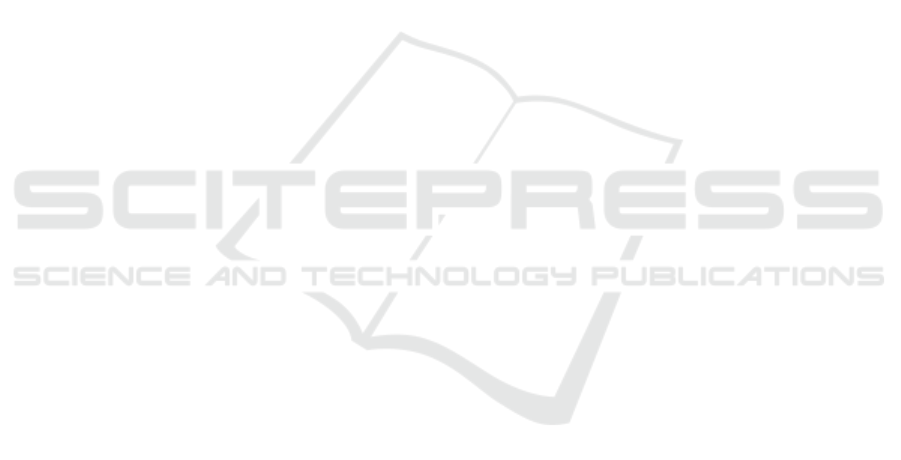
extraction systems by gibbs sampling. In Proceedings
of the 43rd annual meeting of the association for com-
putational linguistics (ACL’05), pages 363–370.
Freitas, C., Carvalho, P., Gonc¸alo Oliveira, H., Mota, C.,
and Santos, D. (2010). Second harem: advanc-
ing the state of the art of named entity recogni-
tion in portuguese. In quot; In Nicoletta Calzolari;
Khalid Choukri; Bente Maegaard; Joseph Mariani;
Jan Odijk; Stelios Piperidis; Mike Rosner; Daniel
Tapias (ed) Proceedings of the International Confer-
ence on Language Resources and Evaluation (LREC
2010)(Valletta 17-23 May de 2010) European Lan-
guage Resources Association. European Language
Resources Association.
Grishman, R. and Sundheim, B. M. (1996). Message under-
standing conference-6: A brief history. In COLING
1996 Volume 1: The 16th International Conference
on Computational Linguistics.
Gu, L., Zhang, W., Wang, Y., Li, B., and Mao, S. (2020).
Named entity recognition in judicial field based on
bert-bilstm-crf model. In 2020 International Work-
shop on Electronic Communication and Artificial In-
telligence (IWECAI), pages 170–174. IEEE.
Huang, Z., Xu, W., and Yu, K. (2015). Bidirectional
lstm-crf models for sequence tagging. arXiv preprint
arXiv:1508.01991.
Jia, C., Shi, Y., Yang, Q., and Zhang, Y. (2020). Entity en-
hanced bert pre-training for chinese ner. In Proceed-
ings of the 2020 Conference on Empirical Methods in
Natural Language Processing (EMNLP), pages 6384–
6396.
J
´
unior, C. M., Macedo, H., Bispo, T., Santos, F., Silva, N.,
and Barbosa, L. (2015). Paramopama: a brazilian-
portuguese corpus for named entity recognition. En-
contro Nac. de Int. Artificial e Computacional.
Labusch, K., Kulturbesitz, P., Neudecker, C., and Zellh
¨
ofer,
D. (2019). Bert for named entity recognition in con-
temporary and historical german. In Proceedings of
the 15th conference on natural language processing,
pages 9–11.
Lafferty, J., McCallum, A., and Pereira, F. C. (2001). Con-
ditional random fields: Probabilistic models for seg-
menting and labeling sequence data.
Lample, G., Ballesteros, M., Subramanian, S., Kawakami,
K., and Dyer, C. (2016). Neural architectures
for named entity recognition. arXiv preprint
arXiv:1603.01360.
Leitner, E., Rehm, G., and Moreno-Schneider, J. (2019).
