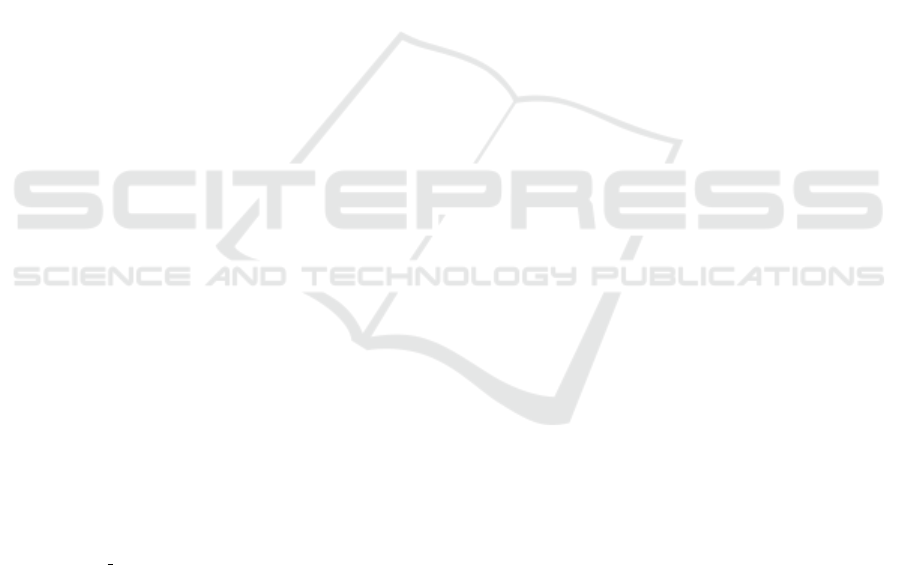
REFERENCES
Ahmad, Z., Shahid Khan, A., Nisar, K., Haider, I., Hassan,
R., Haque, M. R., Tarmizi, S., and Rodrigues, J. J.
(2021). Anomaly detection using deep neural network
for iot architecture. Applied Sciences, 11(15):7050.
Alani, M. M. (2014). TCP/IP Model, pages 19–50. Springer
International Publishing, Cham.
Alani, M. M. (2022). Iotprotect: A machine-learning based
iot intrusion detection system. In 2022 6th Interna-
tional Conference on Cryptography, Security and Pri-
vacy (CSP), pages 61–65. IEEE.
Alazab, M., RM, S. P., Parimala, M., Maddikunta, P. K. R.,
Gadekallu, T. R., and Pham, Q.-V. (2021). Federated
learning for cybersecurity: Concepts, challenges, and
future directions. IEEE Transactions on Industrial In-
formatics, 18(5):3501–3509.
autonomio (2022). talos. [Online; accessed 22. Dec. 2022].
Gupta, K., Sharma, D. K., Datta Gupta, K., and Kumar,
A. (2022). A tree classifier based network intrusion
detection model for internet of medical things. Com-
puters and Electrical Engineering, 102:108158.
Hady, A. A., Ghubaish, A., Salman, T., Unal, D., and Jain,
R. (2020). Intrusion detection system for healthcare
systems using medical and network data: A compari-
son study. IEEE Access, 8:106576–106584.
Kang, H., Ahn, D. H., Lee, G. M., Yoo, J. D., Park, K. H.,
and Kim, H. K. (2019). Iot network intrusion dataset.
IEEE Dataport.
Koroniotis, N., Moustafa, N., Sitnikova, E., and Turnbull,
B. (2019). Towards the development of realistic botnet
dataset in the internet of things for network forensic
analytics: Bot-iot dataset. Future Generation Com-
puter Systems, 100:779–796.
Learn, S.-K. (2022). scikit-learn: machine learning in
Python — scikit-learn 1.2.0 documentation. [Online;
accessed 22. Dec. 2022].
Lee, J. D., Cha, H. S., Rathore, S., and Park, J. H. (2021).
M-idm: A multi-classification based intrusion detec-
tion model in healthcare iot. Computers, Materials
and Continua, 67(2):1537–1553.
Lundberg, S. M. and Lee, S.-I. (2017). A unified approach
to interpreting model predictions. Advances in neural
information processing systems, 30.
Moustafa, N. (2021). A new distributed architecture for
evaluating ai-based security systems at the edge: Net-
work ton iot datasets. Sustainable Cities and Society,
72:102994.
Nandy, S., Adhikari, M., Khan, M. A., Menon, V. G., and
Verma, S. (2022). An intrusion detection mechanism
for secured iomt framework based on swarm-neural
network. IEEE Journal of Biomedical and Health In-
formatics, 26(5):1969–1976.
Raschka, S., Liu, Y. H., Mirjalili, V., and Dzhulgakov, D.
(2022). Machine Learning with PyTorch and Scikit-
Learn: Develop machine learning and deep learning
models with Python. Packt Publishing Ltd.
Reynolds, J. (1992). RFC 1340: Assigned Numbers. [On-
line; accessed 22. Dec. 2022].
Sharafaldin, I., Lashkari, A. H., Hakak, S., and Ghorbani,
A. A. (2019). Developing realistic distributed denial
of service (ddos) attack dataset and taxonomy. In 2019
International Carnahan Conference on Security Tech-
nology (ICCST), pages 1–8. IEEE.
Si-Ahmed, A., Al-Garadi, M. A., and Boustia, N. (2022).
Survey of machine learning based intrusion detection
methods for internet of medical things. arXiv preprint
arXiv:2202.09657.
Statista (2022a). eHealth Devices - Worldwide | Statista
Market Forecast. [Online; accessed 22. Dec. 2022].
Statista (2022b). eHealth Devices - Worldwide | Statista
Market Forecast. [Online; accessed 22. Dec. 2022].
Techopedia (2014). What is an Ephemeral Port? - Defi-
nition from Techopedia. [Online; accessed 22. Dec.
2022].
Wahab, F., Zhao, Y., Javeed, D., Al-Adhaileh, M. H., Al-
maaytah, S. A., Khan, W., Saeed, M. S., and Ku-
mar Shah, R. (2022). An ai-driven hybrid framework
for intrusion detection in iot-enabled e-health. Com-
putational Intelligence and Neuroscience, 2022.
XMeDNN: An Explainable Deep Neural Network System for Intrusion Detection in Internet of Medical Things
151