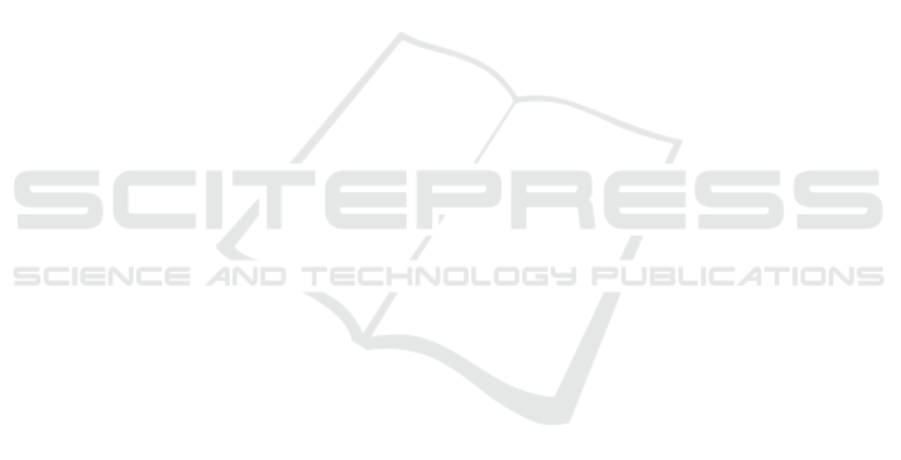
While the existing method failed to correctly cap-
ture the risk regions, the proposed method was able to
identify the risk regions accurately, so the proposed
method improved the accuracy of RMSE and other
metrics. From the values of Precision and Recall, we
also find that the risks were estimated more accurately
by the proposed method for regions where objects are
present.
6 CONCLUSION
In this paper, we proposed a method for estimating
accident risk maps, which represent the accident risk
to the own vehicle, based on in-vehicle images.
The dataset required for training the GAN was
created using a model independent of the camera pa-
rameters. Unlike the conventional Time-to-Contact,
the dataset created by the proposed method can rep-
resent with high accuracy the greater risk only for ob-
jects approaching in the direction of the own vehicle.
Moreover, by combining the trained UString-Net, it is
possible to create a dataset of accident risk maps that
represent only hazards in situations where accidents
are likely to occur.
We also proposed a network for generating the
risk map images from in-vehicle images. The pro-
posed network trained by the proposed dataset can
estimate the accident risk map more accurately than
the conventional network by dealing with scenes with
different camera parameters.
Finally, we confirmed through real-world experi-
ments that the proposed method can visualize the risk
to the own vehicle using any type of in-vehicle cam-
era.
REFERENCES
Bao, W., Yu, Q., and Kong, Y. (2020). Uncertainty-based
traffic accident anticipation with spatio-temporal rela-
tional learning. In Proc. ACM International Confer-
ence on Multimedia.
Chan, F.-H., Chen, Y.-T., Xiang, Y., and Sun, M. (2016).
Anticipating accidents in dashcam videos. In Proc.
Asian Conference on Computer Vision.
Corcoran, G.-P. and Clark, J. (2019). Traffic risk as-
sessment: A two-stream approach using dynamic-
attention. In Proc. Conference on Computer and
Robot Vision (CRV), pages 166–173.
Cordts, M., Omran, M., Ramos, S., Rehfeld, T., Enzweiler,
M., Benenson, R., Franke, U., Roth, S., and Schiele,
B. (2016). The cityscapes dataset for semantic urban
scene understanding.
Hassanein, A. S., Mohammad, S., Sameer, M., and Ragab,
M. E. (2015). A survey on hough transform, theory,
techniques and applications. arXiv.
He, K., Gkioxari, G., Doll
´
ar, P., and Girshick, R. (2017).
Mask R-CNN. In Proc. International Conference on
Computer Vision (ICCV).
Hou, Y., Ma, Z., Liu, C., and Loy, C. C. (2019). Learning
lightweight lane detection cnns by self attention dis-
tillation. In Proc. International Conference on Com-
puter Vision.
Ilg, E., Mayer, N., Saikia, T., Keuper, M., Dosovitskiy, A.,
and Brox, T. (2017). Flownet 2.0: Evolution of opti-
cal flow estimation with deep networks. In Proc. Con-
ference on Computer Vision and Pattern Recognition
(CVPR).
Ke, L., Li, X., Danelljan, M., Tai, Y.-W., Tang, C.-K.,
and Yu, F. (2021). Prototypical cross-attention net-
works for multiple object tracking and segmentation.
In Proc. Advances in Neural Information Processing
Systems.
Suzuki, T., Kataoka, H., Aoki, Y., and Satoh, Y. (2018).
Anticipating traffic accidents with adaptive loss and
large-scale incident db. In Proc. Conference on Com-
puter Vision and Pattern Recognition (CVPR).
Tresilian, J. (1991). Empirical and theoretical issues in the
perception of time to contact. Journal of Experimen-
tal Psychology: Human Perception and Performance,
17(3):865–876.
Voigtlaender, P., Krause, M., Osep, A., Luiten, J., Sekar, B.
B. G., Geiger, A., and Leibe, B. (2019). MOTS: Multi-
object tracking and segmentation. In Proc. Conference
on Computer Vision and Pattern Recognition (CVPR).
Vu, D., Ngo, B., and Phan, H. (2022). Hybridnets: End-to-
end perception network. arXiv.
Wang, T.-C., Liu, M.-Y., Zhu, J.-Y., Liu, G., Tao, A., Kautz,
J., and Catanzaro, B. (2018a). Video-to-video synthe-
sis. In Proc. Conference on Neural Information Pro-
cessing Systems (NeurIPS).
Wang, Z., Ren, W., and Qiu, Q. (2018b). LaneNet: Real-
time lane detection networks for autonomous driving.
arXiv.
Yao, Y., Xu, M., Wang, Y., Crandall, D. J., and Atkins,
E. M. (2019). Unsupervised traffic accident detection
in first-person videos. In Proc. International Confer-
ence on Intelligent Robots and Systems (IROS).
Yu, F., Chen, H., Wang, X., Xian, W., Chen, Y., Liu, F.,
Madhavan, V., and Darrell, T. (2018). Bdd100k: A
diverse driving dataset for heterogeneous multitask
learning. In Proc. Conference on Computer Vision and
Pattern Recognition.
Zeng, K.-H., Chou, S.-H., Chan, F.-H., Niebles, J. C., and
Sun, M. (2017). Agent-centric risk assessment: Ac-
cident anticipation and risky region localization. In
Proc. Conference on Computer Vision and Pattern
Recognition (CVPR).
Seeing Risk of Accident from In-Vehicle Cameras
679