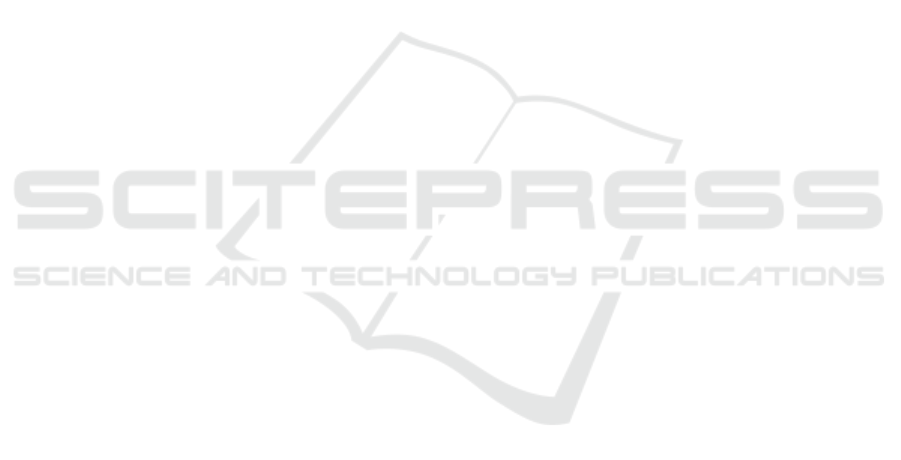
This observation demonstrates the benefit of the use
of both image and text masks as input in combination
with attention mechanism.
To measure the competitive results of our pro-
posed approach, we compare them with other saliency
prediction alternatives, establishing a benchmark for
eye-gaze prediction task. In our comparison, we
found state-of-the-art results of our model with high
scores across quantitative metrics, and lower false
positive rates than other approaches. Visual analy-
sis further confirms our findings, that our approach
produces more accurate prediction of eye-gaze loca-
tions on relevant website locations, including where
text and image are present. The result suggests the su-
periority of our approach in capturing user behavior.
Future work will be to incorporate other user behav-
ior characteristics (e.g. mouse trajectory), including
users’ identity (if accessible) such as age, and loca-
tions as additional modalities to further benefit from
this information to improve prediction accuracy.
ACKNOWLEDGEMENT
This work is funded by UDeco project by Germany
BMBF-KMU Innovativ - 01IS20030B.
REFERENCES
Aspandi, D., Doosdal, S.,
¨
Ulger, V., Gillich, L., and
Staab, S. (2022a). User interaction analysis through
contrasting websites experience. arXiv preprint
arXiv:2201.03638.
Aspandi, D., Mallol-Ragolta, A., Schuller, B., and Binefa,
X. (2020). Latent-based adversarial neural networks
for facial affect estimations. In 2020 15th IEEE Inter-
national Conference on Automatic Face and Gesture
Recognition (FG 2020), pages 606–610. IEEE.
Aspandi, D., Sukno, F., Schuller, B. W., and Binefa, X.
(2022b). Audio-visual gated-sequenced neural net-
works for affect recognition. IEEE Transactions on
Affective Computing.
Bylinskii, Z., Judd, T., Oliva, A., Torralba, A., and Durand,
F. (2018). What do different evaluation metrics tell us
about saliency models? IEEE transactions on pattern
analysis and machine intelligence, 41(3):740–757.
Chen, L.-C., Papandreou, G., Kokkinos, I., Murphy, K., and
Yuille, A. L. (2017). Deeplab: Semantic image seg-
mentation with deep convolutional nets, atrous convo-
lution, and fully connected crfs. IEEE transactions on
pattern analysis and machine intelligence, 40(4):834–
848.
Dai, Y., Gieseke, F., Oehmcke, S., Wu, Y., and Barnard, K.
(2021). Attentional feature fusion. In Proceedings of
the IEEE/CVF Winter Conference on Applications of
Computer Vision, pages 3560–3569.
Goferman, S., Zelnik-Manor, L., and Tal, A. (2011).
Context-aware saliency detection. IEEE transac-
tions on pattern analysis and machine intelligence,
34(10):1915–1926.
Hou, X. and Zhang, L. (2007). Saliency detection: A spec-
tral residual approach. In 2007 IEEE Conference on
computer vision and pattern recognition, pages 1–8.
Ieee.
Hou, X. and Zhang, L. (2008). Dynamic visual attention:
Searching for coding length increments. Advances in
neural information processing systems, 21.
Houx, D., HAREL, J., and KOCH, C. (2012). Image signa-
ture: highlighting sparse salient regions. IEEE Trans-
actions on Pattern Analysis and Machine Intelligence,
34(1):194–201.
Jetley, S., Murray, N., and Vig, E. (2016). End-to-end
saliency mapping via probability distribution predic-
tion. In Proceedings of the IEEE conference on com-
puter vision and pattern recognition, pages 5753–
5761.
Jiang, M., Huang, S., Duan, J., and Zhao, Q. (2015). Sali-
con: Saliency in context. In Proceedings of the IEEE
conference on computer vision and pattern recogni-
tion, pages 1072–1080.
Judd, T., Ehinger, K., Durand, F., and Torralba, A. (2009).
Learning to predict where humans look. In 2009
IEEE 12th international conference on computer vi-
sion, pages 2106–2113. IEEE.
Kingma, D. P. and Ba, J. (2014). Adam: A
method for stochastic optimization. arXiv preprint
arXiv:1412.6980.
Kroner, A., Senden, M., Driessens, K., and Goebel, R.
(2020). Contextual encoder–decoder network for vi-
sual saliency prediction. Neural Networks, 129:261–
270.
Le Meur, O., Le Callet, P., and Barba, D. (2007). Predict-
ing visual fixations on video based on low-level visual
features. Vision research, 47(19):2483–2498.
Li, J., Levine, M. D., An, X., Xu, X., and He, H. (2012). Vi-
sual saliency based on scale-space analysis in the fre-
quency domain. IEEE transactions on pattern analy-
sis and machine intelligence, 35(4):996–1010.
Menges, R. (2020). Gazemining: A dataset of video and
interaction recordings on dynamic web pages. labels
of visual change, segmentation of videos into stimulus
shots, and discovery of visual stimuli.
Pan, S. J. and Yang, Q. (2010). A survey on transfer learn-
ing. IEEE Transactions on Knowledge and Data En-
gineering, 22(10):1345–1359.
Peters, R. J., Iyer, A., Itti, L., and Koch, C. (2005). Compo-
nents of bottom-up gaze allocation in natural images.
Vision Research, 45(18):2397–2416.
Riche, N., Mancas, M., Gosselin, B., and Dutoit, T. (2012).
Rare: A new bottom-up saliency model. In 2012 19th
IEEE International Conference on Image Processing,
pages 641–644. IEEE.
Rodriguez-Diaz, N., Aspandi, D., Sukno, F. M., and Binefa,
X. (2021). Machine learning-based lie detector ap-
plied to a novel annotated game dataset. Future Inter-
net, 14(1):2.
Predicting Eye Gaze Location on Websites
131