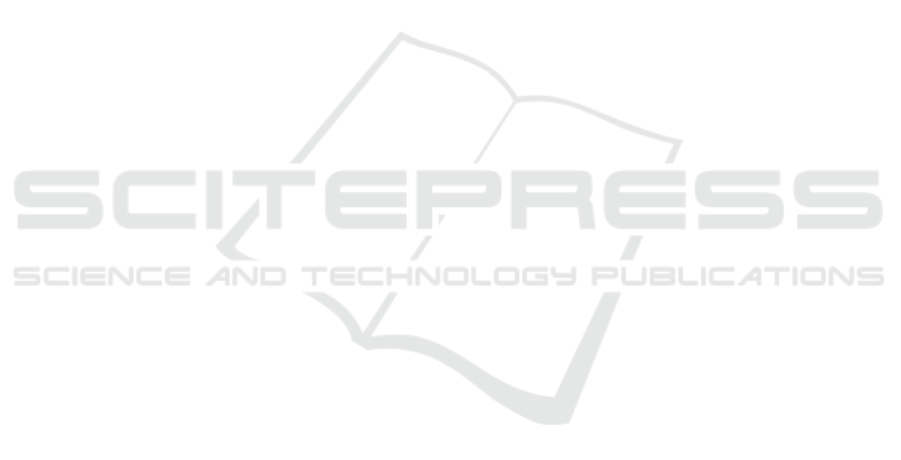
Janssen, M., Charalabidis, Y., and Zuiderwijk, A. (2012).
Benefits, adoption barriers and myths of open data and
open government. Information systems management,
29(4):258–268.
Kurada, R. R., Ramu, Y., and Pattem, S. (2021). Lessoning
geospatial visualizations on real-time data. In 2021
IEEE International Conference on Computation Sys-
tem and Information Technology for Sustainable So-
lutions (CSITSS), pages 1–6.
Mart
´
ın-Mart
´
ın, A., Orduna-Malea, E., Thelwall, M., and
L
´
opez-C
´
ozar, E. D. (2018). Google scholar, web of
science, and scopus: A systematic comparison of ci-
tations in 252 subject categories. Journal of informet-
rics, 12(4):1160–1177.
Mongeon, P. and Paul-Hus, A. (2016). The journal coverage
of web of science and scopus: a comparative analysis.
Scientometrics, 106:213–228.
Morelli, N., Mulder, I., Pedersen, J. S., Jaskiewicz, T.,
G
¨
otzen, A. d., et al. (2017). Open data as a new com-
mons. empowering citizens to make meaningful use
of a new resource. In International Conference on In-
ternet Science, pages 212–221.
Obie, H. O., Chua, C., Avazpour, I., Abdelrazek, M.,
Grundy, J., and Bednarz, T. (2020). Authoring logi-
cally sequenced visual data stories with gravity. Jour-
nal of Computer Languages, 58:100961.
Open Knowledge Foundation (2013). Defining open data.
https://blog.okfn.org/2013/10/03/defining-open-data,
[Online, Last access November 2022].
Piedra, N., Chicaiza, J., L
´
opez, J., and Caro, E. T. (2016).
Integrating oer in the design of educational mate-
rial: Blended learning and linked-open-educational-
resources-data approach. In Global Engineering Edu-
cation Conference, pages 1179–1187.
Piedra, N., Chicaiza, J., L
´
opez, J., and Caro, E. T. (2017). A
rating system that open-data repositories must satisfy
to be considered oer: Reusing open data resources in
teaching. In Global Engineering Education Confer-
ence, pages 1768–1777.
Renuka, T., Chitra, C., Pranesha, T., G., D., and M., S.
(2017). Open data usage by undergraduate students.
In 5th IEEE International Conference on MOOCs, In-
novation and Technology in Education, pages 46–51.
Rubin, A. (2021). What to consider when we consider data.
Teaching Statistics, 43(S1):S23–S33.
Ruijer, E., Grimmelikhuijsen, S., van den Berg, J., and Mei-
jer, A. (2020). Open data work: understanding open
data usage from a practice lens. International Review
of Administrative Sciences, 86(1):3–19.
Saddiqa, M., Kirikova, M., Magnussen, R., Larsen, B., and
Pedersen, J. M. (2019a). Enterprise architecture ori-
ented requirements engineering for the design of a
school friendly open data web interface. Complex Sys-
tems Informatics and Modeling Quarterly, (21):1–20.
Saddiqa, M., Larsen, B., Magnussen, R., Rasmussen, L. L.,
and Pedersen, J. M. (2019b). Open data visualization
in danish schools: A case study. In Proc. of Intern.
Conf. in Central Europe on Computer Graphics, Visu-
alization and Computer Vision.
Saddiqa, M., Magnussen, R., Larsen, B., and Pedersen,
J. M. (2021). Open data interface (odi) for sec-
ondary school education. Computers & Education,
174:104294.
Saddiqa, M., Rasmussen, L., Magnussen, R., Larsen, B.,
and Pedersen, J. M. (2019c). Bringing open data
into danish schools and its potential impact on school
pupils. In Proc. of the 15th International Symposium
on Open Collaboration.
Seymoens, T., Van Audenhove, L., Van den Broeck, W.,
and Mari
¨
en, I. (2020). Data literacy on the road:
Setting up a large-scale data literacy initiative in the
databuzz project. Journal of Media Literacy Educa-
tion, 12(3):102–119.
Shamash, K., Alperin, J. P., and Bordini, A. (2015). Teach-
ing data analysis in the social sciences: A case study
with article level metrics. Open Data as Open Educa-
tional Resources, page 49.
Susha, I., Gr
¨
onlund,
˚
A., and Janssen, M. (2015). Organi-
zational measures to stimulate user engagement with
open data. Transforming Government: People, Pro-
cess and Policy.
Tovar, E. and Piedra, N. (2014). Guest editorial: open
educational resources in engineering education: var-
ious perspectives opening the education of engineers.
IEEE Transactions on Education, 57(4):213–219.
Trentini, A. and Scaravati, S. (2020). Raising curiosity
about open data via the ’physiradio’ musicalization iot
device. Data Science Journal, 19:39.
Van Audenhove, L., Van den Broeck, W., and Mari
¨
en, I.
(2020). Data literacy and education: Introduction and
the challenges for our field. Journal of Media Literacy
Education, 3:1–5.
Vargianniti, I. and Karpouzis, K. (2020). Using big and
open data to generate content for an educational game
to increase student performance and interest. Big Data
and Cognitive Computing, 4(4).
Watson, J. (2017). Open data in australian schools: Taking
statistical literacy and the practice of statistics across
the curriculum. In Data visualization and statistical
literacy for open and big data, pages 29–54.
Weishart, J. E. (2020). Democratizing education rights.
William & Mary Bill of Rights Journal, 29:1.
Windhager, F., Mayr, E., Schreder, G., and Smuc, M.
(2016). Linked information visualization for linked
open government data. a visual synthetics approach
to governmental data and knowledge collections.
JeDEM-eJournal of eDemocracy and Open Govern-
ment, 8(2):87–116.
Wolff, A., Wermelinger, M., and Petre, M. (2019). Explor-
ing design principles for data literacy activities to sup-
port children’s inquiries from complex data. Interna-
tional Journal of Human-Computer Studies, 129:41–
54.
´
Alvarez Otero, J., L
´
azaro, M., and JesusG, M. (2018). A
cloud-based giscience learning approach to spanish
national parks. European Journal of Geography, 9:6–
20.
At School of Open Data: A Literature Review
183