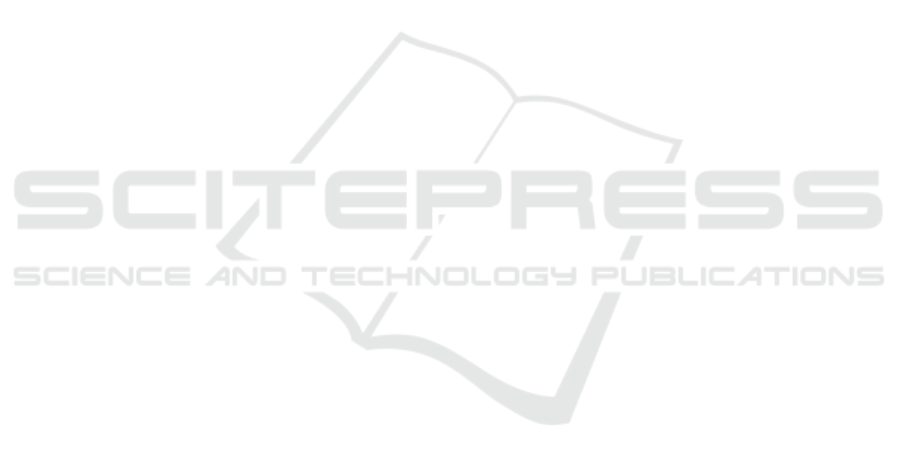
ACKNOWLEDGEMENTS
Work performed while A. Karakasidis was cooperat-
ing with Athens University of Economics and Busi-
ness. This research has been funded by the Human
Brain Project (HBP) - SGA3, Grant agreement no
945539.
REFERENCES
Acar, A., Aksu, H., Uluagac, A. S., and Conti, M. (2018).
A survey on homomorphic encryption schemes: The-
ory and implementation. ACM Computing Surveys,
51(4):1–35.
Basin, D., Debois, S., and Hildebrandt, T. (2018). On pur-
pose and by necessity: compliance under the gdpr. In
International Conference on Financial Cryptography
and Data Security, pages 20–37. Springer.
Belhajjame, K., Faci, N., Maamar, Z., Bur
´
egio, V., Soares,
E., and Barhamgi, M. (2020). On privacy-aware
escience workflows. Computing, 102(5):1171–1185.
Besik, S. I. and Freytag, J.-C. (2019). Ontology-based pri-
vacy compliance checking for clinical workflows. In
LWDA, pages 218–229.
Besik, S. I. and Freytag, J.-C. (2020). Managing consent in
workflows under gdpr. In ZEUS, pages 18–25.
Dumas, M., Garc
´
ıa-Ba
˜
nuelos, L., and Laud, P. (2016). Dif-
ferential privacy analysis of data processing work-
flows. In International Workshop on Graphical Mod-
els for Security, pages 62–79. Springer.
Dwork, C. (2008). Differential privacy: A survey of results.
In International conference on theory and applica-
tions of models of computation, pages 1–19. Springer.
Eke, D., Aasebø, I. E., Akintoye, S., Knight, W., Karaka-
sidis, A., Mikulan, E., Ochang, P., Ogoh, G., Oosten-
veld, R., Pigorini, A., et al. (2021). Pseudonymisation
of neuroimages and data protection: increasing access
to data while retaining scientific utility. Neuroimage:
Reports, 1(4):100053.
European Union (2016-05-04). Regulation 2016/679. Offi-
cial Journal L110, 59:1–78.
Garijo, D., Corcho, O., Gil, Y., Braskie, M. N., Hibar,
D., Hua, X., Jahanshad, N., Thompson, P., and Toga,
A. W. (2014). Workflow reuse in practice: A study of
neuroimaging pipeline users. In 2014 IEEE 10th In-
ternational Conference on e-Science, volume 1, pages
239–246. IEEE.
Gazula, H., Kelly, R., Romero, J., Verner, E., Baker, B. T.,
Silva, R. F., Imtiaz, H., Saha, D. K., Raja, R., Turner,
J. A., et al. (2020). Coinstac: Collaborative in-
formatics and neuroimaging suite toolkit for anony-
mous computation. Journal of Open Source Software,
5(54):2166.
