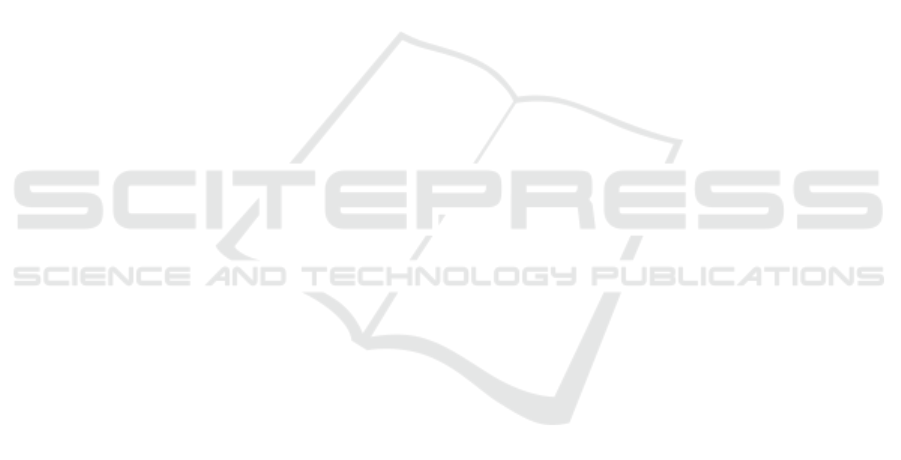
5.6 Network Convergence
Figure 6 plots the network validation loss during the
training process for DeepCaps and DeepCaps+. The
validation loss is the sum of the decoder (reconstruc-
tion) loss and the margin loss. As the figure shows,
both networks converge almost at the same epoch. In
the meantime, training DeepCaps+ is more stable than
DeepCaps. In addition, DeepCaps+ shows a slightly
higher final loss value compared to DeepCaps.
6 CONCLUSION
In this work, we present DeepCaps+ as a powerful
variant of Capsule-based Network, as an extension
of DeepCaps. This network performs competitively
compared to the state-of-the-art CapsNet-based net-
works. DeepCaps+ includes the 3D Dynamic rout-
ing and the class-independent decoder introduced by
Rajasegaran et al. (Rajasegaran et al., 2019) and in-
troduces the novel Capsule Assembler module in or-
der to reduce the primary capsules to speed up the
network and reduce the number of parameters while
maintaining the test accuracy. Using a 7-ensemble
model, DeepCaps+ obtains an accuracy of 91.63% for
the CIFAR-10 dataset using 7.3M parameters while
taking 1.71ms to test a single image. With 67.56%
test accuracy on the CIFAR-100 dataset, DeepCaps+
is among the few variants of vector-based CapsNet
that can process this dataset providing acceptable re-
sults.
ACKNOWLEDGEMENTS
This research has been funded in part or completely
by the Computing Hardware for Emerging Intelligent
Sensory Applications (COHESA) project. COHESA
is financed under the National Sciences and Engineer-
ing Research Council of Canada (NSERC) Strategic
Networks grant number NETGP485577-15.
REFERENCES
Ayidzoe, M. A., Yu, Y., Mensah, P. K., Cai, J., Adu, K., and
Tang, Y. (2021). Gabor capsule network with prepro-
cessing blocks for the recognition of complex images.
Machine Vision and Applications, 32(4).
Chen, J. and Liu, Z. (2020). Mask dynamic routing to com-
bined model of deep capsule network and u-net. IEEE
Transactions on Neural Networks and Learning Sys-
tems, 31(7):2653–2664.
Deli, A. (2018). HitNet : a neural network with capsules
embedded in a Hit-or-Miss layer , extended with hy-
brid data augmentation and ghost capsules. pages 1–
19.
Deng, J., Dong, W., Socher, R., Li, L.-J., Kai Li, and Li
Fei-Fei (2009). ImageNet: A large-scale hierarchical
image database. In 2009 IEEE Conference on Com-
puter Vision and Pattern Recognition, pages 248–255.
IEEE.
He, J., Cheng, X., He, J., and Xu, H. (2019). Cv-
CapsNet: Complex-valued capsule network. IEEE
Access, 7:85492–85499.
Huang, G., Liu, Z., van der Maaten, L., and Weinberger,
K. Q. (2016). Densely Connected Convolutional Net-
works.
Huang, W. and Zhou, F. (2020). DA-CapsNet: dual atten-
tion mechanism capsule network. Scientific Reports.
Kolesnikov, A., Beyer, L., Zhai, X., Puigcerver, J., Yung, J.,
Gelly, S., and Houlsby, N. (2019). Big Transfer (BiT):
General Visual Representation Learning.
Krizhevsky, A., Nair, V., and Hinton, G. (2009). CIFAR-10
and CIFAR-100 datasets.
LECUN and Y. THE MNIST DATABASE of handwritten
digits. http://yann.lecun.com/exdb/mnist/.
Rajasegaran, J., Jayasundara, V., Jayasekara, S., Jayasekara,
H., Seneviratne, S., and Rodrigo, R. (2019). Deep-
Caps: Going Deeper with Capsule Networks.
Sabour, S., Frosst, N., and Hinton, G. E. (2017). Dynamic
Routing Between Capsules. (Nips).
Shiri, P. and Baniasadi, A. (2021). Convolutional Fully-
Connected Capsule Network (CFC-CapsNet). In ACM
International Conference Proceeding Series.
Shiri, P., Sharifi, R., and Baniasadi, A. (2020). Quick-
CapsNet (QCN): A Fast Alternative to Capsule Net-
works. In Proceedings of IEEE/ACS International
Conference on Computer Systems and Applications,
AICCSA, volume 2020-Novem.
Tao, J., Zhang, X., Luo, X., Wang, Y., Song, C., and Sun, Y.
(2022). Adaptive capsule network. Computer Vision
and Image Understanding, 218:103405.
Xiao, H., Rasul, K., and Vollgraf, R. (2017). Fashion-
MNIST: a Novel Image Dataset for Benchmarking
Machine Learning Algorithms.
Yang, S., Lee, F., Miao, R., Cai, J., Chen, L., Yao, W.,
Kotani, K., and Chen, Q. (2020). RS-CapsNet: An
Advanced Capsule Network. IEEE Access.
VISAPP 2023 - 18th International Conference on Computer Vision Theory and Applications
220