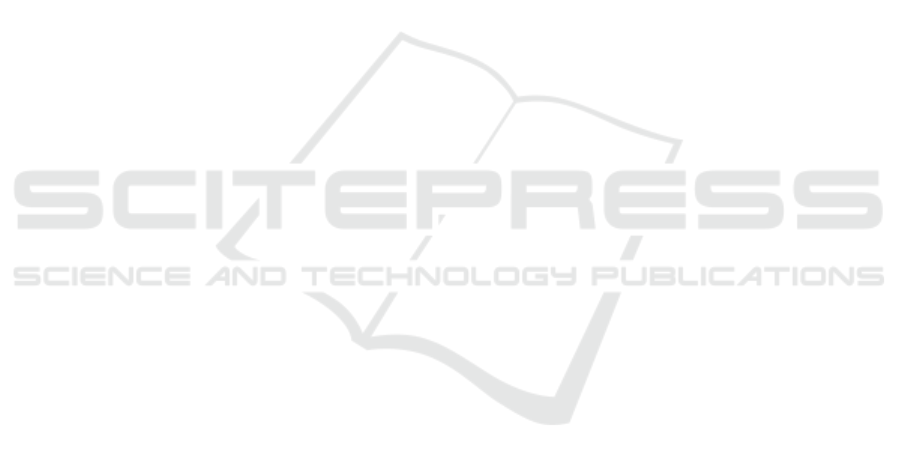
4 CONCLUSION
In this paper, we propose a parallel multi-branch net-
work, combining the advantages of both the convo-
lution and transformer, which can capture rich de-
tailed features while modelling the long-range rela-
tions. Besides, the Intensive attention mechanism
is utilized in the network, which enables the net-
work to gain multi-model feature maps with differ-
ent resolutions for better representations and focus
global attention weight rapidly on sparse and mean-
ingful locations. Additionally, we propose a novel
and effective loss function, Smooth Wing Loss, which
steadily accelerates the convergence speed of the net-
work and can further converge at the later training
stage. Extensive experiments show that IACT outper-
forms the state-of-the-art methods, and the ablation
studies prove the effectiveness of the proposed meth-
ods.
REFERENCES
Belhumeur, P. N., Jacobs, D. W., Kriegman, D. J., and Ku-
mar, N. (2013). Localizing parts of faces using a
consensus of exemplars. IEEE transactions on pat-
tern analysis and machine intelligence, 35(12):2930–
2940.
Burgos-Artizzu, X. P., Perona, P., and Doll
´
ar, P. (2013).
Robust face landmark estimation under occlusion. In
Proceedings of the IEEE international conference on
computer vision, pages 1513–1520.
Carion, N., Massa, F., Synnaeve, G., Usunier, N., Kirillov,
A., and Zagoruyko, S. (2020). End-to-end object de-
tection with transformers. In European conference on
computer vision, pages 213–229. Springer.
Dai, J., Qi, H., Xiong, Y., Li, Y., Zhang, G., Hu, H., and
Wei, Y. (2017). Deformable convolutional networks.
In Proceedings of the IEEE international conference
on computer vision, pages 764–773.
Dapogny, A., Bailly, K., and Cord, M. (2019a). Decafa:
Deep convolutional cascade for face alignment in the
wild. In Proceedings of the IEEE/CVF International
Conference on Computer Vision, pages 6893–6901.
Dapogny, A., Bailly, K., and Cord, M. (2019b). Decafa:
Deep convolutional cascade for face alignment in the
wild. In Proceedings of the IEEE/CVF International
Conference on Computer Vision, pages 6893–6901.
Deng, J., Trigeorgis, G., Zhou, Y., and Zafeiriou, S. (2019).
Joint multi-view face alignment in the wild. IEEE
Transactions on Image Processing, 28(7):3636–3648.
