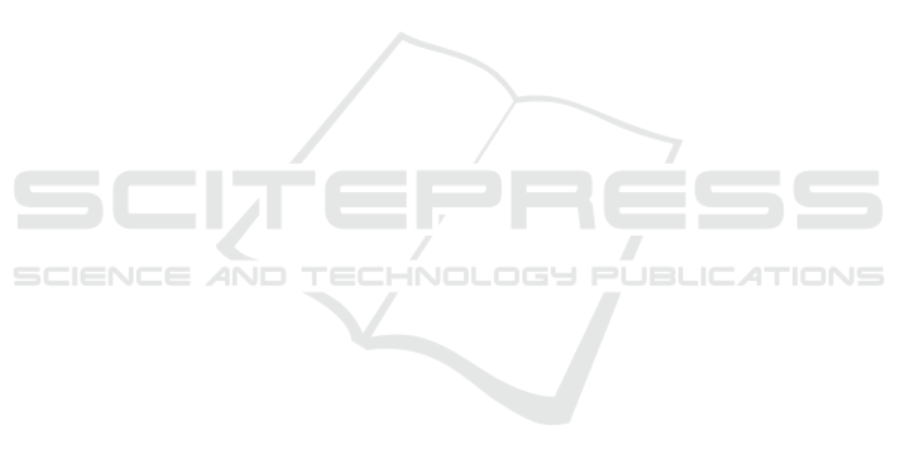
16th International Symposium on Biomedical Imag-
ing, pages 1008–1012.
Badrinarayanan, V., Kendall, A., and Cipolla, R. (2017).
Segnet: A deep convolutional encoder-decoder archi-
tecture for image segmentation. In Pattern Analysis
and Machine Intelligence, volume 39, pages 2481–
2495.
Chen, L. C., Zhu, Y., Papandreou, G., Schroff, F., and
Adam, H. (2018). Encoder-decoder with atrous sepa-
rable convolution for semantic image segmentation. In
Proceedings of the European conference on computer
vision, pages 801–818.
Edlund, C., Jackson, T. R., Khalid, N., Bevan, N., Dale,
T., Dengel, A., Ahmed, S., Trygg, J., and Sj
¨
ogren, R.
(2021). Livecell—a large-scale dataset for label-free
live cell segmentation. In Nature methods, volume 18,
pages 1038–1045.
Gerhard, S., Funke, J., Martel, J., Cardona, A., and Fet-
ter, R. (2013). Segmented anisotropic sstem dataset of
neural tissue. In figshare.
Hiramatsu, Y., Hotta, K., Imanishi, A., Matsuda, M., and
Terai, K. (2018). Cell image segmentation by inte-
grating multiple cnns. In IEEE/CVF Conference on
Computer Vision and Pattern Recognition Workshops.
https://medicalsegmentation.com/covid19/ (2020). Covid-
19 ct segmentation dataset.
Jacobs, R. A., Jordan, M. I., Nowlan, S. J., and Hinton, G. E.
(1991). Adaptive mixtures of local experts. In Neural
Computation, volume 3, pages 79–87.
Kumagai, S., Hotta, K., and Kurita, T. (2018). Mixture of
counting cnns. In Machine Vision and Applications,
volume 29, pages 1119–1126.
Lin, T. Y., Dollar, P., Girshick, R., He, K., Hariharan, B.,
and Belongie, S. (2017). Feature pyramid networks
for object detection. In Proceedings of the IEEE con-
ference on computer vision and pattern recognition,
pages 2117–2125.
Long, J., Shelhamer, E., and Darrell, T. (2015). Fully con-
volutional networks for semantic segmentation. In
Proceedings of the IEEE conference on computer vi-
sion and pattern recognition, pages 3431–3440.
Ma, N., x. Zhang, Zheng, H. T., and Sun, J. (2018). Shuf-
flenet v2: Practical guidelines for efficient cnn archi-
tecture design. In Proceedings of the European con-
ference on computer vision, pages 116–131.
Milletari, F., Navab, N., and Ahmadi, S. A. (2016). V-
net: Fully convolutional neural networks for volumet-
ric medical image segmentation. In Proceedings of In-
ternational Conference on 3D Vision, pages 565–571.
Ronneberge, O., Fischer, P., and Brox, T. (2015). U-net:
Convolutional networks for biomedical image seg-
mentation. In Proceedings of International Confer-
ence on Medical Image Computing and Computer-
Assisted Intervention, pages 234–241.
Sun, K., Xiao, B., Liu, D., and Wang, J. (2019). Deep high-
resolution representation learning for human pose es-
timation. In Proc. IEEE/CVF Conference on Com-
puter Vision and Pattern Recognition, pages 5693–
5703.
Sun, K., Zhao, Y., Jiang, B., Cheng, T., Xiao, B., Liu, D.,
Mu, Y., Wang, X., Liu, W., and Wang, J. (2020). High-
resolution representations for labeling pixels and re-
gions. In arXiv:1904.04514.
Wang, J., Sun, K., Cheng, T., Jiang, B., Deng, C., Zhao, Y.,
Liu, D., Mu, Y., Tan, M., Wang, X., Liu, W., and Xiao,
B. (2020). Deep high-resolution representation learn-
ing for visual recognition. In IEEE transactions on
pattern analysis and machine intelligence, volume 43,
pages 3349–3364.
Yuan, Y., Chen, X., Chen, X., and Wang, J. (2019). Segmen-
tation transformer: Object-contextual representations
for semantic segmentation. In arXiv:1909.11065.
Yuan, Y., Fu, R., Huang, L., Lin, W., Zhang, C., Chen,
X., and Wang, J. (2021). Hrformer: High-resolution
transformer for dense prediction. In Neural Infor-
mation Processing Systems, volume 34, pages 7281–
7293.
Zhao, H., Shi, J., Qi, X., Wang, Z., and Jia, J. (2017). Pyra-
mid scene parsing network. In Proceeding of the IEEE
Conference on Computer Vision and Pattern Recogni-
tion, pages 2881–2890.
VISAPP 2023 - 18th International Conference on Computer Vision Theory and Applications
400