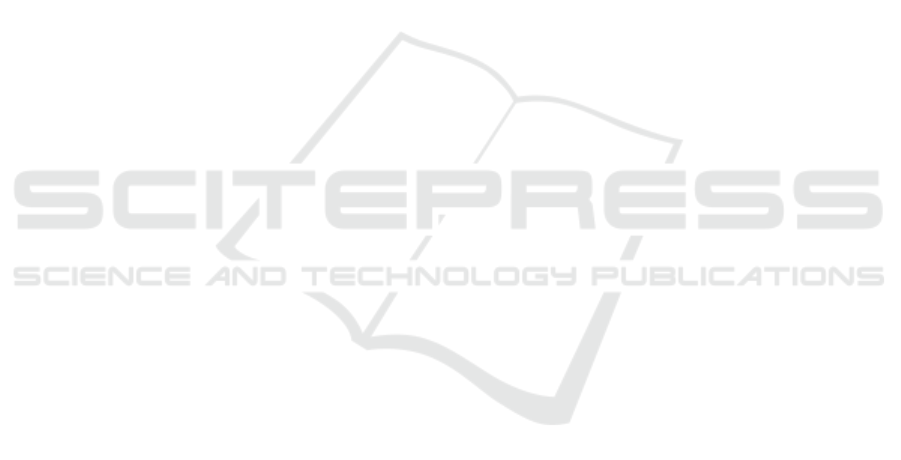
Lin, T.-Y., Goyal, P., Girshick, R., He, K., and Doll
´
ar, P.
(2017). Focal loss for dense object detection. In
Proceedings of the IEEE international conference on
computer vision, pages 2980–2988.
Liu, W., Anguelov, D., Erhan, D., Szegedy, C., Reed, S.,
Fu, C.-Y., and Berg, A. C. (2016). Ssd: Single shot
multibox detector. In European conference on com-
puter vision, pages 21–37. Springer.
Liu, W., Ren, G., Yu, R., Guo, S., Zhu, J., and Zhang,
L. (2022). Image-adaptive yolo for object detection
in adverse weather conditions. In Proceedings of
the AAAI Conference on Artificial Intelligence, vol-
ume 36, pages 1792–1800.
Loh, Y. P. and Chan, C. S. (2019). Getting to know low-light
images with the exclusively dark dataset. Computer
Vision and Image Understanding, 178:30–42.
Mishra, B., Garg, D., Narang, P., and Mishra, V. (2020).
Drone-surveillance for search and rescue in natural
disaster. Computer Communications, 156:1–10.
Mueller, M., Smith, N., and Ghanem, B. (2016). A bench-
mark and simulator for uav tracking. In European con-
ference on computer vision, pages 445–461. Springer.
Nada, H., Sindagi, V. A., Zhang, H., and Patel, V. M.
(2018). Pushing the limits of unconstrained face de-
tection: a challenge dataset and baseline results. In
2018 IEEE 9th International Conference on Biomet-
rics Theory, Applications and Systems (BTAS), pages
1–10. IEEE.
Oh, S., Hoogs, A., Perera, A., Cuntoor, N., Chen, C.-C.,
Lee, J. T., Mukherjee, S., Aggarwal, J., Lee, H., Davis,
L., et al. (2011). A large-scale benchmark dataset
for event recognition in surveillance video. In CVPR
2011, pages 3153–3160. IEEE.
Purkait, P., Zhao, C., and Zach, C. (2017). Spp-net: Deep
absolute pose regression with synthetic views. arXiv
preprint arXiv:1712.03452.
Qin, X., Wang, Z., Bai, Y., Xie, X., and Jia, H. (2020). Ffa-
net: Feature fusion attention network for single im-
age dehazing. In Proceedings of the AAAI Conference
on Artificial Intelligence, volume 34, pages 11908–
11915.
Ren, S., He, K., Girshick, R., and Sun, J. (2015). Faster
r-cnn: Towards real-time object detection with region
proposal networks. Advances in neural information
processing systems, 28.
