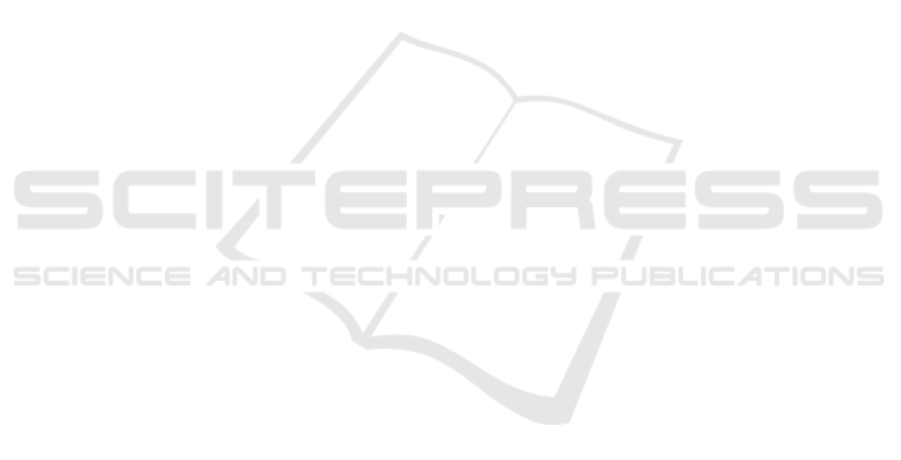
Khraisat, A., Gondal, I., Vamplew, P., and Kamruzzaman,
J. (2019). Survey of intrusion detection systems:
techniques, datasets and challenges. Cybersecurity,
2(1):1–22.
Lee, W. and Stolfo, S. (1998). Data mining approaches for
intrusion detection.
Lee, W. and Xiang, D. (2000). Information-theoretic mea-
sures for anomaly detection. In Proceedings 2001
IEEE Symposium on Security and Privacy. S&P 2001,
pages 130–143. IEEE.
Liu, M., Xue, Z., Xu, X., Zhong, C., and Chen, J. (2018).
Host-based intrusion detection system with system
calls: Review and future trends. ACM Computing Sur-
veys (CSUR), 51(5):1–36.
Luo, Z., Hsieh, J.-T., Balachandar, N., Yeung, S., Pusiol,
G., Luxenberg, J., Li, G., Li, L.-J., Downing, N. L.,
Milstein, A., et al. (2018). Computer vision-based
descriptive analytics of seniors’ daily activities for
long-term health monitoring. Machine Learning for
Healthcare (MLHC), 2:1.
Maseer, Z. K., Yusof, R., Bahaman, N., Mostafa, S. A., and
Foozy, C. F. M. (2021). Benchmarking of machine
learning for anomaly based intrusion detection sys-
tems in the cicids2017 dataset. IEEE access, 9:22351–
22370.
Mirsky, Y., Doitshman, T., Elovici, Y., and Shabtai, A.
(2018). Kitsune: an ensemble of autoencoders for
online network intrusion detection. arXiv preprint
arXiv:1802.09089.
Nunes, M. (2018). Dynamic Malware Analysis kernel and
user-level calls.
Nunes, M., Burnap, P., Rana, O., Reinecke, P., and Lloyd,
K. (2019). Getting to the root of the problem: A de-
tailed comparison of kernel and user level data for dy-
namic malware analysis. Journal of Information Se-
curity and Applications, 48:102365.
Rigoutsos, I. and Floratos, A. (1998). Combinatorial pattern
discovery in biological sequences: The teiresias algo-
rithm. Bioinformatics (Oxford, England), 14(1):55–
67.
Roesch, M. et al. (1999). Snort: Lightweight intrusion de-
tection for networks. In Lisa, volume 99, pages 229–
238.
Roshan, S., Miche, Y., Akusok, A., and Lendasse, A.
(2018). Adaptive and online network intrusion detec-
tion system using clustering and extreme learning ma-
chines. Journal of the Franklin Institute, 355(4):1752–
1779.
Schwartz, D., Stoecklin, S., and Yilmaz, E. (2002). Case-
based agents for packet-level intrusion detection in ad
hoc networks. In Proc, of the 17th Int. Symp. on Com-
puter and Information Sciences, volume 7, page 59.
Song, M., G
¨
unther, C. W., and Van der Aalst, W. M. (2008).
Trace clustering in process mining. In International
conference on business process management, pages
109–120. Springer.
Tavallaee, M., Bagheri, E., Lu, W., and Ghorbani, A. A.
(2009). A detailed analysis of the kdd cup 99 data
set. In 2009 IEEE symposium on computational intel-
ligence for security and defense applications, pages
1–6. Ieee.
Van Der Aalst, W. (2011). Process mining: discovery,
conformance and enhancement of business processes,
volume 2. Springer.
van Der Aalst, W. M., Ter Hofstede, A. H., Kiepuszewski,
B., and Barros, A. P. (2003). Workflow patterns. Dis-
tributed and parallel databases, 14(1):5–51.
van Zelst, S. J., Bolt, A., Hassani, M., van Dongen, B. F.,
and van der Aalst, W. M. (2019). Online conformance
checking: relating event streams to process models us-
ing prefix-alignments. International Journal of Data
Science and Analytics, 8(3):269–284.
Vijayasarathy, R., Raghavan, S. V., and Ravindran, B.
(2011). A system approach to network modeling for
ddos detection using a naive bayesian classifier. In
2011 Third International Conference on Communica-
tion Systems and Networks (COMSNETS 2011), pages
1–10. IEEE.
Wang, B., Su, Y., Zhang, M., and Nie, J. (2020). A deep
hierarchical network for packet-level malicious traffic
detection. IEEE Access, 8:201728–201740.
Wespi, A., Dacier, M., and Debar, H. (2000). Intrusion de-
tection using variable-length audit trail patterns. In
International Workshop on Recent Advances in Intru-
sion Detection, pages 110–129. Springer.
Zavrak, S. and
˙
Iskefiyeli, M. (2020). Anomaly-based intru-
sion detection from network flow features using vari-
ational autoencoder. IEEE Access, 8:108346–108358.
Zhong, Y., Goulermas, J. Y., and Lisitsa, A. (2022). Pro-
cess mining algorithm for online intrusion detection
system. arXiv preprint arXiv:2205.12064.
Zhong, Y. and Lisitsa, A. (2022). Can process mining help
in anomaly-based intrusion detection? arXiv preprint
arXiv:2206.10379.
ICISSP 2023 - 9th International Conference on Information Systems Security and Privacy
582