Gkoulalas-Divanis, A. and Loukides, G. (2015). Medical
data privacy handbook. Springer.
Imtiaz, H. and Sarwate, A. D. (2018). Distributed differ-
entially private algorithms for matrix and tensor fac-
torization. IEEE journal of selected topics in signal
processing, 12(6):1449–1464.
Kaissis, G. A., Makowski, M. R., R
¨
uckert, D., and Braren,
R. F. (2020). Secure, privacy-preserving and feder-
ated machine learning in medical imaging. Nature
Machine Intelligence, 2(6):305–311.
Kalavathi, P. and Prasath, V. (2016). Methods on skull strip-
ping of mri head scan images—a review. Journal of
digital imaging, 29(3):365–379.
Milchenko, M. and Marcus, D. (2013). Obscuring surface
anatomy in volumetric imaging data. Neuroinformat-
ics, 11(1):65–75.
Plis, S. M., Sarwate, A. D., Wood, D., Dieringer, C., Landis,
D., Reed, C., Panta, S. R., Turner, J. A., Shoemaker,
J. M., Carter, K. W., et al. (2016). Coinstac: a privacy
enabled model and prototype for leveraging and pro-
cessing decentralized brain imaging data. Frontiers in
neuroscience, 10:365.
Sarwate, A. D., Plis, S. M., Turner, J. A., Arbabshirani,
M. R., and Calhoun, V. D. (2014). Sharing privacy-
sensitive access to neuroimaging and genetics data: a
review and preliminary validation. Frontiers in neu-
roinformatics, 8:35.
Savio, A. M., Schutte, M., Gra
˜
na, M., and Yakushev, I.
(2017). Pypes: workflows for processing multimodal
neuroimaging data. Frontiers in Neuroinformatics,
11:25.
Scherer, J., Nolden, M., Kleesiek, J., Metzger, J., Kades,
K., Schneider, V., Bach, M., Sedlaczek, O., Bucher,
A. M., Vogl, T. J., et al. (2020). Joint imaging plat-
form for federated clinical data analytics. JCO Clini-
cal Cancer Informatics, 4:1027–1038.
Schimke, N. and Hale, J. (2011). Quickshear defacing for
neuroimages. In HealthSec.
Schimke, N. and Hale, J. (2015). Privacy considerations and
techniques for neuroimages. In Medical Data Privacy
Handbook, pages 527–547. Springer.
Schwarz, C. G., Kremers, W. K., Wiste, H. J., Gunter, J. L.,
Vemuri, P., Spychalla, A. J., Kantarci, K., Schultz,
A. P., Sperling, R. A., Knopman, D. S., et al. (2021).
Changing the face of neuroimaging research: Com-
paring a new mri de-facing technique with popular al-
ternatives. NeuroImage, 231:117845.
Senanayake, N., Podschwadt, R., Takabi, D., Calhoun,
V. D., and Plis, S. M. (2021). Neurocrypt: Ma-
chine learning over encrypted distributed neuroimag-
ing data. Neuroinformatics, pages 1–18.
Silva, S., Altmann, A., Gutman, B., and Lorenzi, M. (2020).
Fed-biomed: A general open-source frontend frame-
work for federated learning in healthcare. In Do-
main Adaptation and Representation Transfer, and
Distributed and Collaborative Learning, pages 201–
210. Springer.
White, T., Blok, E., and Calhoun, V. D. (2022). Data sharing
and privacy issues in neuroimaging research: Oppor-
tunities, obstacles, challenges, and monsters under the
bed. Human Brain Mapping, 43(1):278–291.
Zhao, C., Zhao, S., Zhao, M., Chen, Z., Gao, C.-Z., Li, H.,
and Tan, Y.-a. (2019). Secure multi-party computa-
tion: theory, practice and applications. Information
Sciences, 476:357–372.
On the Design of GDPR Compliant Workflows for Responsible Neuroimage Data Sharing
607