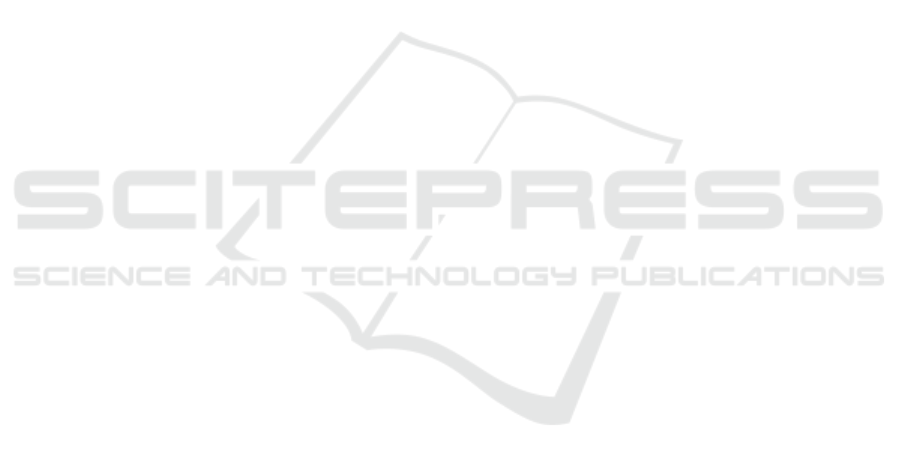
Lake, B. M., Salakhutdinov, R., and Tenenbaum, J. B.
(2015). Human-level concept learning through proba-
bilistic program induction. Science, 350(6266):1332–
1338. Publisher: American Association for the Ad-
vancement of Science.
Li, M., Zhao, K., Peng, C., Hobson, P., Jennings, T., and
Lovell, B. C. (2021). Deep Adaptive Few Example
Learning for Microscopy Image Cell Counting. In
2021 Digital Image Computing: Techniques and Ap-
plications (DICTA), pages 1–7.
Lin, T.-Y., Maire, M., Belongie, S. J., Bourdev, L. D., Gir-
shick, R. B., Hays, J., Perona, P., Ramanan, D., Doll
´
ar,
P., and Zitnick, C. L. (2014). Microsoft COCO: Com-
mon Objects in Context. In Computer Vision - ECCV
2014, volume 8693, pages 740–755, Cham. Springer
International Publishing.
Medela, A., Picon, A., Saratxaga, C. L., Belar, O., Cabez
´
on,
V., Cicchi, R., Bilbao, R., and Glover, B. (2019). Few
Shot Learning in Histopathological Images:Reducing
the Need of Labeled Data on Biological Datasets. In
2019 IEEE 16th International Symposium on Biomed-
ical Imaging (ISBI 2019), pages 1860–1864. ISSN:
1945-8452.
Nichol, A., Achiam, J., and Schulman, J. (2018). Reptile:
A Scalable Meta-Learning Algorithm.
Rajeswaran, A., Finn, C., Kakade, S. M., and Levine, S.
(2019). Meta-learning with implicit gradients. In Pro-
ceedings of the 33rd International Conference on Neu-
ral Information Processing Systems, pages 113–124,
Red Hook, NY, USA. Curran Associates Inc.
Rodr
´
ıguez, P., Laradji, I., Drouin, A., and Lacoste, A.
(2020). Embedding Propagation: Smoother Manifold
for Few-Shot Classification. In Vedaldi, A., Bischof,
H., Brox, T., and Frahm, J.-M., editors, Computer Vi-
sion – ECCV 2020, Lecture Notes in Computer Sci-
ence, pages 121–138, Cham. Springer International
Publishing.
Russakovsky, O., Deng, J., Su, H., Krause, J., Satheesh, S.,
Ma, S., Huang, Z., Karpathy, A., Khosla, A., Bern-
stein, M., Berg, A. C., and Fei-Fei, L. (2015). Ima-
geNet Large Scale Visual Recognition Challenge. Int
J Comput Vis, 115(3):211–252.