Sakaridis, C., Dai, D., and Van Gool, L. (2018). Semantic
foggy scene understanding with synthetic data. In-
ternational Journal of Computer Vision, 126(9):973–
992.
Shan, Y., Lu, W. F., and Chew, C. M. (2019). Pixel
and feature level based domain adaptation for object
detection in autonomous driving. Neurocomputing,
367:31–38.
Sindagi, V. A., Oza, P., Yasarla, R., and Patel, V. M. (2020).
Prior-based domain adaptive object detection for hazy
and rainy conditions. In European Conference on
Computer Vision, pages 763–780. Springer.
Singh, A., Bhave, A., and Prasad, D. K. (2020). Single
image dehazing for a variety of haze scenarios using
back projected pyramid network. In European Confer-
ence on Computer Vision, pages 166–181. Springer.
Szegedy, C., Vanhoucke, V., Ioffe, S., Shlens, J., and Wojna,
Z. (2015). Rethinking the inception architecture for
computer vision. CoRR, abs/1512.00567.
Timofte, R., Agustsson, E., Van Gool, L., Yang, M.-H., and
Zhang, L. (2017). Ntire 2017 challenge on single im-
age super-resolution: Methods and results. In Pro-
ceedings of the IEEE conference on computer vision
and pattern recognition workshops, pages 114–125.
Uijlings, J. R., Van De Sande, K. E., Gevers, T., and
Smeulders, A. W. (2013). Selective search for object
recognition. International journal of computer vision,
104(2):154–171.
Wang, L., Wang, L., Lu, H., Zhang, P., and Ruan, X. (2016).
Saliency detection with recurrent fully convolutional
networks. In European conference on computer vi-
sion, pages 825–841. Springer.
Wei, C., Wang, W., Yang, W., and Liu, J. (2018). Deep
retinex decomposition for low-light enhancement.
arXiv preprint arXiv:1808.04560.
Wu, Y., Kirillov, A., Massa, F., Lo, W.-Y., and Gir-
shick, R. (2019). Detectron2. https://github.com/
facebookresearch/detectron2.
Yang, W., Tan, R. T., Feng, J., Liu, J., Guo, Z., and Yan, S.
(2017). Deep joint rain detection and removal from
a single image. In Proceedings of the IEEE con-
ference on computer vision and pattern recognition,
pages 1357–1366.
Yao, B., Yang, X., and Zhu, S.-C. (2007). Introduc-
tion to a large-scale general purpose ground truth
database: methodology, annotation tool and bench-
marks. In International Workshop on Energy Min-
imization Methods in Computer Vision and Pattern
Recognition, pages 169–183. Springer.
Zhang, H., Sindagi, V., and Patel, V. M. (2019). Image
de-raining using a conditional generative adversarial
network. IEEE transactions on circuits and systems
for video technology, 30(11):3943–3956.
Zhang, N., Zhang, L., and Cheng, Z. (2017a). Towards sim-
ulating foggy and hazy images and evaluating their
authenticity. In International Conference on Neural
Information Processing, pages 405–415. Springer.
Zhang, S., Tuo, H., Hu, J., and Jing, Z. (2021a). Domain
adaptive yolo for one-stage cross-domain detection. In
Asian Conference on Machine Learning, pages 785–
797. PMLR.
Zhang, Y., Ding, L., and Sharma, G. (2017b). Hazerd: an
outdoor scene dataset and benchmark for single image
dehazing. In 2017 IEEE international conference on
image processing (ICIP), pages 3205–3209. IEEE.
Zhang, Z., Jiang, Y., Jiang, J., Wang, X., Luo, P., and Gu,
J. (2021b). Star: A structure-aware lightweight trans-
former for real-time image enhancement. In Proceed-
ings of the IEEE/CVF International Conference on
Computer Vision, pages 4106–4115.
Zheng, S. and Gupta, G. (2022). Semantic-guided zero-shot
learning for low-light image/video enhancement. In
Proceedings of the IEEE/CVF Winter Conference on
Applications of Computer Vision, pages 581–590.
ICAART 2023 - 15th International Conference on Agents and Artificial Intelligence
664