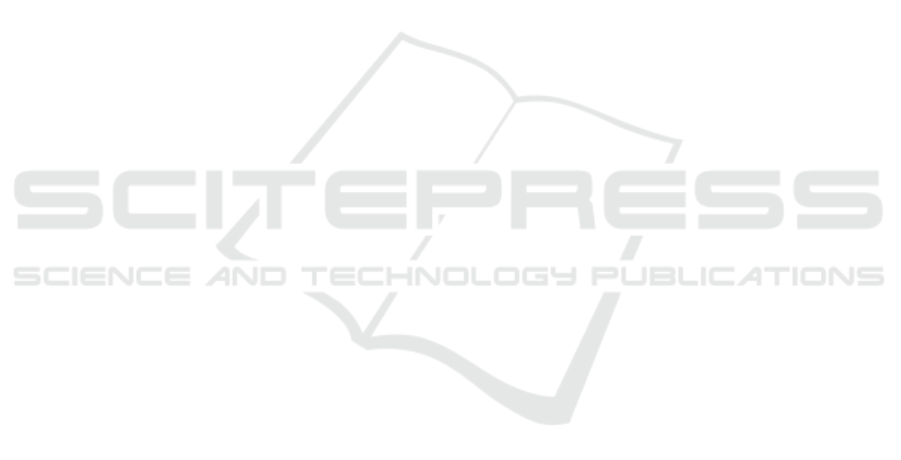
construction using transformers. Neural Information
Processing Systems. 2
Chabra, R., Lenssen, J. E., Ilg, E., Schmidt, T., Straub, J.,
Lovegrove, S., and Newcombe, R. (2020). Deep local
shapes: Learning local sdf priors for detailed 3d re-
construction. European Conference on Computer Vi-
sion. 1, 3
Chen, X., Zhang, Q., Li, X., Chen, Y., Feng, Y., Wang, X.,
and Wang, J. (2021a). Hallucinated neural radiance
fields in the wild. Conference on Computer Vision and
Pattern Recognition. 2
Chen, Z. and Zhang, H. (2021). Neural marching cubes.
ACM Transactions on Graphics. 1
Chen, Z., Zhang, Y., Genova, K., Fanello, S. R., Bouaziz,
S., H
¨
ane, C., Du, R., Keskin, C., Funkhouser, T. A.,
and Tang, D. (2021b). Multiresolution deep implicit
functions for 3d shape representation. International
Conference on Computer Vision. 1
Dahnert, M., Hou, J., Nießner, M., and Dai, A. (2021).
Panoptic 3d scene reconstruction from a single rgb im-
age. Neural Information Processing Systems. 2, 3, 8
Dasgupta, S., Fang, K., Chen, K., and Savarese, S. (2016).
Delay: Robust spatial layout estimation for cluttered
indoor scenes. In Conference on Computer Vision and
Pattern Recognition, pages 616–624, Las Vegas, NV,
USA. IEEE. 2
Davies, T., Nowrouzezahrai, D., and Jacobson, A. (2020).
On the effectiveness of weight-encoded neural im-
plicit 3d shapes. Arxiv. 1
Denninger, M., Sundermeyer, M., Winkelbauer, D., Zidan,
Y., Olefir, D., Elbadrawy, M., Lodhi, A., and Katam,
H. (2019). Blenderproc. Arxiv. 8
Denninger, M. and Triebel, R. (2020). 3d scene reconstruc-
tion from a single viewport. In European Conference
on Computer Vision. 1, 2, 3, 4, 5, 6, 7, 8
Fu, H., Cai, B., Gao, L., Zhang, L., Li, J. W. C., Xun, Z.,
Sun, C., Jia, R., Zhao, B., and Zhang, H. (2020a). 3d-
front: 3d furnished rooms with layouts and semantics.
International Conference on Computer Vision. 8
Fu, H., Jia, R., Gao, L., Gong, M., Zhao, B., Maybank, S.,
and Tao, D. (2020b). 3d-future: 3d furniture shape
with texture. International Conference on Computer
Vision. 8
Gkioxari, G., Malik, J., and Johnson, J. (2019). Mesh r-cnn.
