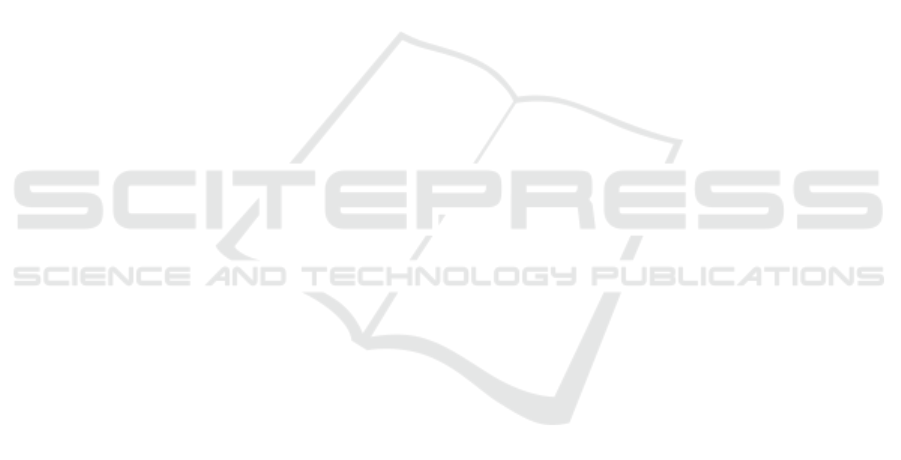
After showing all the problems examined by the
DSS during the assessment phase, the system checks
the presence of different problems related to the
datatype inside the reproducibility database. If any,
these are shown to the user. This step helps review
problems that, maybe, the analyst has not thought
about. In the end, the user could propose new prob-
lems to test, and they would be saved as new experi-
ences in the database.
5 CONCLUSIONS
DQ and DC are fundamental for any professional
working with data. This paper has proposed a frame-
work that helps users to qualitatively better under-
stand their data and to save time in pre-processing it.
The framework aims to give a general overview of
the data quality status. It computes indicators related
to DQ and DC to support the user in estimating the
time they need to spend performing the cleaning pro-
cess. Furthermore, the framework focuses on speed-
ing up the cleaning process, assisting the user during
the identification of any problem then providing pos-
sible solutions for any cleaning issues. The last part
of the paper described the application of the frame-
work in an industrial POC, the low voltage grid. It
was shown that some metrics are not always appli-
cable, but the framework can still be relevant. The
dataset employed contained public time series data
of energy consumption profiles for the 2016 calendar
year in Belgium.
In future work, the framework will be tested with
different types of datasets and use cases. Another fo-
cus will be on how to use historical experiences more
effectively and efficiently to better suggest cleaning
issues and solutions during the Analysis Phase. Then,
the module will be inserted into the design of a data-
driven decision support system.
ACKNOWLEDGEMENTS
The authors greatly thank Ms. Lola Botman
and Mr. Jonas Soenen (KU Leuven) for their
support and useful suggestions. This research
received funding by KU Leuven: • Research
Fund (projects C16/15/059, C3/19/053, C24/18/022,
C3/20/117, C3I-21-00316), Industrial Research Fund
(Fellowships 13-0260, IOFm/16/004) and sev-
eral Leuven Research and Development bilat-
eral industrial projects; • Flemish Government
Agencies: ◦ FWO: EOS Project no G0F6718N
(SeLMA), SBO project S005319N, Infrastructure
project I013218N, TBM Project T001919N; PhD
Grant (SB/1SA1319N), ◦ EWI: the Flanders AI Re-
search Program, ◦ VLAIO: CSBO (HBC.2021.0076)
Baekeland PhD (HBC.20192204) • European Com-
mission: European Research Council under the Euro-
pean Union’s Horizon 2020 research and innovation
programme (ERC Adv. Grant grant agreement No
885682); • Other funding: Foundation ‘Kom op tegen
Kanker’, CM (Christelijke Mutualiteit)
REFERENCES
Botman, L., Soenen, J., Theodorakos, K., Yurtman, A.,
Bekker, J., Vanthournout, K., Blockeel, H., Moor,
B. D., and Lago, J. (2022). A scalable ensemble
approach to forecast the electricity consumption of
households. IEEE Transactions on Smart Grid.
Chengalur-Smith, I., Ballou, D., and Pazer, H. (1999). The
impact of data quality information on decision mak-
ing: an exploratory analysis. IEEE Transactions on
Knowledge and Data Engineering.
Data Europa EU (2021). Data Quality Guidelines. Publica-
tions Office of the European Union.
Ehrlinger, L., Haunschmid, V., Palazzini, D., and Lettner,
C. (2019). A daql to monitor data quality in machine
learning applications. In Prooceedings of the 30th In-
ternational Conference on Database and Expert Sys-
tems Applications - Part I.
Ehrlinger, L. and W
¨
oß, W. (2017). Automated data qual-
ity monitoring. In Proceedings of the 22nd MIT In-
ternational Conference on Information Quality (ICIQ
2017).
Ehrlinger, L. and W
¨
oß, W. (2022). A survey of data quality
measurement and monitoring tools. Frontiers in Big
Data.
Eurostat (2020). Energy statistics - supply, transforma-
tion and consumption. https://www.eea.europa.eu/
data-and-maps/data/external/supply-transformation-
consumption-electricity-annual-data.
Kiefer, C. (2019). Quality indicators for text data. In BTW
2019 – Workshopband.
Oliveira, O. and Oliveira, B. (2022). An extensible frame-
work for data reliability assessment. In Proceedings
of the 24th International Conference on Enterprise In-
formation Systems - Volume 1: ICEIS,.
Sadiq, S. and Indulska, M. (2017). Open data: Quality over
quantity. International Journal of Information Man-
agement.
Soenen, J., Yurtman, A., Becker, T., D’hulst, R., Van-
thournout, K., Meert, W., and Blockeel, H. (2023).
Scenario generation of residential electricity con-
sumption through sampling of historical data. Sus-
tainable Energy, Grids and Networks.
Wilkinson, M. D., Dumontier, M., Aalbersberg, I. J., Apple-
ton, G., Axton, M., Baak, A., Blomberg, N., Boiten,
J.-W., da Silva Santos, L. B., Bourne, P. E., et al.
(2016). The fair guiding principles for scientific data
management and stewardship. Scientific Data.
ICEIS 2023 - 25th International Conference on Enterprise Information Systems
452