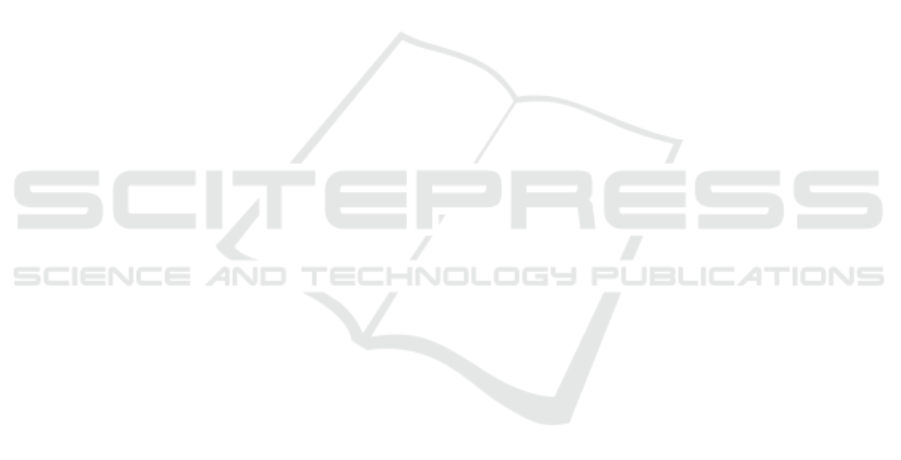
national AAAI Conference on Web and Social Media,
volume 14, pages 95–106.
Castelletti, A. and Soncini-Sessa, R. (2007). Bayesian net-
works and participatory modelling in water resource
management. Environmental Modelling & Software,
22(8):1075–1088.
Chen, N., Ribeiro, B., and Chen, A. (2016). Financial credit
risk assessment: A recent review. Artif. Intell. Rev.,
45(1):1–23.
Chouldechova, A. (2017). Fair prediction with disparate im-
pact: A study of bias in recidivism prediction instru-
ments. Big Data, 5(2):153–163. PMID: 28632438.
Christian, B. (2020). The alignment problem: Machine
learning and human values. WW Norton & Company.
Christin, A. (2017). Algorithms in practice: Comparing
web journalism and criminal justice. Big Data & So-
ciety, 4(2):2053951717718855.
Chu, E., Roy, D., and Andreas, J. (2020). Are visual ex-
planations useful? a case study in model-in-the-loop
prediction. arXiv preprint arXiv:2007.12248.
Cooper, G. F. (1990). The computational complexity
of probabilistic inference using bayesian belief net-
works. Artificial intelligence, 42(2-3):393–405.
Culverhouse, P. F., Williams, R., Reguera, B., Herry, V.,
and Gonz
´
alez-Gil, S. (2003). Do experts make mis-
takes? a comparison of human and machine indenti-
fication of dinoflagellates. Marine ecology progress
series, 247:17–25.
Cummings, M. (2004). Automation bias in intelligent time
critical decision support systems. In AIAA 1st intelli-
gent systems technical conference, page 6313.
Darwiche, A. (2003). A differential approach to inference
in bayesian networks. Journal of the ACM (JACM),
50(3):280–305.
Darwiche, A. (2009). Modeling and reasoning with
Bayesian networks. Cambridge university press.
Dechter, R., Meiri, I., and Pearl, J. (1991). Temporal con-
straint networks. Artificial intelligence, 49(1-3):61–
95.
Dietvorst, B. J., Simmons, J. P., and Massey, C. (2015).
Algorithm aversion: people erroneously avoid algo-
rithms after seeing them err. Journal of Experimental
Psychology: General, 144(1):114.
Doshi-Velez, F. and Kim, B. (2017). Towards a rigorous sci-
ence of interpretable machine learning. arXiv preprint
arXiv:1702.08608.
Dressel, J. and Farid, H. (2018). The accuracy, fairness,
and limits of predicting recidivism. Science advances,
4(1):eaao5580.
