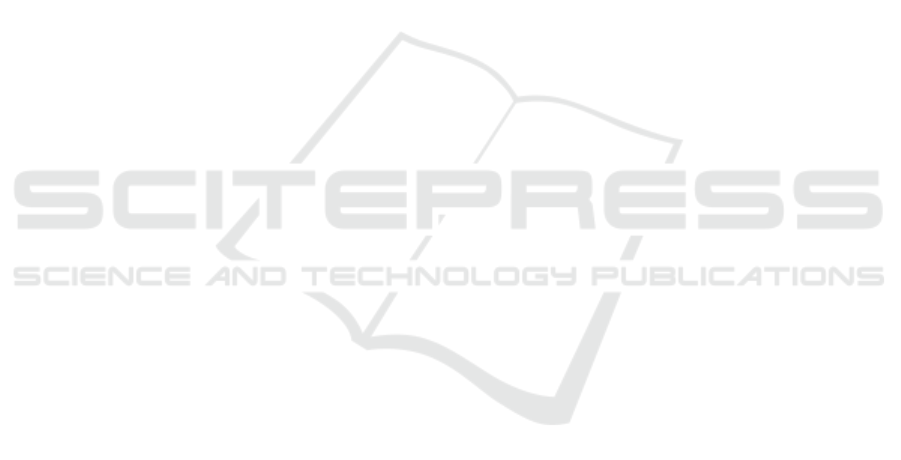
chines by vibration or current signals: A critical com-
parison. In 2008 IEEE Industry Applications Society
Annual Meeting, pages 1–8.
Caggiano, A., Zhang, J., Alfieri, V., Caiazzo, F., Gao, R.,
and Teti, R. (2019). Machine learning-based image
processing for on-line defect recognition in additive
manufacturing. CIRP Annals, 68(1):451–454.
Chawla, N. V., Bowyer, K. W., Hall, L. O., and Kegelmeyer,
W. P. (2002). Smote: synthetic minority over-
sampling technique. Journal of artificial intelligence
research, 16:321–357.
Greydanus, S. J., Dzumba, M., and Yosinski, J. (2019).
Hamiltonian neural networks. In 33rd Conference
on Neural Information Processing Systems (NeurIPS),
Vancouver, Canada.
Jiang, Q., Jia, M., Hu, J., and Xu, F. (2009). Machinery fault
diagnosis using supervised manifold learning. Me-
chanical systems and signal processing, 23(7):2301–
2311.
Karniadakis, G. E., Kevrekidis, I. G., Lu, L., Perdikaris,
P., Wang, S., and Yang, L. (2021). Physics-informed
machine learning. Nature Reviews Physics, 3(6):422–
440.
Lei, Y., He, Z., and Zi, Y. (2008). A new approach to in-
telligent fault diagnosis of rotating machinery. Expert
Systems with applications, 35(4):1593–1600.
Li, C., S
˜
A¡nchez, R.-V., Zurita, G., Cerrada, M., and Cabr-
era, D. (2016). Fault diagnosis for rotating machinery
using vibration measurement deep statistical feature
learning. Sensors, 16(6).
Li, P., Kong, F., He, Q., and Liu, Y. (2013). Multiscale slope
feature extraction for rotating machinery fault diagno-
sis using wavelet analysis. Measurement, 46(1):497–
505.
Marins, M. A., Ribeiro, F. M., Netto, S. L., and da Silva,
E. A. (2018). Improved similarity-based modeling for
the classification of rotating-machine failures. Journal
of the Franklin Institute, 355(4):1913–1930. Special
Issue on Recent advances in machine learning for sig-
nal analysis and processing.
Nayana, B. R. and Geethanjali, P. (2017). Analysis of sta-
tistical time-domain features effectiveness in identifi-
cation of bearing faults from vibration signal. IEEE
Sensors Journal, 17(17):5618–5625.
Ribeiro, F., Marins, M., Netto, S., and da Silva, E. (2017).
Rotating machinery fault diagnosis using similarity-
based models. In XXXV Simp
´
osio Brasileiro de
Telecomunicac¸
˜
oes e Processamento de Sinais, S
˜
ao Pe-
dro, Brasil.
Rizzo, P. and di Scalea, F. L. (2006). Feature extraction for
defect detection in strands by guided ultrasonic waves.
Structural Health Monitoring, 5(3):297–308.
Seshadrinath, J., Singh, B., and Panigrahi, B. K. (2014).
Investigation of vibration signatures for multiple fault
diagnosis in variable frequency drives using complex
wavelets. IEEE Transactions on Power Electronics,
29(2):936–945.
Usamentiaga, R., Venegas, P., Guerediaga, J., Vega, L., and
L
´
opez, I. (2013). Feature extraction and analysis for
automatic characterization of impact damage in car-
bon fiber composites using active thermography. NDT
& E International, 54:123–132.
Van, B., Van Hoa, N., Nguyen, H., and Jang, Y. M. (2020).
Statistical feature extraction in machine fault detec-
tion using vibration signal. In International Confer-
ence on Information and Communication Technology
Convergence (ICTC), pages 666–669.
Wei, J., Chu, X., Sun, X.-Y., Xu, K., Deng, H.-X., Chen,
J., Wei, Z., and Lei, M. (2019). Machine learning in
materials science. InfoMat, 1(3):338–358.
Yen, G. and Lin, K.-C. (2000). Wavelet packet feature ex-
traction for vibration monitoring. IEEE Transactions
on Industrial Electronics, 47(3):650–667.
Yin, L., Ye, B., Zhang, Z., Tao, Y., Xu, H., Salas Avila,
J. R., and Yin, W. (2019). A novel feature extraction
method of eddy current testing for defect detection
based on machine learning. NDT & E International,
107:102108.
ICPRAM 2023 - 12th International Conference on Pattern Recognition Applications and Methods
480