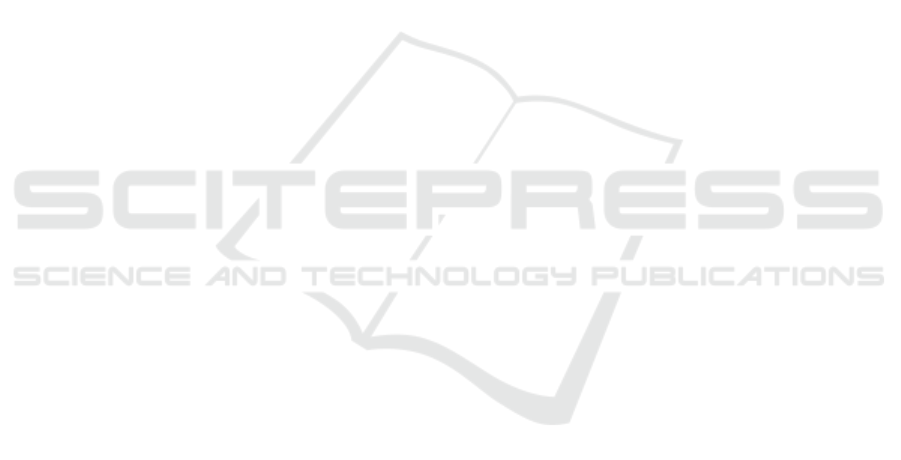
(specialized vocabulary), with the terms/words of the
application context, which allows to semantically
enrich the BoC method.
Experimental results were carried out with
the objective of analyzing the performance of the
proposed approach in the retrieval of legal documents
by calculating the semantic similarity of their vector
representations. The proposed BoLC-Th method was
compared with the traditional BoW, TF-IDF and BoC
approaches. The proposed method achieved better
performance when compared to the BoW model and,
on average, 40% more efficient than that obtained
with the BoC. Thus, a significant advantage was
demonstrated with the use of the BoLC-Th technique
for the analyzed case study. As future work, other
clustering techniques for the generation of concepts
should be considered.
The main contribution of the BoLC-Th is that it
incorporates the advantages of the BoC approach,
such as the compact representation, while enriching
legal documents representation as it considers
specific words/terms from the context area. This
is a valuable contribution to a domain area with
peculiar characteristics, providing a valuable tool for
retrieving textual information accurately and quickly.
REFERENCES
Analytics Vidhya, N. (2017). An intuitive understanding of
word embeddings: From count vectors to word2vec.
Dhillon, I. S. and Modha, D. S. (2001). Concept
decompositions for large sparse text data using
clustering. Machine learning, 42(1):143–175.
Hematialam, H., Garbayo, L., Gopalakrishnan, S.,
and Zadrozny, W. W. (2021). A method for
computing conceptual distances between medical
recommendations: Experiments in modeling medical
disagreement. Applied Sciences, 11(5).
Kim, H. K., Kim, H., and Cho, S. (2017). Bag-of-
concepts: Comprehending document representation
through clustering words in distributed representation.
Neurocomputing, 266:336–352.
Le, Q. and Mikolov, T. (2014). Distributed representations
of sentences and documents. In Xing, E. P. and Jebara,
T., editors, Proceedings of the 31st International
Conference on Machine Learning, volume 32 of
Proceedings of Machine Learning Research, pages
1188–1196, Bejing, China. PMLR.
Lee, Y. (2020). Systematic homonym detection and
replacement based on contextual word embedding.
53(1):17–36.
Lee, Y.-H., Hu, P. J.-H., Tsao, W.-J., and Li, L. (2021). Use
of a domain-specific ontology to support automated
document categorization at the concept level: Method
development and evaluation. 174:114681.
Li, P., Mao, K., Xu, Y., Li, Q., and Zhang, J. (2020). Bag-
of-concepts representation for document classification
based on automatic knowledge acquisition from
probabilistic knowledge base. Knowledge-based
systems, 193:105436.
Ling, W., Dyer, C., Black, A. W., and Trancoso, I. (2015).
Two/too simple adaptations of Word2Vec for syntax
problems. In Proceedings of the 2015 Conference
of the North American Chapter of the Association
for Computational Linguistics: Human Language
Technologies, pages 1299–1304, Denver, Colorado.
Association for Computational Linguistics.
Mehanna, Y. S. and Mahmuddin, M. B. (2021). A semantic
conceptualization using tagged bag-of-concepts for
sentiment analysis. IEEE access, 9:118736–118756.
Mikolov, T., Chen, K., Corrado, G., and Dean, J. (2013).
Efficient estimation of word representations in vector
space. arXiv preprint arXiv:1301.3781.
Mourino Garcia, M. A., Perez Rodriguez, R., and
Anido Rifon, L. E. (2015). Biomedical literature
classification using encyclopedic knowledge: a
wikipedia-based bag-of-concepts approach. PeerJ
(San Francisco, CA), 3:e1279–e1279.
Niebla Zatarain, J. M. (2018). Artificial intelligence and
legal analytics: New tools for law practice in the
digital age. SCRIPT-ed, 15:156–161. doi: https:
//doi.org/10.2966/scrip.150118.156.
Rajabi, Z., Valavi, M. R., and Hourali, M. (2020). A
context-based disambiguation model for sentiment
concepts using a bag-of-concepts approach. Cognitive
computation, 12(6):1299–1312.
Renjit, S. and Idicula, S. M. (2019). Cusat nlp@ aila-
fire2019: Similarity in legal texts using document
level embeddings. In FIRE (Working Notes), pages
25–30.
Salim, M. N. and Mustafa, B. S. (2022). A survey on
word representation in natural language processing.
In 1ST Samara International Conference for Pure
and Applied Science (SICPS2021): SICPS2021. AIP
Publishing. doi: https://doi.org/10.1063/5.0121147.
Sansone, C. and Sperl
´
ı, G. (2022). Legal information
retrieval systems: State-of-the-art and open issues.
Information Systems, 106:101967.
Shalaby, W. and Zadrozny, W. (2019). Learning
concept embeddings for dataless classification via
efficient bag-of-concepts densification. Knowledge
and Information Systems, 61(2):1047–1070.
Turney, P. D. (2006). Similarity of semantic relations.
Computational Linguistics, 32(3):379–416.
Wang, F., Wang, Z., Li, Z., and Wen, J.-R. (2014).
Concept-based short text classification and ranking.
In Proceedings of the 23rd ACM International
Conference on Conference on Information and
Knowledge Management, CIKM ’14, page
1069–1078, New York, NY, USA. Association
for Computing Machinery.
Yan, J. (2009). Text Representation, pages 3069–3072.
Springer US, Boston, MA.
Legal Information Retrieval Based on a Concept-Frequency Representation and Thesaurus
311