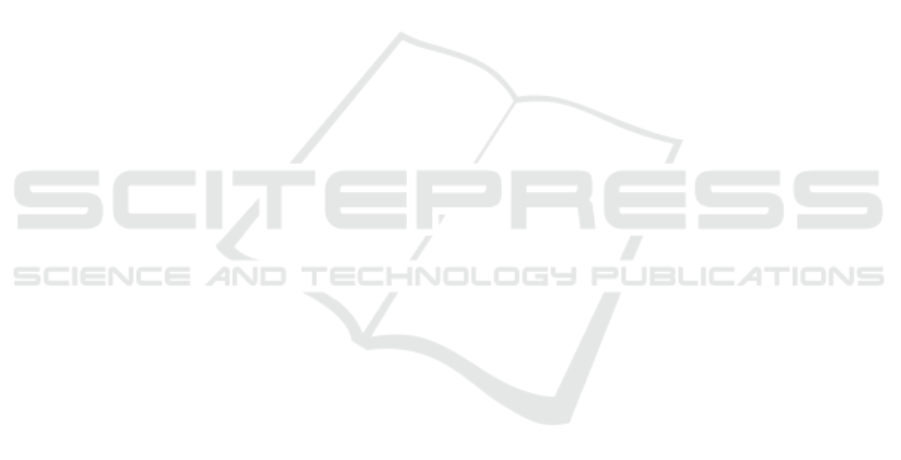
position determination, the movement detection, the
embedded triage guidance together with the user in-
terface of the bracelet as well as the central situation
overview still have to be designed.
The integration of machine learning to forecast
possible triage status changes is an interesting re-
search topic. In both prehospital and clinical settings
data based triage models show promising results. A
prehospital triage forecasting model based on time
series data can only be developed after it was deter-
mined which vital data can be measured continuously
during a prehospital triage.
The effectiveness of an electronic triage system
can only be validated with emergency drills or even
better with real emergency situations. Especially
our assumption that first responders without spe-
cific triage training are enabled to perform a semi-
automated prehospital triage has to be verified this
way. Additionally, in the same manner, the validity
of the proposed blood pressure measurement method
must be confirmed.
REFERENCES
Bußmann, A. B., Durasiewicz, C. P., Kibler, S. H. A., and
Wald, C. K. (2021a). Piezoelectric titanium based
microfluidic pump and valves for implantable medi-
cal applications. Sensors and Actuators A: Physical,
323:112649.
Bußmann, A. B., Gr
¨
unerbel, L. M., Durasiewicz, C. P.,
Thalhofer, T. A., Wille, A., and Richter, M. (2021b).
Microdosing for drug delivery application—a review.
Sensors and Actuators A: Physical, 330:112820.
EMFT Steel Pumps. Stainless steel micropumps for
medical-, laboratory and analysis technology.
https://www.emft.fraunhofer.de/content/dam/emft/
emft-neu/deutsch/dokumente/infoblatt-stainless-
steel-micropumps-medical-laboratory-analysis-
technology-fraunhofer-emft.pdf. Accessed: 2023-01-
02.
Gao, T., Massey, T., Bishop, W., Bernstein, D., Selavo,
L., Alm, A., White, D., and Sarrafzadeh, M. (2006).
Integration of triage and biomedical devices for con-
tinuous, real-time, automated patient monitoring. In
2006 3rd IEEE/EMBS International Summer School
on Medical Devices and Biosensors, pages 34–39.
Gao, T., Massey, T., Selavo, L., Crawford, D., Chen, B.-
r., Lorincz, K., Shnayder, V., Hauenstein, L., Dabiri,
F., Jeng, J., Chanmugam, A., White, D., Sarrafzadeh,
M., and Welsh, M. (2007). The advanced health and
disaster aid network: A light-weight wireless medical
system for triage. IEEE Transactions on Biomedical
Circuits and Systems, 1(3):203–216.
Gruenerbel, L. (2022). Experimental and theoretical
methodologies for high-flow optimization of microp-
umps in medical applications. Dissertation (not pub-
lished yet), page 165.
Johnson, A., Bulgarelli, L., Pollard, T., Horng, S., Celi,
L. A., and Mark, R. (2022). Mimic-iv. https://
physionet.org/content/mimiciv/2.0/. Accessed: 2022-
11-16.
Lenert, L. A., Kirsh, D., Griswold, W. G., Buono, C., Lyon,
J., Rao, R., and Chan, T. C. (2011). Design and eval-
uation of a wireless electronic health records system
for field care in mass casualty settings. Journal of the
American Medical Informatics Association : JAMIA,
18(6):842–852.
Levin, S., Toerper, M., Hamrock, E., Hinson, J. S., Barnes,
S., Gardner, H., Dugas, A., Linton, B., Kirsch, T., and
Kelen, G. (2018). Machine-learning-based electronic
triage more accurately differentiates patients with re-
spect to clinical outcomes compared with the emer-
gency severity index. Annals of Emergency Medicine,
71(5):565–574.e2.
Lorincz, K., Malan, D. J., Fulford-Jones, T., Nawoj, A.,
Clavel, A., Shnayder, V., Mainland, G., Welsh, M.,
and Moulton, S. (2004). Sensor networks for emer-
gency response: Challenges and opportunities. IEEE
Pervasive Computing, 3(4):16–23.
MIMIC. The medical information mart for intensive care.
https://mimic.mit.edu/. Accessed: 2022-11-16.
Park, J. Y. (2021). Real-time monitoring electronic
triage tag system for improving survival rate in
disaster-induced mass casualty incidents. Healthcare,
9(7):877.
Paul, A. O., Kay, M. V., Huppertz, T., Mair, F., Dierking,
Y., Hornburger, P., Mutschler, W., and Kanz, K.-G.
(2009). Validierung der vorsichtung nach dem mstart-
algorithmus: Pilotstudie zur entwicklung einer multi-
zentrischen evaluation. Der Unfallchirurg, 112(1):23–
30, 32.
Petrie, J. C., O’Brien, E. T., Littler, W. A., and de Swiet,
M. (1986). Recommendations on blood pressure mea-
surement. Br Med J (Clin Res Ed), 293(6547):611–
615.
Ramsey, M. (1979). Noninvasive automatic determination
of mean arterial pressure. Medical & biological engi-
neering & computing, 17(1):11–18.
RescueWave (2022). Digitale sichtungskarte f
¨
ur manv.
https://rescuewave.de/. Accessed: 2022-06-29.
Rodriguez, D., Heuer, S., Guerra, A., Stork, W., Weber, B.,
and Eichler, M. (2014). Towards automatic sensor-
based triage for individual remote monitoring during
mass casualty incidents. undefined.
Sakanushi, K., Hieda, T., Shiraishi, T., Ode, Y., Takeuchi,
Y., Imai, M., Higashino, T., and Tanaka, H. (2013).
Electronic triage system for continuously monitoring
casualties at disaster scenes. Journal of Ambient Intel-
ligence and Humanized Computing, 4(5):547–558.
Schm
¨
oller, G. and Hagen, F. (2017). Sichtung und vorsich-
tung bei massenanfall von verletzten und erkrankten.
retten!, 6(05):372–381.
Sharman, J. E., Tan, I., Stergiou, G. S., Lombardi, C., Sala-
dini, F., Butlin, M., Padwal, R., Asayama, K., Avolio,
A., Brady, T. M., Murray, A., and Parati, G. (2022).
Automated ’oscillometric’ blood pressure measuring
Towards an Intelligent Triage Bracelet: A Conceptual Study of a Semi-Automated Prehospital Triage Algorithm and the Integration of
Blood Pressure Measurement
175