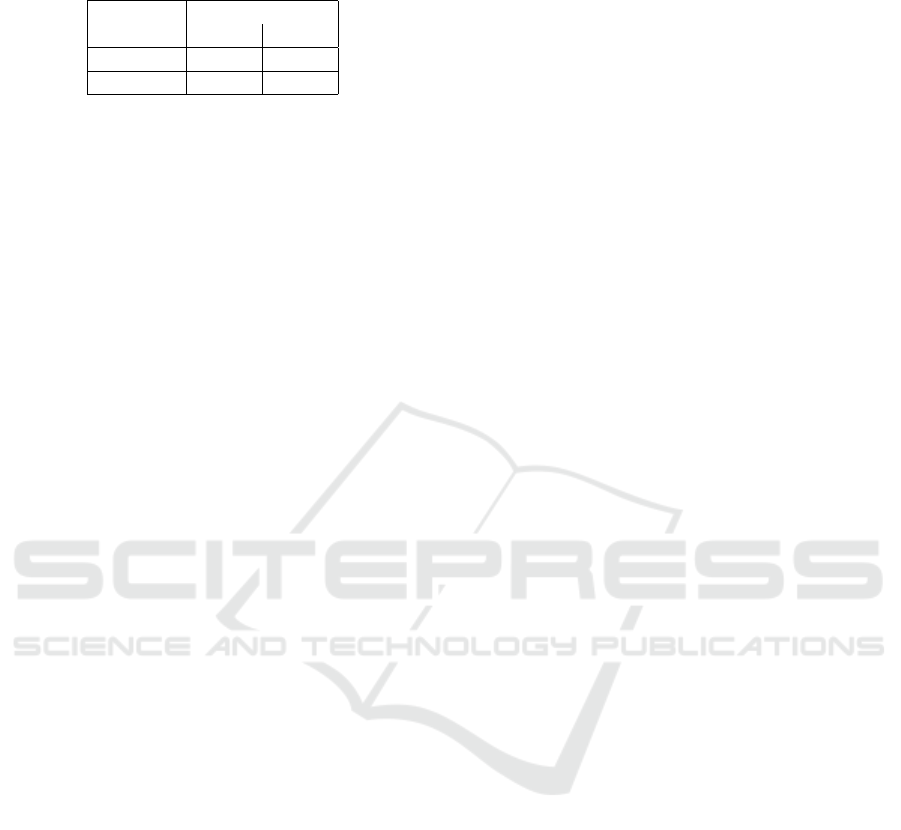
Table 6: Confusion matrix of sample authentication com-
puted from data sets P
1
C
1
b and P
1
C
1
s.
Actual
Predicted P
1
C
1
b P
1
C
1
s
P
1
C
1
b 98.7% 2.7%
P
1
C
1
s 1.3% 97.3%
tion applications. Additionally, the use of relatively
small data sets for training stage, motivates us to con-
tinue to study this approach for real-world authentica-
tion applications.
6 CONCLUSIONS
Medicine falsification is a big issue nowadays. We
need to find simple and relatively cheap protection
solutions. One of such solutions is the use of foren-
sics approaches. To print pharmaceutical packag-
ing printing manufactures use the rotogravure print-
ing technique that has some specific characteristics.
Previously, it was shown that the signature of the ro-
togravure cylinder can be easily identified using Pear-
son correlation. Nevertheless, the identification of the
rotogravure press and of the printing support were
shown to be a difficult problem. In this paper, we
investigated the use of the similarity metric learning
approach for these two identification scenarios.
Our experimental results prove the possibility to
easily identify both the printing support used and the
rotogravure press used for packaging pharmaceuti-
cal samples, using the similarity metric learning ap-
proach.
The next step will be to construct a full authen-
tication system using the proposed similarity metric
learning approach, and explore the possibility to use
a smartphone camera for authentication.
ACKNOWLEDGEMENTS
We would like to thanks the PAUSE Program: Emer-
gency Assistance to Ukrainian Researchers to support
the scientific stay of T. Yemelianenko in LIRIS lab-
oratory. This work was done in the context of the
FakeNets project funded by F
´
ed
´
eration Informatique
de Lyon. All the printed samples were provided by
Sergusa Solutions Pvt Ltd in the context of PackMark
project (IFCPAR-7127) supported by the Indo-French
Center for the Promotion of Advanced Research.
REFERENCES
Bromley, J., Guyon, I., LeCun, Y., S
¨
ackinger, E., and Shah,
R. (1993). Signature verification using a” siamese”
time delay neural network. Advances in neural infor-
mation processing systems, 6.
Davison, M. (2011). Pharmaceutical anti-counterfeiting:
combating the real danger from fake drugs. John Wi-
ley & Sons.
Duffner, S., Garcia, C., Idrissi, K., and Baskurt, A. (2021).
Similarity metric learning. In Multi-faceted Deep
Learning, pages 103–125. Springer.
He, K., Zhang, X., Ren, S., and Sun, J. (2016). Deep
residual learning for image recognition. In IEEE Con-
ference on Computer Vision and Pattern Recognition,
CVPR 2016, Las Vegas, NV, USA, June 27-30, 2016,
pages 770–778. IEEE Computer Society.
Joshi, S., Saxena, S., and Khanna, N. (2020). Source printer
identification from document images acquired using
smartphone. arXiv preprint arXiv:2003.12602.
Kipphan, H. (2001). Handbook of print media: technolo-
gies and production methods. Springer Science &
Business Media.
Nguyen, Q.-T., Mai, A., Chagas, L., and Reverdy-Bruas, N.
(2021). Microscopic printing analysis and application
for classification of source printer. Computers & Se-
curity, 108:102320.
Schraml, R., Debiasi, L., Kauba, C., and Uhl, A. (2017). On
the feasibility of classification-based product package
authentication. In Information Forensics and Security
(WIFS), 2017 IEEE Workshop on, pages 1–6. IEEE.
Schraml, R., Debiasi, L., and Uhl, A. (2018). Real or
fake: Mobile device drug packaging authentication.
In Proceedings of the 6th ACM Workshop on Informa-
tion Hiding and Multimedia Security, pages 121–126.
ACM.
Simonyan, K. and Zisserman, A. (2015). Very deep convo-
lutional networks for large-scale image recognition. In
3rd International Conference on Learning Represen-
tations, ICLR 2015, San Diego, CA, USA, May 7-9,
2015, Conference Track Proceedings.
Tkachenko, I., Tr
´
emeau, A., and Fournel, T. (2019). Au-
thentication of medicine blister foils: Characterization
of the rotogravure printing process. In VISIGRAPP (4:
VISAPP), pages 577–583.
Tkachenko, I., Tr
´
emeau, A., and Fournel, T. (2020). Fight-
ing against medicine packaging counterfeits: ro-
togravure press vs cylinder signatures. In 2020 IEEE
International Workshop on Information Forensics and
Security (WIFS), pages 1–6. IEEE.
Tkachenko, I., Tr
´
emeau, A., and Fournel, T. (2022). Au-
thentication of rotogravure print-outs using a regular
test pattern. Journal of Information Security and Ap-
plications, 66:103133.
Tsai, M.-J., Tao, Y.-H., and Yuadi, I. (2019). Deep learn-
ing for printed document source identification. Signal
Processing: Image Communication, 70:184–198.
Weinberger, K. Q., Blitzer, J., and Saul, L. (2005). Distance
metric learning for large margin nearest neighbor clas-
sification. Advances in neural information processing
systems, 18.
Printed Packaging Authentication: Similarity Metric Learning for Rotogravure Manufacture Process Identification
911