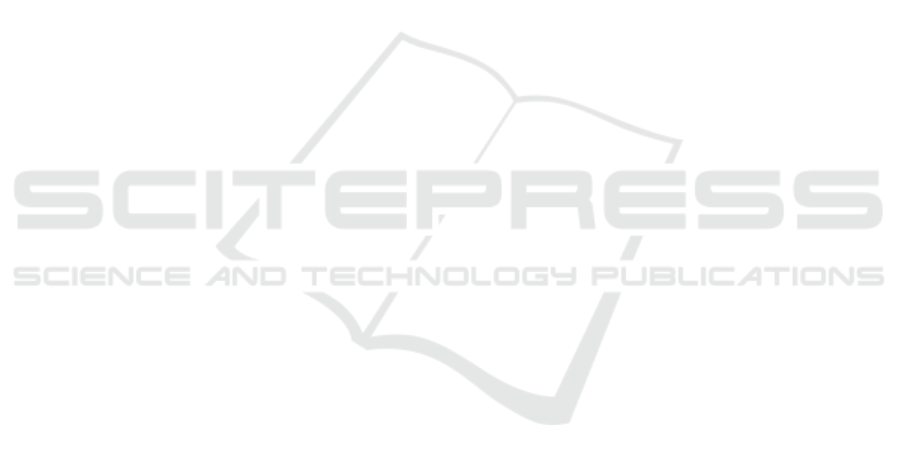
dataset: Multi-sensor infrastructure-based dataset for
mobility research. In 2022 IEEE Intelligent Vehicles
Symposium (IV), pages 965–970. IEEE.
Deng, S., Liang, Z., Sun, L., and Jia, K. (2022). Vista:
Boosting 3d object detection via dual cross-view spa-
tial attention. In Proceedings of the IEEE/CVF Con-
ference on Computer Vision and Pattern Recognition,
pages 8448–8457.
Everingham, M., Van Gool, L., Williams, C. K., Winn, J.,
and Zisserman, A. (2010). The pascal visual object
classes (voc) challenge. International journal of com-
puter vision, 88(2):303–338.
Fan, L., Pang, Z., Zhang, T., Wang, Y.-X., Zhao, H., Wang,
F., Wang, N., and Zhang, Z. (2022). Embracing single
stride 3d object detector with sparse transformer. In
Proceedings of the IEEE/CVF Conference on Com-
puter Vision and Pattern Recognition, pages 8458–
8468.
Geiger, A., Lenz, P., Stiller, C., and Urtasun, R. (2013).
Vision meets robotics: The kitti dataset. The Inter-
national Journal of Robotics Research, 32(11):1231–
1237.
Geiger, A., Lenz, P., and Urtasun, R. (2012). Are we ready
for autonomous driving? the kitti vision benchmark
suite. In 2012 IEEE conference on computer vision
and pattern recognition, pages 3354–3361. IEEE.
Geyer, J., Kassahun, Y., Mahmudi, M., Ricou, X., Durgesh,
R., Chung, A. S., Hauswald, L., Pham, V. H.,
M
¨
uhlegg, M., Dorn, S., et al. (2020). A2d2:
Audi autonomous driving dataset. arXiv preprint
arXiv:2004.06320.
Hartley, R. and Zisserman, A. (2003). Multiple view geom-
etry in computer vision. Cambridge university press.
He, C., Li, R., Li, S., and Zhang, L. (2022). Voxel set trans-
former: A set-to-set approach to 3d object detection
from point clouds. In Proceedings of the IEEE/CVF
Conference on Computer Vision and Pattern Recogni-
tion, pages 8417–8427.
Hu, Y., Ding, Z., Ge, R., Shao, W., Huang, L., Li, K., and
Liu, Q. (2022). Afdetv2: Rethinking the necessity
of the second stage for object detection from point
clouds. In Proceedings of the AAAI Conference on
Artificial Intelligence, volume 1, pages 969–979.
Huang, D., Chen, Y., Ding, Y., Liao, J., Liu, J., Wu, K., Nie,
Q., Liu, Y., and Wang, C. (2022). Rethinking dimen-
sionality reduction in grid-based 3d object detection.
arXiv preprint arXiv:2209.09464.
Huang, X., Wang, P., Cheng, X., Zhou, D., Geng, Q., and
Yang, R. (2019). The apolloscape open dataset for
autonomous driving and its application. IEEE trans-
actions on pattern analysis and machine intelligence,
42(10):2702–2719.