Santoro, A., Bartunov, S., Botvinick, M., Wierstra, D., and
Lillicrap, T. (2016). Meta-Learning with Memory-
Augmented Neural Networks. In Proceedings of The
33rd International Conference on Machine Learning,
pages 1842–1850. PMLR. ISSN: 1938-7228.
Scotland, R. W. and Wortley, A. H. (2003). How Many
Species of Seed Plants Are There? Taxon, 52(1):101–
104.
Sevillano, V., Holt, K., and Aznarte, J. L. (2020). Precise
automatic classification of 46 different pollen types
with convolutional neural networks. PLOS ONE,
15(6):e0229751.
Shaikh, N. N., Wasag, K., and Nie, Y. (2022). Artifact
Identification in Digital Histopathology Images Using
Few-Shot Learning. In 2022 IEEE 19th International
Symposium on Biomedical Imaging (ISBI), pages 1–4.
ISSN: 1945-8452.
Simonyan, K. and Zisserman, A. (2015). Very Deep Con-
volutional Networks for Large-Scale Image Recogni-
tion. In International Conference on Learning Repre-
sentations.
Snell, J., Swersky, K., and Zemel, R. (2017). Prototypi-
cal Networks for Few-shot Learning. In Advances in
Neural Information Processing Systems, volume 30.
Curran Associates, Inc.
Stillman, E. C. and Flenley, J. R. (1996). The needs and
prospects for automation in palynology. Quaternary
Science Reviews, 15(1):1–5.
Sung, F., Yang, Y., Zhang, L., Xiang, T., Torr, P. H., and
Hospedales, T. M. (2018). Learning to Compare:
Relation Network for Few-Shot Learning. In 2018
IEEE/CVF Conference on Computer Vision and Pat-
tern Recognition, pages 1199–1208. ISSN: 2575-
7075.
Tan, M. and Le, Q. (2021). EfficientNetV2: Smaller Mod-
els and Faster Training. In Proceedings of the 38th In-
ternational Conference on Machine Learning, pages
10096–10106. PMLR. ISSN: 2640-3498.
Tsiknakis, N., Savvidaki, E., Kafetzopoulos, S., Manikis,
G., Vidakis, N., Marias, K., and Alissandrakis, E.
(2021). Segmenting 20 Types of Pollen Grains for
the Cretan Pollen Dataset v1 (CPD-1). Applied Sci-
ences, 11(14):6657. Number: 14 Publisher: Multidis-
ciplinary Digital Publishing Institute.
Viertel, P. and K
¨
onig, M. (2022). Pattern recognition
methodologies for pollen grain image classification:
a survey. Machine Vision and Applications, 33(1):18.
Viertel, P., K
¨
onig, M., and Rexilius, J. (2021). Pollen-
GAN: Synthetic Pollen Grain Image Generation for
Data Augmentation. In 2021 20th IEEE International
Conference on Machine Learning and Applications
(ICMLA), pages 44–49.
Vinyals, O., Blundell, C., Lillicrap, T., kavukcuoglu, k., and
Wierstra, D. (2016). Matching Networks for One Shot
Learning. In Advances in Neural Information Process-
ing Systems, volume 29. Curran Associates, Inc.
Walsh, R., Abdelpakey, M. H., Shehata, M. S., and Mo-
hamed, M. M. (2022). Automated human cell classifi-
cation in sparse datasets using few-shot learning. Sci
Rep, 12(1):2924. Number: 1 Publisher: Nature Pub-
lishing Group.
Zagoruyko, S. and Komodakis, N. (2015). Learning to
compare image patches via convolutional neural net-
works. In 2015 IEEE Conference on Computer Vision
and Pattern Recognition (CVPR), pages 4353–4361.
ISSN: 1063-6919.
Zhu, X. and Ghahramani, Z. (2002). Learning from Labeled
and Unlabeled Data with Label Propagation. Techni-
cal report, Carnegie Mellon University.
Metric-Based Few-Shot Learning for Pollen Grain Image Classification
425