Finlay, S. (2011). Multiple classifier architectures and their
application to credit risk assessment. European Jour-
nal of Operational Research, 210(2):368–378.
Floridi, L., Cowls, J., Beltrametti, M., Chatila, R.,
Chazerand, P., Dignum, V., Luetge, C., Madelin, R.,
Pagallo, U., Rossi, F., et al. (2018). Ai4people—an
ethical framework for a good ai society: Opportuni-
ties, risks, principles, and recommendations. Minds
and Machines, 28(4):689–707.
Friis-Hansen, A. (2000). Bayesian networks as a decision
support tool in marine applications.
Gabriel, I. (2020). Artificial intelligence, values, and align-
ment. Minds and machines, 30(3):411–437.
Galanos, V. (2019). Exploring expanding expertise: arti-
ficial intelligence as an existential threat and the role
of prestigious commentators, 2014–2018. Technology
Analysis & Strategic Management, 31(4):421–432.
Geiger, D., Verma, T., and Pearl, J. (1990). d-separation:
From theorems to algorithms. In Machine Intelligence
and Pattern Recognition, volume 10, pages 139–148.
Elsevier.
Gillespie, T. (2016). #Trendingistrending: When Algo-
rithms Become Culture. Routledge.
Goh, Y., Cai, X., Theseira, W., Ko, G., and Khor, K.
(2020). Evaluating human versus machine learning
performance in classifying research abstracts. Scien-
tometrics, 125.
Grove, W. M., Zald, D. H., Lebow, B. S., Snitz, B. E., and
Nelson, C. (2000). Clinical versus mechanical pre-
diction: a meta-analysis. Psychological assessment,
12(1):19.
Guidotti, R., Monreale, A., Ruggieri, S., Turini, F., Gian-
notti, F., and Pedreschi, D. (2018). A survey of meth-
ods for explaining black box models. ACM computing
surveys (CSUR), 51(5):1–42.
Gunning, D. and Aha, D. (2019). Darpa’s explainable
artificial intelligence (xai) program. AI magazine,
40(2):44–58.
Gunning, D., Stefik, M., Choi, J., Miller, T., Stumpf, S.,
and Yang, G.-Z. (2019). Xai—explainable artificial
intelligence. Science Robotics, 4(37):eaay7120.
Guo, W. (2020). Explainable artificial intelligence for 6g:
Improving trust between human and machine. IEEE
Communications Magazine, 58(6):39–45.
Gutmann, B., Thon, I., Kimmig, A., Bruynooghe, M., and
De Raedt, L. (2011). The magic of logical inference
in probabilistic programming. TPLP, 11:663–680.
Hilder, S., Harvey, R. W., and Theobald, B.-J. (2009). Com-
parison of human and machine-based lip-reading. In
AVSP, pages 86–89.
Hood, C. and Heald, D. (2006). Transparency in historical
perspective. Number 135. Oxford University Press.
Hu, Z., Ma, X., Liu, Z., Hovy, E. H., and Xing, E. P.
(2016). Harnessing deep neural networks with logic
rules. CoRR, abs/1603.06318.
Jobin, A., Ienca, M., and Vayena, E. (2019). The global
landscape of ai ethics guidelines. Nature Machine In-
telligence, 1(9):389–399.
Kalet, A. M., Gennari, J. H., Ford, E. C., and Phillips, M. H.
(2015). Bayesian network models for error detection
in radiotherapy plans. Physics in Medicine & Biology,
60(7):2735.
Kaur, H., Nori, H., Jenkins, S., Caruana, R., Wallach, H.,
and Wortman Vaughan, J. (2020). Interpreting inter-
pretability: understanding data scientists’ use of inter-
pretability tools for machine learning. In Proceedings
of the 2020 CHI conference on human factors in com-
puting systems, pages 1–14.
Kleinberg, J., Lakkaraju, H., Leskovec, J., Ludwig, J., and
Mullainathan, S. (2017). Human Decisions and Ma-
Model Transparency: Why Do We Care?
655