Jiao, Y., Jie, Z., Chen, S., Chen, J., Wei, X., Ma, L., and
Jiang, Y.-G. (2022). Msmdfusion: Fusing lidar and
camera at multiple scales with multi-depth seeds for
3d object detection. arXiv preprint arXiv:2209.03102.
Kesten, R., Usman, M., Houston, J., Pandya, T., Nad-
hamuni, K., Ferreira, A., Yuan, M., Low, B., Jain,
A., Ondruska, P., Omari, S., Shah, S., Kulkarni, A.,
Kazakova, A., Tao, C., Platinsky, L., Jiang, W., and
Shet, V. (2019). Level 5 perception dataset 2020.
https://level-5.global/level5/data/.
Ku, J., Mozifian, M., Lee, J., Harakeh, A., and Waslander,
S. L. (2018). Joint 3d proposal generation and object
detection from view aggregation. In 2018 IEEE/RSJ
International Conference on Intelligent Robots and
Systems (IROS), pages 1–8. IEEE.
Kumar, A., Brazil, G., and Liu, X. (2021). Groomed-
nms: Grouped mathematically differentiable nms for
monocular 3d object detection. In Proceedings of the
IEEE/CVF Conference on Computer Vision and Pat-
tern Recognition, pages 8973–8983.
Lang, A. H., Vora, S., Caesar, H., Zhou, L., Yang, J., and
Beijbom, O. (2019). Pointpillars: Fast encoders for
object detection from point clouds. In Proceedings
of the IEEE/CVF conference on computer vision and
pattern recognition, pages 12697–12705.
Li, Y., Chen, Y., Qi, X., Li, Z., Sun, J., and Jia, J.
(2022a). Unifying voxel-based representation with
transformer for 3d object detection. arXiv preprint
arXiv:2206.00630.
Li, Y., Yu, A. W., Meng, T., Caine, B., Ngiam, J., Peng,
D., Shen, J., Lu, Y., Zhou, D., Le, Q. V., et al.
(2022b). Deepfusion: Lidar-camera deep fusion for
multi-modal 3d object detection. In Proceedings of
the IEEE/CVF Conference on Computer Vision and
Pattern Recognition, pages 17182–17191.
Liang, T., Xie, H., Yu, K., Xia, Z., Lin, Z., Wang, Y., Tang,
T., Wang, B., and Tang, Z. (2022). Bevfusion: A sim-
ple and robust lidar-camera fusion framework. arXiv
preprint arXiv:2205.13790.
Liao, Y., Xie, J., and Geiger, A. (2022). Kitti-360: A novel
dataset and benchmarks for urban scene understand-
ing in 2d and 3d. IEEE Transactions on Pattern Anal-
ysis and Machine Intelligence.
Liu, Y., Wang, T., Zhang, X., and Sun, J. (2022a). Petr:
Position embedding transformation for multi-view 3d
object detection. arXiv preprint arXiv:2203.05625.
Liu, Y., Yan, J., Jia, F., Li, S., Gao, Q., Wang, T., Zhang,
X., and Sun, J. (2022b). Petrv2: A unified framework
for 3d perception from multi-camera images. arXiv
preprint arXiv:2206.01256.
Liu, Z., Tang, H., Amini, A., Yang, X., Mao, H., Rus, D.,
and Han, S. (2022c). Bevfusion: Multi-task multi-
sensor fusion with unified bird’s-eye view representa-
tion. arXiv preprint arXiv:2205.13542.
Luo, S., Dai, H., Shao, L., and Ding, Y. (2021). M3dssd:
Monocular 3d single stage object detector. In Pro-
ceedings of the IEEE/CVF Conference on Computer
Vision and Pattern Recognition, pages 6145–6154.
Mahmoud, A., Hu, J. S., and Waslander, S. L. (2022). Dense
voxel fusion for 3d object detection. arXiv preprint
arXiv:2203.00871.
Mao, J., Niu, M., Jiang, C., Liang, H., Chen, J., Liang, X.,
Li, Y., Ye, C., Zhang, W., Li, Z., et al. (2021). One
million scenes for autonomous driving: Once dataset.
arXiv preprint arXiv:2106.11037.
Mao, J., Shi, S., Wang, X., and Li, H. (2022). 3d object
detection for autonomous driving: A review and new
outlooks. arXiv preprint arXiv:2206.09474.
3D Object Detection for Autonomous Driving: A Practical Survey
71