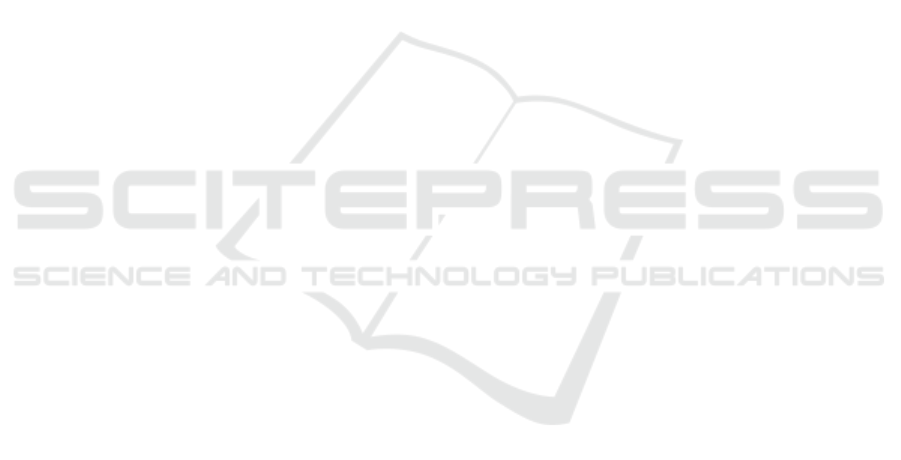
ACKNOWLEDGEMENTS
MF gratefully acknowledges support from the AXA
Research Fund and the Agence Nationale de la
Recherche (grant ANR-18-CE46-0002 and ANR-19-
P3IA-0002).
REFERENCES
Blundell, C., Cornebise, J., Kavukcuoglu, K., and Wierstra,
D. (2015). Weight uncertainty in neural network. In
International conference on machine learning, pages
1613–1622. PMLR.
Chen, T., Fox, E., and Guestrin, C. (2014). Stochastic Gra-
dient Hamiltonian Monte Carlo. In Xing, E. P. and
Jebara, T., editors, Proceedings of the 31st Interna-
tional Conference on Machine Learning, volume 32
of Proceedings of Machine Learning Research, pages
1683–1691, Bejing, China. PMLR.
Cutajar, K., Bonilla, E. V., Michiardi, P., and Filippone, M.
(2017). Random feature expansions for deep Gaus-
sian processes. In Precup, D. and Teh, Y. W., editors,
Proceedings of the 34th International Conference on
Machine Learning, volume 70 of Proceedings of Ma-
chine Learning Research, pages 884–893. PMLR.
Dua, D. and Graff, C. (2017). UCI machine learning repos-
itory.
Fortuin, V. (2022). Priors in bayesian deep learning: A re-
view. International Statistical Review.
Fortuin, V., Garriga-Alonso, A., Wenzel, F., Ratsch, G.,
Turner, R. E., van der Wilk, M., and Aitchison, L.
(2021). Bayesian neural network priors revisited.
In Third Symposium on Advances in Approximate
Bayesian Inference.
Graves, A. (2011). Practical variational inference for neural
networks. In Shawe-Taylor, J., Zemel, R., Bartlett, P.,
Pereira, F., and Weinberger, K., editors, Advances in
Neural Information Processing Systems, volume 24.
Curran Associates, Inc.
Kallenberg, O. and Kallenberg, O. (1997). Foundations of
modern probability, volume 2. Springer.
Kanagawa, M., Hennig, P., Sejdinovic, D., and Sriperum-
budur, B. K. (2018). Gaussian processes and kernel
methods: A review on connections and equivalences.
arXiv preprint arXiv:1807.02582.
Lakshminarayanan, B., Pritzel, A., and Blundell, C. (2017).
Simple and scalable predictive uncertainty estimation
using deep ensembles. In Guyon, I., Luxburg, U. V.,
Bengio, S., Wallach, H., Fergus, R., Vishwanathan,
S., and Garnett, R., editors, Advances in Neural Infor-
mation Processing Systems, volume 30. Curran Asso-
ciates, Inc.
Ma, C., Li, Y., and Hernandez-Lobato, J. M. (2019). Vari-
ational implicit processes. In Chaudhuri, K. and
Salakhutdinov, R., editors, Proceedings of the 36th
International Conference on Machine Learning, vol-
ume 97 of Proceedings of Machine Learning Re-
search, pages 4222–4233. PMLR.
Milios, D., Camoriano, R., Michiardi, P., Rosasco, L.,
and Filippone, M. (2018). Dirichlet-based gaussian
processes for large-scale calibrated classification. In
Bengio, S., Wallach, H., Larochelle, H., Grauman,
K., Cesa-Bianchi, N., and Garnett, R., editors, Ad-
vances in Neural Information Processing Systems,
volume 31. Curran Associates, Inc.
Neal, R. (1996). Bayesian learning for neural networks.
Lecture Notes in Statistics.
Rahimi, A. and Recht, B. (2007). Random features for
large-scale kernel machines. In Platt, J., Koller, D.,
Singer, Y., and Roweis, S., editors, Advances in Neu-
ral Information Processing Systems, volume 20. Cur-
ran Associates, Inc.
Rudner, T. G. J., Chen, Z., and Gal, Y. (2021). Rethinking
function-space variational inference in bayesian neu-
ral networks. In Third Symposium on Advances in Ap-
proximate Bayesian Inference.
Shi, J., Sun, S., and Zhu, J. (2018). A spectral approach to
gradient estimation for implicit distributions. In Dy, J.
and Krause, A., editors, Proceedings of the 35th Inter-
national Conference on Machine Learning, volume 80
of Proceedings of Machine Learning Research, pages
4644–4653. PMLR.
Sun, S., Zhang, G., Shi, J., and Grosse, R. (2019). Func-
tional Variational Bayesian Neural Networks. In In-
ternational Conference on Learning Representations.
Tran, B.-H., Rossi, S., Milios, D., and Filippone, M. (2022).
All you need is a good functional prior for bayesian
deep learning. Journal of Machine Learning Re-
search, 23(74):1–56.
Wenk, P., Gotovos, A., Bauer, S., Gorbach, N. S., Krause,
A., and Buhmann, J. M. (2019). Fast gaussian process
based gradient matching for parameter identification
in systems of nonlinear odes. In Chaudhuri, K. and
Sugiyama, M., editors, Proceedings of the Twenty-
Second International Conference on Artificial Intelli-
gence and Statistics, volume 89 of Proceedings of Ma-
chine Learning Research, pages 1351–1360. PMLR.
Wenzel, F., Roth, K., Veeling, B., Swiatkowski, J., Tran, L.,
Mandt, S., Snoek, J., Salimans, T., Jenatton, R., and
Nowozin, S. (2020). How good is the Bayes poste-
rior in deep neural networks really? In III, H. D. and
Singh, A., editors, Proceedings of the 37th Interna-
tional Conference on Machine Learning, volume 119
of Proceedings of Machine Learning Research, pages
10248–10259. PMLR.
Williams, C. K. and Rasmussen, C. E. (2006). Gaussian
processes for machine learning, volume 2. MIT press
Cambridge, MA.
Imposing Functional Priors on Bayesian Neural Networks
457