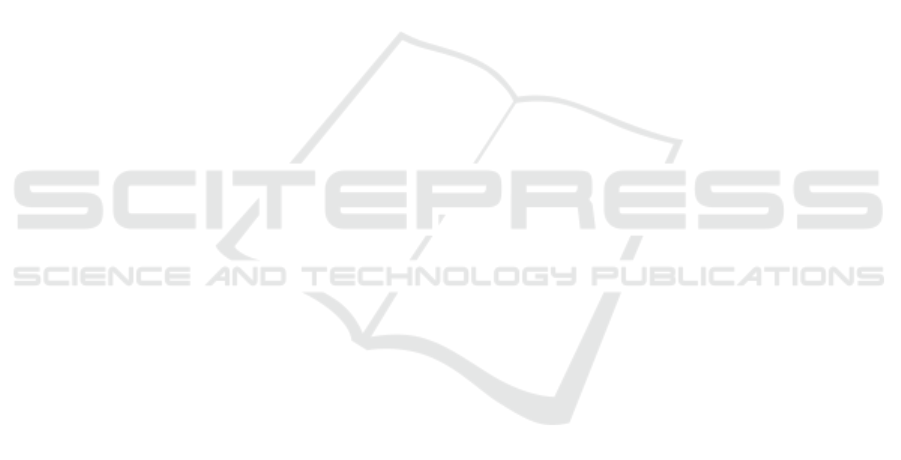
an initial foray into using a 2DFT technique to per-
form material classification of such manuscripts. The
ability to quickly classify the writing surface material
has the potential to expedite the initial manuscript in-
vestigation. The straightforward approach presented
here may be used as a starting point to help resolve
any debate over the nature of a DSS fragment’s ma-
terial and may be applied to other ancient historical
manuscripts. Furthermore, by building upon and de-
veloping the proposed system, this method demon-
strates a potential for use in helping to answer more
specialized questions. Examples may include intra-
material classification to provide evidence for differ-
ing production techniques and/or manuscript dating.
The consequences of gaining such insight by substi-
tuting in the proposed technique are threefold; the
preservation of delicate ancient manuscripts from fur-
ther degradation, a relatively low cost uncomplicated
implementable method, and an additional extendable
tool in gathering evidence to help conclude the ques-
tions surrounding the production of such manuscripts.
ACKNOWLEDGMENT
The authors like to thank Mladen Popovi
´
c, PI of
the European Research Council (EU Horizon 2020)
project: The Hands that Wrote the Bible: Digital
Palaeography and Scribal Culture of the Dead Sea
Scrolls (HandsandBible 640497), who allowed work
with the data and provided valuable inputs and the la-
bels for the materials. Finally, for the high-resolution
images of the Dead Sea Scrolls, we are grateful to
the Israel Antiquities Authority (IAA), courtesy of the
Leon Levy DSS Digital Library; photographer: Shai
Halevi.
REFERENCES
Abitbol, R. and Shimshoni, I. (2021). Machine Learning
Based Assembly of Fragments of Ancient Papyrus.
ACM Journal on Computing and Cultural Heritage,
14(3).
Bajcsy, R. (1973). Computer description of textured sur-
faces. Ijcai, pages 572–579.
Bell, S., Upchurch, P., Snavely, N., and Bala, K. (2015).
Material recognition in the wild with the Materials in
Context Database. Proceedings of the IEEE Computer
Society Conference on Computer Vision and Pattern
Recognition, 07-12-June:3479–3487.
Bharati, M. H., Liu, J. J., and MacGregor, J. F. (2004).
Image texture analysis: Methods and comparisons.
Chemometrics and Intelligent Laboratory Systems,
72(1):57–71.
Camargo, A. and Smith, J. S. (2009). Image pattern classifi-
cation for the identification of disease causing agents
in plants. Computers and Electronics in Agriculture,
66(2):121–125.
Cevikalp, H. and Kurt, Z. (2017). the Fourier Transform
Based Descriptor for Visual Object Classification.
Anadolu University journal of Science and Technol-
ogy - Applied Sciences and Engineering, 18(1):247–
247.
Criminisi, A., P
´
erez, P., and Toyama, K. (2004). Region
filling and object removal by exemplar-based image
inpainting. IEEE Transactions on Image Processing,
13(9):1200–1212.
Dhali, M. A., de Wit, J. W., and Schomaker, L. (2019).
BiNet: Degraded-Manuscript Binarization in Diverse
Document Textures and Layouts using Deep Encoder-
Decoder Networks. arXiv.
Dhali, M. A., He, S., Popovi
´
c, M., Tigchelaar, E., and
Schomaker, L. (2017). A digital palaeographic ap-
proach towards writer identification in the dead sea
scrolls. ICPRAM 2017 - Proceedings of the 6th In-
ternational Conference on Pattern Recognition Appli-
cations and Methods, 2017-Janua(Icpram):693–702.
Dhali, M. A., Jansen, C. N., de Wit, J. W., and Schomaker,
L. (2020). Feature-extraction methods for histori-
cal manuscript dating based on writing style develop-
ment. Pattern Recognition Letters, 131:413–420.
Franzen, F. and Yuan, C. (2019). Visualizing image clas-
sification in fourier domain. ESANN 2019 - Proceed-
ings, 27th European Symposium on Artificial Neural
Networks, Computational Intelligence and Machine
Learning, 27(April):535–540.
Freedman, J., van Dorp, L., and Brace, S. (2018). Destruc-
tive sampling natural science collections: an overview
for museum professionals and researchers. Journal of
Natural Science Collections, 5:21–34.
Hassner, M. and Sklansky, J. (1980). The use of Markov
Random Fields as models of texture. Computer
Graphics and Image Processing, 12(4):357–370.
Hu, X. and Ensor, A. (2019). Fourier Spectrum Image Tex-
ture Analysis. International Conference Image and
Vision Computing New Zealand, 2018-Novem(1):1–6.
Hui, S. and Zak, S. H. (2014). Discrete Fourier trans-
form based pattern classifiers. Bulletin of the Polish
Academy of Sciences: Technical Sciences, 62(1):15–
22.
Kalliatakis, G., Stamatiadis, G., Ehsan, S., Leonardis, A.,
Gall, J., Sticlaru, A., and McDonald-Maier, K. D.
(2017). Evaluating deep convolutional neural net-
works for material classification. arXiv, 2.
Kliangsuwan, T. and Heednacram, A. (2018). FFT features
and hierarchical classification algorithms for cloud
images. Engineering Applications of Artificial Intel-
ligence, 76(May 2016):40–54.
Kumar, Y., Jajoo, G., and Yadav, S. K. (2020). 2d-fft based
modulation classification using deep convolution neu-
ral network. In 2020 IEEE 17th India Council Inter-
national Conference (INDICON), pages 1–6. IEEE.
Loll, C., Quandt, A., Mass, J., Kupiec, T., Pollak, R., and
Shugar, A. (2019). Museum of the Bible Dead Sea
ICPRAM 2023 - 12th International Conference on Pattern Recognition Applications and Methods
704