Distributional similarity
for relation learning. In Proceedings of the 57th Annual
Meeting of the Association for Computational Linguis-
tics, pages 2895–2905.
Wu, L., Petroni, F., Josifoski, M., Riedel, S., and Zettle-
moyer, L. (2020). Scalable zero-shot entity linking with
dense entity retrieval. In Proceedings of the 2020 Con-
ference on Empirical Methods in Natural Language Pro-
cessing (EMNLP), pages 6397–6407.
Yao, Y., Ye, D., Li, P., Han, X., Lin, Y., Liu, Z., Liu, Z.,
Huang, L., Zhou, J., and Sun, M. (2019). Docred: A
large-scale document-level relation extraction dataset. In
ACL (1).
Zaporojets, K., Deleu, J., Develder, C., and Demeester, T.
(2021). Dwie: An entity-centric dataset for multi-task
document-level information extraction. Information Pro-
cessing & Management, 58(4):102563.
Zhang, W., Hua, W., and Stratos, K. (2021). Entqa: Entity
linking as question answering. In International Confer-
ence on Learning Representations.
Zhou, W., Huang, K., Ma, T., and Huang, J. (2021).
Document-level relation extraction with adaptive thresh-
olding and localized context pooling. In Proceedings
of the AAAI conference on artificial intelligence, vol-
ume 35, pages 14612–14620.
Evaluating and Improving End-to-End Systems for Knowledge Base Population
649