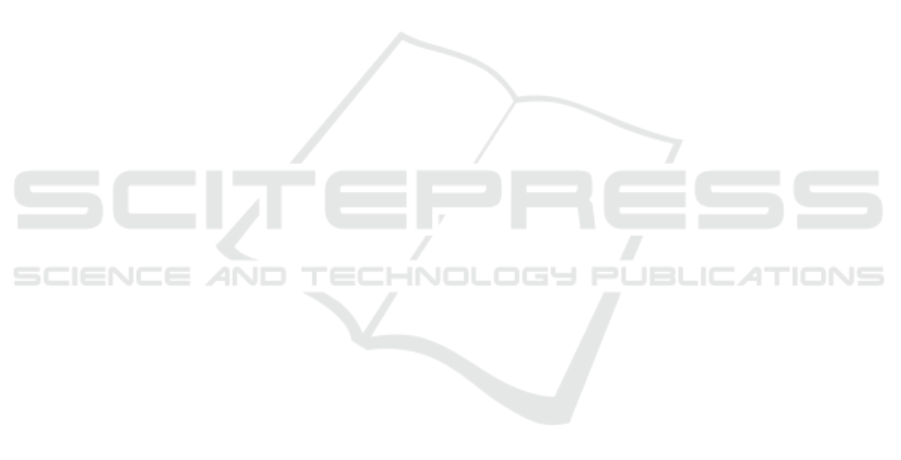
4 CONCLUSIONS
In this paper, we present a user-centred approach
for calibration and validation of agent-based models.
By including the end user in most of the modelling
phases, especially calibration and validation, we are
hoping for better explainability of simulation results
and a smoother transmission of expert knowledge.
We illustrate this position with an application dealing
with urban growth. Such an application involves com-
plex, spatially explicit models of human behaviour,
and experts who have some knowledge of the under-
lying mechanisms, but who want interpretable models
and acceptable results.
In order to achieve this goal, we think it is impor-
tant to involve more the experts in the model design.
They must be able to define behaviour rules them-
selves using a visual programming language. They
must also be able to define their validation measures
and to navigate easily between behaviour rules and
validation results. Machine learning methods can
help to automate and simplifying the calibration pro-
cess, but chosen methods must produce interpretable
models, such that experts can trust simulation results.
We are currently implementing such an approach with
a geographer in the setting of informal settlement
growth modelling (e.g. slums), and the first results
are very encouraging.
ACKNOWLEDGEMENTS
We specifically thank the University of South-Pacific
(USP) for participating in data acquisition for an in-
formal settlement in Fiji.
This work was financed by the Pacific Islands Uni-
versities Research Network (PIURN) and the French
Ministry for Foreign Affairs (Pacific Fund). It is now
supported by the French National Research Agency
(ANR SITI project).
REFERENCES
Bus¸oniu, L., Babu
ˇ
ska, R., and Schutter, B. D. (2010). Multi-
agent reinforcement learning: An overview. In Inno-
vations in Multi-Agent Systems and Applications - 1,
pages 183–221. Springer Berlin Heidelberg.
Crooks, A. (2018). Agent-Based Modelling and Geograph-
ical Information Systems: A Practical Primer. Spatial
Analysis and GIS. SAGE Publications.
Ferber, J. (1999). Multi-Agent Systems : An Introduction
to Distributed Artificial Intelligence. Addison Wesley
Longman.
Gonz
´
alez-M
´
endez, M., Olaya, C., Fasolino, I., Grimaldi,
M., and Obreg
´
on, N. (2021). Agent-Based Model-
ing for Urban Development Planning based on Hu-
man Needs. Conceptual Basis and Model Formula-
tion. Land Use Policy, 101:105110.
Grimm, V., Berger, U., Bastiansen, F., Eliassen, S., Ginot,
V., Giske, J., Goss-Custard, J., Grand, T., Heinz, S. K.,
Huse, G., Huth, A., Jepsen, J. U., Jørgensen, C.,
Mooij, W. M., M
¨
uller, B., Pe’er, G., Piou, C., Rails-
back, S. F., Robbins, A. M., Robbins, M. M., Ross-
manith, E., R
¨
uger, N., Strand, E., Souissi, S., Still-
man, R. A., Vabø, R., Visser, U., and DeAngelis, D. L.
(2006). A standard protocol for describing individual-
based and agent-based models. Ecological Modelling,
198(1-2):115–126.
Grimm, V., Berger, U., DeAngelis, D. L., Polhill, J. G.,
Giske, J., and Railsback, S. F. (2010). The ODD pro-
tocol: A review and first update. Ecological Mod-
elling, 221(23):2760–2768.
Heppenstall, A., Crooks, A., Malleson, N., Manley, E., Ge,
J., and Batty, M. (2021). Future Developments in Ge-
ographical Agent-Based Models: Challenges and Op-
portunities. Geographical Analysis, 53(1):76–91.
Jokar Arsanjani, J., Helbich, M., and de Noronha Vaz, E.
(2013). Spatiotemporal simulation of urban growth
patterns using agent-based modeling: The case of
Tehran. Cities, 32:33–42.
Lee, J.-S., Filatova, T., Ligmann-Zielinska, A., Hassani-
Mahmooei, B., Stonedahl, F., Lorscheid, I., Voinov,
A., Polhill, G., Sun, Z., and Parker, D. C. (2015). The
Complexities of Agent-Based Modeling Output Anal-
ysis. Journal of Artificial Societies and Social Simu-
lation, 18(4):4.
M
¨
uller, B., Bohn, F., Dreßler, G., Groeneveld, J., Klassert,
C., Martin, R., Schl
¨
uter, M., Schulze, J., Weise, H.,
and Schwarz, N. (2013). Describing human decisions
in agent-based models – ODD + D, an extension of the
ODD protocol. Environmental Modelling & Software,
48:37–48.
Orsi, F. and Geneletti, D. (2016-11). Transportation as
a protected area management tool: An agent-based
model to assess the effect of travel mode choices on
hiker movements. Computers, Environment and Ur-
ban Systems, 60:12–22.
Ozik, J., Collier, N., Combs, T., Macal, C. M., and North,
M. (2015). Repast simphony statecharts. Journal of
Artificial Societies and Social Simulation, 18(3).
Pires, B. and Crooks, A. T. (2017). Modeling the emergence
of riots: A geosimulation approach. Computers, Envi-
ronment and Urban Systems, 61:66–80.
Railsback, S. F. and Grimm, V. (2019). Agent-Based and
Individual-Based Modeling A Practical Introduction,
Second Edition. Princeton University Press.
Reuillon, R., Leclaire, M., and Rey-Coyrehourcq, S.
(2013). OpenMOLE, a workflow engine specifi-
cally tailored for the distributed exploration of simu-
lation models. Future Generation Computer Systems,
29(8):1981–1990.
Rockafellar, R. T. and Wets, R. J.-B. (2009). Variational
Analysis. Springer Science & Business Media.
ICAART 2023 - 15th International Conference on Agents and Artificial Intelligence
328