Fine-grained named entity recognition in legal docu-
ments. In International Conference on Semantic Sys-
tems, pages 272–287. Springer.
Leitner, E., Rehm, G., and Moreno-Schneider, J. (2020). A
dataset of german legal documents for named entity
recognition. arXiv preprint arXiv:2003.13016.
Li, J., Zhao, S., Yang, J., Huang, Z., Liu, B., Chen, S., Pan,
H., and Wang, Q. (2020). Wcp-rnn: a novel rnn-based
approach for bio-ner in chinese emrs. The journal of
supercomputing, 76(3):1450–1467.
Liu, Y., Ott, M., Goyal, N., Du, J., Joshi, M., Chen, D.,
Levy, O., Lewis, M., Zettlemoyer, L., and Stoyanov,
V. (2019). Roberta: A robustly optimized bert pre-
training approach. arXiv preprint arXiv:1907.11692.
Luz de Araujo, P. H., Campos, T. E. d., de Oliveira,
R. R., Stauffer, M., Couto, S., and Bermejo, P. (2018).
Lener-br: a dataset for named entity recognition in
brazilian legal text. In International Conference on
Computational Processing of the Portuguese Lan-
guage, pages 313–323. Springer.
Mayfield, J., McNamee, P., and Piatko, C. (2003). Named
entity recognition using hundreds of thousands of
features. In Proceedings of the seventh conference
on Natural language learning at HLT-NAACL 2003,
pages 184–187.
Morwal, S., Jahan, N., and Chopra, D. (2012). Named en-
tity recognition using hidden markov model (hmm).
International Journal on Natural Language Comput-
ing (IJNLC) Vol, 1.
Pais, V., Mitrofan, M., Gasan, C. L., Ianov, A., Ghit, C.,
Coneschi, V. S., and Onut, A. Legalnero: A linked
corpus for named entity recognition in the romanian
legal domain.
Sang, E. F. and De Meulder, F. (2003). Introduction to
the conll-2003 shared task: Language-independent
named entity recognition. arXiv preprint cs/0306050.
Sarzynska-Wawer, J., Wawer, A., Pawlak, A., Szy-
manowska, J., Stefaniak, I., Jarkiewicz, M., and
Okruszek, L. (2021). Detecting formal thought disor-
der by deep contextualized word representations. Psy-
chiatry Research, 304:114135.
Shao, Y., Mao, J., Liu, Y., Ma, W., Satoh, K., Zhang, M.,
and Ma, S. (2020). Bert-pli: Modeling paragraph-
level interactions for legal case retrieval. In IJCAI,
pages 3501–3507.
Silveira, R., Fernandes, C., Neto, J. A. M., Furtado, V., and
Pimentel Filho, J. E. (2021). Topic modelling of legal
documents via legal-bert. Proceedings http://ceur-ws
org ISSN, 1613:0073.
Souza, F., Nogueira, R., and Lotufo, R. (2019). Por-
tuguese named entity recognition using bert-crf. arXiv
preprint arXiv:1909.10649.
Taher, E., Hoseini, S. A., and Shamsfard, M. (2020).
Beheshti-ner: Persian named entity recognition using
bert. arXiv preprint arXiv:2003.08875.
Vaswani, A., Shazeer, N., Parmar, N., Uszkoreit, J., Jones,
L., Gomez, A. N., Kaiser, Ł., and Polosukhin, I.
(2017). Attention is all you need. Advances in neural
information processing systems, 30.
Wehnert, S., Sudhi, V., Dureja, S., Kutty, L., Shahania, S.,
and De Luca, E. W. (2021). Legal norm retrieval with
variations of the bert model combined with tf-idf vec-
torization. In Proceedings of the eighteenth interna-
tional conference on artificial intelligence and law,
pages 285–294.
Zanuz, L. and Rigo, S. J. (2022). Fostering judiciary appli-
cations with new fine-tuned models for legal named
entity recognition in portuguese. In International
Conference on Computational Processing of the Por-
tuguese Language, pages 219–229. Springer.
ICAART 2023 - 15th International Conference on Agents and Artificial Intelligence
728