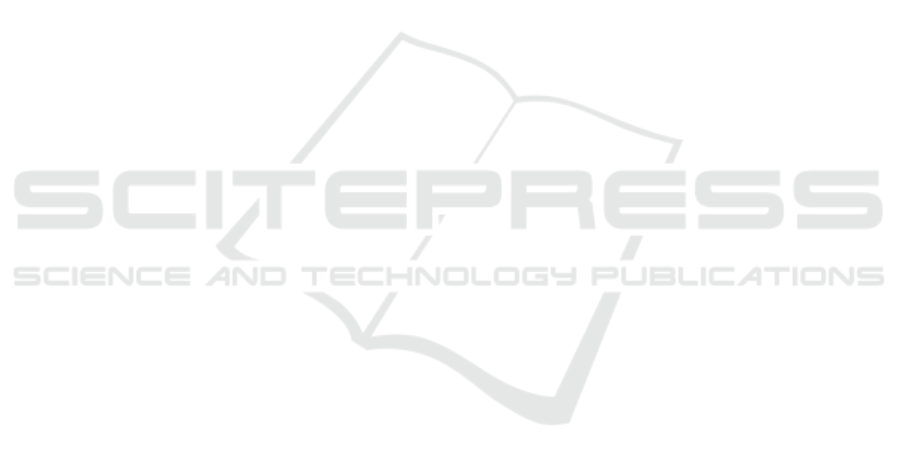
such differences are not significant if we consider that
the trials not meeting the C
2
criterion present a clus-
tering behavior that is correlated with the electrodes
placement. Moreover, the fact of a better robustness
of the MDS outcome against signals reduction when
assessing the C
1
criterion, confirms the effectiveness
of the proposed method in establishing correlations
between the EEG recordings and the electrodes spa-
tial distribution.
4 CONCLUSIONS
In this work, the raw multichannel EEG recordings
from the SEED-IV database were analyzed using the
MDS technique combined with the Procrustes algo-
rithm. To the best of our knowledge, this is the first
work establishing a direct link between EEG record-
ings and recording electrodes position.
Regarding multichannel EEG studies, an issue
that remains unsolved is related to determining the
number of electrodes and the recording sites that pro-
vides relevant information on emotions. It is a com-
mon practice defining a fixed set of electrodes sites
on the scalp for studying a given population. Re-
cent investigations pose a subject-specific setup of
EEG recording sites (Gannouni et al., 2021; Ozel
and Akan, 2021). Our results suggest that the EEG
time series from most of the SEED-IV trials con-
tain information of their underlying recording locus,
so that the electrodes spatial distribution can be re-
covered. This outcome may support the importance
of defining a set of EEG recording sites specific to
each subject. Moreover, the proposed methodology
sheds some light upon the information content of si-
multaneous EEG signals and its correlation with the
underlying cerebral structures. We did not focus on
the 32% trials of the database from which the elec-
trodes position cannot be recovered by means of the
MDS analysis. Our next research work will aim to
unveil the causes and implications of such outcome.
Although we did not observe a clear correlation be-
tween proper Procrustes alignment and evoked emo-
tions, the recordings obtained during neutral emotion
induction show a slightly superior alignment capacity
with regard to remaining emotions. Further studies
are needed to assess the significance of such differ-
entiated behavior. Additionally, we analyzed multiple
EEG recordings corresponding to 15 subjects (72 tri-
als per subject), which may generate a self-bias due to
similar cognitive patterns of the same subject. There-
fore, the proposed methodology requires further vali-
dation with a larger population.
REFERENCES
Cha, S.-H. (2007). Comprehensive survey on dis-
tance/similarity measures between probability density
functions. City, 1(2):1.
Doma, V. and Pirouz, M. (2020). A comparative analysis
of machine learning methods for emotion recognition
using EEG and peripheral physiological signals. Jour-
nal of Big Data, 7(1):1–21.
Gannouni, S., Aledaily, A., Belwafi, K., and Aboalsamh, H.
(2021). Emotion detection using electroencephalogra-
phy signals and a zero-time windowing-based epoch
estimation and relevant electrode identification. Sci
Rep, 11(1):7071.
Jia, Z., Lin, Y., Cai, X., Chen, H., Gou, H., and Wang, J.
(2020). SST-EmotionNet: Spatial-Spectral-Temporal
based Attention 3D Dense Network for EEG Emotion
Recognition. In MM 2020 - Proceedings of the 28th
ACM International Conference on Multimedia, pages
2909–2917. Association for Computing Machinery,
Inc.
Kendall, D. G. (1989). A Survey of the Statistical The-
ory of Shape. https://doi.org/10.1214/ss/1177012582,
4(2):87–99.
Ozel, P. and Akan, A. (2021). Channel contributions of
eeg in emotion modelling based on multivariate adap-
tive orthogonal signal decomposition. IETE Journal
of Research, 0(0):1–12.
Rahman, M. M., Sarkar, A. K., Hossain, M. A., Hossain,
M. S., Islam, M. R., Hossain, M. B., Quinn, J. M., and
Moni, M. A. (2021). Recognition of human emotions
using eeg signals: A review. Computers in Biology
and Medicine, 136:104696.
Torgerson, W. S. (1952). Multidimensional scaling: I. The-
ory and method. Psychometrika, 17(4):401–419.
Warheit, K. I., Rohlf, F. J., and Bookstein, F. L. (1992). Pro-
ceedings of the Michigan Morphometrics Workshop.
Systematic Biology, 41(3):392.
Xu, B., Kajimoto, H., Konyo, M., Saga, S., and Hatzfeld
(2004). Perceptual scaling of the gloss of a one-
dimensional series of painted black samples. Textile
Chemist & Colorist, 29(3):1–18.
Zheng, W. L., Liu, W., Lu, Y., Lu, B. L., and Cichocki, A.
(2019). EmotionMeter: A Multimodal Framework for
Recognizing Human Emotions. IEEE Transactions on
Cybernetics, 49(3):1110–1122.
BIOSIGNALS 2023 - 16th International Conference on Bio-inspired Systems and Signal Processing
316