Dong, X., Yan, Y., Ouyang, W., and Yang, Y. (2018). Style
aggregated network for facial landmark detection. In
Proceedings of the IEEE Conference on Computer Vi-
sion and Pattern Recognition, pages 379–388.
Dong, X., Yang, Y., Wei, S.-E., Weng, X., Sheikh, Y.,
and Yu, S.-I. (2020). Supervision by registration and
triangulation for landmark detection. IEEE transac-
tions on pattern analysis and machine intelligence,
43(10):3681–3694.
Dosovitskiy, A., Beyer, L., Kolesnikov, A., Weissenborn,
D., Zhai, X., Unterthiner, T., Dehghani, M., Minderer,
M., Heigold, G., Gelly, S., et al. (2020). An image is
worth 16x16 words: Transformers for image recogni-
tion at scale. arXiv preprint arXiv:2010.11929.
Feng, Z.-H., Kittler, J., Awais, M., Huber, P., and Wu, X.-J.
(2018). Wing loss for robust facial landmark localisa-
tion with convolutional neural networks. In Proceed-
ings of the IEEE conference on computer vision and
pattern recognition, pages 2235–2245.
Guo, X., Li, S., Yu, J., Zhang, J., Ma, J., Ma, L., Liu, W.,
and Ling, H. (2019). Pfld: A practical facial landmark
detector. arXiv preprint arXiv:1902.10859.
He, K., Zhang, X., Ren, S., and Sun, J. (2015).
Deep residual learning for image recognition. cite
arxiv:1512.03385Comment: Tech report.
Huang, Y., Yang, H., Li, C., Kim, J., and Wei, F. (2021). Ad-
net: Leveraging error-bias towards normal direction
in face alignment. In Proceedings of the IEEE/CVF
International Conference on Computer Vision, pages
3080–3090.
Jin, H., Liao, S., and Shao, L. (2021). Pixel-in-pixel
net: Towards efficient facial landmark detection in
the wild. International Journal of Computer Vision,
129(12):3174–3194.
Kingma, D. P. and Ba, J. (2014). Adam: A
method for stochastic optimization. arXiv preprint
arXiv:1412.6980.
Kowalski, M., Naruniec, J., and Trzcinski, T. (2017). Deep
alignment network: A convolutional neural network
for robust face alignment. In Proceedings of the IEEE
conference on computer vision and pattern recogni-
tion workshops, pages 88–97.
Kumar, A., Marks, T. K., Mou, W., Wang, Y., Jones, M.,
Cherian, A., Koike-Akino, T., Liu, X., and Feng, C.
(2020). Luvli face alignment: Estimating landmarks’
location, uncertainty, and visibility likelihood. In Pro-
ceedings of the IEEE/CVF Conference on Computer
Vision and Pattern Recognition, pages 8236–8246.
Le, V., Brandt, J., Lin, Z., Bourdev, L., and Huang, T. S.
(2012). Interactive facial feature localization. In Euro-
pean conference on computer vision, pages 679–692.
Springer.
Li, W., Lu, Y., Zheng, K., Liao, H., Lin, C., Luo, J., Cheng,
C.-T., Xiao, J., Lu, L., Kuo, C.-F., et al. (2020). Struc-
tured landmark detection via topology-adapting deep
graph learning. In European Conference on Computer
Vision, pages 266–283. Springer.
Lin, C., Zhu, B., Wang, Q., Liao, R., Qian, C., Lu, J.,
and Zhou, J. (2021). Structure-coherent deep feature
learning for robust face alignment. IEEE Transactions
on Image Processing, 30:5313–5326.
Liu, Z., Lin, Y., Cao, Y., Hu, H., Wei, Y., Zhang, Z., Lin,
S., and Guo, B. (2021). Swin transformer: Hierarchi-
cal vision transformer using shifted windows. In Pro-
ceedings of the IEEE/CVF International Conference
on Computer Vision, pages 10012–10022.
IACT: Intensive Attention in Convolution-Transformer Network for Facial Landmark Localization
409