International Conference on Computer Vision. 2
He, K., Zhang, X., Ren, S., and Sun, J. (2016). Deep resid-
ual learning for image recognition. In Conference on
Computer Vision and Pattern Recognition, pages 770–
778. 5
Jiang, C. M., Sud, A., Makadia, A., Huang, J., Nießner, M.,
and Funkhouser, T. (2020). Local implicit grid rep-
resentations for 3d scenes. Conference on Computer
Vision and Pattern Recognition. 1
Kuo, W., Angelova, A., Lin, T.-Y., and Dai, A. (2020).
Mask2cad: 3d shape prediction by learning to seg-
ment and retrieve. European Conference on Computer
Vision. 2
Lorensen, W. E. and Cline, H. E. (1987). Marching
cubes: A high resolution 3d surface construction al-
gorithm. In Computer graphics and interactive tech-
niques, SIGGRAPH ’87, page 163–169, New York,
NY, USA. Association for Computing Machinery. 1
Martin-Brualla, R., Radwan, N., Sajjadi, M. S. M., Barron,
J. T., Dosovitskiy, A., and Duckworth, D. (2020). Nerf
in the wild: Neural radiance fields for unconstrained
photo collections. Conference on Computer Vision
and Pattern Recognition. 2
Mildenhall, B., Hedman, P., Martin-Brualla, R., Srinivasan,
P., and Barron, J. T. (2021). Nerf in the dark: High
dynamic range view synthesis from noisy raw images.
Conference on Computer Vision and Pattern Recogni-
tion. 2
Mildenhall, B., Srinivasan, P. P., Tancik, M., Barron, J. T.,
Ramamoorthi, R., and Ng, R. (2020). Nerf: Repre-
senting scenes as neural radiance fields for view syn-
thesis. Communications of the ACM. 2
Nie, Y., Han, X., Guo, S., Zheng, Y., Chang, J., and Zhang,
J. J. (2020). Total3dunderstanding: Joint layout, ob-
ject pose and mesh reconstruction for indoor scenes
from a single image. Conference on Computer Vision
and Pattern Recognition. 8
Oechsle, M., Peng, S., and Geiger, A. (2021). UNISURF:
unifying neural implicit surfaces and radiance fields
for multi-view reconstruction. International Confer-
ence on Computer Vision. 2
Ren, Y., Chen, C., Li, S., and Kuo, C. C. J. (2016). A coarse-
to-fine indoor layout estimation (cfile) method. Asian
Conference on Computer Vision. 2
Shin, D., Ren, Z., Sudderth, E. B., and Fowlkes, C. C.
(2019). 3d scene reconstruction with multi-layer depth
and epipolar transformers. International Conference
on Computer Vision. 2
Song, S., Yu, F., Zeng, A., Chang, A. X., Savva, M., and
Funkhouser, T. (2016). Semantic scene completion
from a single depth image. Conference on Computer
Vision and Pattern Recognition. 2
Straub, J., Whelan, T., Ma, L., Chen, Y., Wijmans, E.,
Green, S., Engel, J. J., Mur-Artal, R., Ren, C., Verma,
S., Clarkson, A., Yan, M., Budge, B., Yan, Y., Pan,
X., Yon, J., Zou, Y., Leon, K., Carter, N., Briales, J.,
Gillingham, T., Mueggler, E., Pesqueira, L., Savva,
M., Batra, D., Strasdat, H. M., Nardi, R. D., Goe-
sele, M., Lovegrove, S., and Newcombe, R. (2019).
The replica dataset: A digital replica of indoor spaces.
Arxiv. 8
Tancik, M., Srinivasan, P. P., Mildenhall, B., Fridovich-
Keil, S., Raghavan, N., Singhal, U., Ramamoorthi,
R., Barron, J. T., and Ng, R. (2020). Fourier features
let networks learn high frequency functions in low di-
mensional domains. Neural Information Processing
Systems. 4
Wang, P., Liu, L., Liu, Y., Theobalt, C., Komura, T., and
Wang, W. (2021). Neus: Learning neural implicit sur-
faces by volume rendering for multi-view reconstruc-
tion. Arxiv. 2
Xu, Q., Xu, Z., Philip, J., Bi, S., Shu, Z., Sunkavalli, K., and
Neumann, U. (2022). Point-nerf: Point-based neural
radiance fields. Conference on Computer Vision and
Pattern Recognition. 2
3D Semantic Scene Reconstruction from a Single Viewport
25