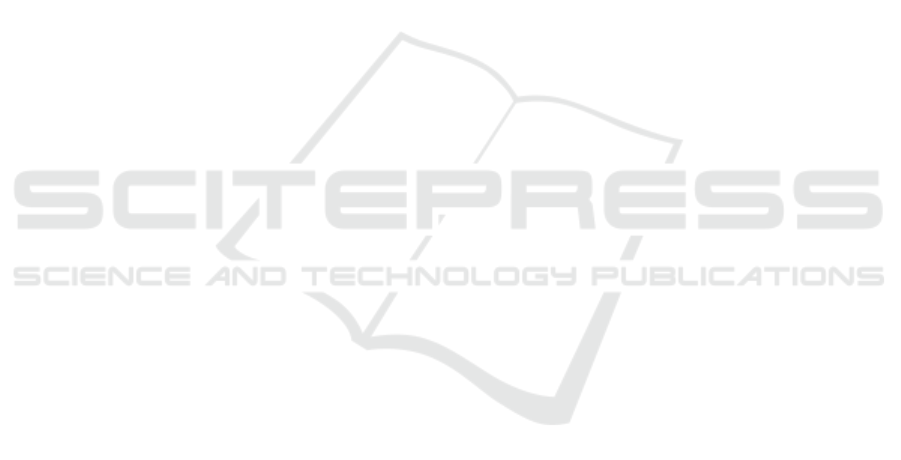
tifacts can help streamlining and accelerating model
development; (iv) training, and ”mind set” on behalf
of engineers, domain experts and other stakeholders,
recognizing in advance the magnitude of the mod-
eling task, and the number and size of the artifacts
involved; (v) building domain specific solutions and
tools as a step toward more general solutions.
Future tasks include the study additional such is-
sues and challenges, some of which are listed in Sec-
tion 1, and the development of complex models sub-
ject to the informally documented approaches and
techniques; these can serve as proofs-of-concept to
the incipient modeling methodologies.
Such research and development should contribute
to the methodologies, languages and tools of model
development and model assessment, and hence, to the
usefulness of models in science and society.
ACKNOWLEDGMENTS
We thank the anonymous reviewers for their insight-
ful comments and suggestions. This work was par-
tially supported by a research grants to David Harel
from the Estate of Harry Levine, the Estate of Avra-
ham Rothstein, Brenda Gruss and Daniel Hirsch, the
One8 Foundation, Rina Mayer, Maurice Levy, and the
Estate of Bernice Bernath, a grant 3698/21 from the
ISF-NSFC joint to the Israel Science Foundation and
the National Science Foundation of China, and a grant
from the Minerva foundation.
REFERENCES
Armoni, M., Gal-Ezer, J., Ittah, M. H., Marelly, R., and
Szekely, S. (2021). Computational problem solving in
plethora. In Informatics in Schools: Situation, Evolu-
tion, and Perspectives, ISSEP.
Blaser, M. J. (2014). The microbiome revolution. J. of
Clinical Investigation, 124(10):4162–4165.
Briand, L., Nejati, S., Sabetzadeh, M., and Bianculli, D.
(2016). Testing the untestable: model testing of com-
plex software-intensive systems. In ICSE Companion,
pages 789–792.
Bucchiarone, A., Cabot, J., Paige, R. F., and Pierantonio, A.
(2020). Grand challenges in model-driven engineer-
ing: an analysis of the state of the research. Softw.
and Sys. Modeling, 19(1):5–13.
Christin, S., Hervet,
´
E., and Lecomte, N. (2021). Going fur-
ther with model verification and deep learning. Meth-
ods in Ecology and Evolution, 12(1):130–134.
Cohen, I. R. and Marron, A. (2020). The evolution of
universal adaptations of life is driven by universal
properties of matter: energy, entropy, and interaction.
F1000Research, 9.
Cohen, I. R. and Marron, A. (2022). The biosphere com-
putes evolution by autoencoding interacting organ-
isms into species and decoding species into ecosys-
tems. arXiv preprint arXiv:2203.11891.
Harel, D. and Kugler, H. (2004). The rhapsody seman-
tics of statecharts (or, on the executable core of the
UML). In Integration of Software Specification Tech-
niques for Applications in Engineering, pages 325–
354. Springer.
Haskel-Ittah, M. (2022). Explanatory black boxes and
mechanistic reasoning. Journal of Research in Sci-
ence Teaching.
Karr, J. R., Takahashi, K., and Funahashi, A. (2015). The
principles of whole-cell modeling. Current opinion in
microbiology, 27:18–24.
Liu, M., Fang, S., Dong, H., and Xu, C. (2021). Review of
digital twin about concepts, technologies, and indus-
trial applications. Journal of Manufacturing Systems,
58:346–361.
Mencuccini, M., Manzoni, S., and Christoffersen, B.
(2019). Modelling water fluxes in plants: from tissues
to biosphere. New Phytologist, 222(3):1207–1222.
Rosenberg, E., Koren, O., Reshef, L., Efrony, R., and
Zilber-Rosenberg, I. (2007). The role of microorgan-
isms in coral health, disease and evolution. Nature
Rev. Microbiology, 5(5):355–362.
Sankararaman, S. and Mahadevan, S. (2015). Integration
of model verification, validation, and calibration for
uncertainty quantification in engineering systems. Re-
liability Engineering & System Safety, 138:194–209.
Saprykin, A., Chokani, N., and Abhari, R. S. (2019). Gem-
sim: A GPU-accelerated multi-modal mobility simu-
lator for large-scale scenarios. Simulation Modelling
Practice and Theory, 94:199–214.
Schr
¨
odinger, E. (1944). What is life? The physical aspect of
the living cell and mind. Cambridge University Press
Cambridge.
Simard, S. W. (2018). Mycorrhizal networks facilitate tree
communication, learning, and memory. In Memory
and learning in plants, pages 191–213. Springer.
Troya, J., Moreno, N., Bertoa, M. F., and Vallecillo, A.
(2021). Uncertainty representation in software mod-
els: a survey. Software and Systems Modeling,
20(4):1183–1213.
Woodward, J. L. and Pitbaldo, R. (2010). LNG risk based
safety: modeling and consequence analysis. John Wi-
ley & Sons.
Challenges in Modeling and Unmodeling Emergence, Rule Composition, and Networked Interactions in Complex Reactive Systems
209