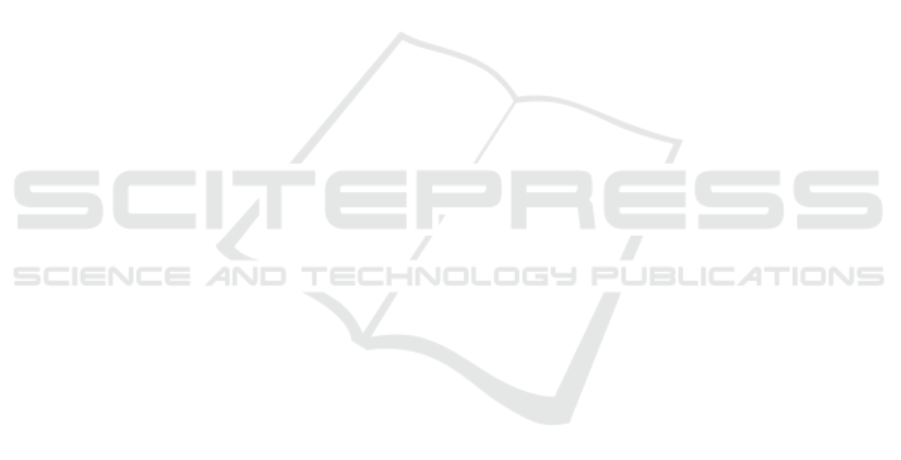
IEEE transactions on medical imaging, 38(10):2281–
2292.
Guo, C., Szemenyei, M., Yi, Y., Wang, W., Chen, B.,
and Fan, C. (2021). Sa-unet: Spatial attention u-net
for retinal vessel segmentation. In 2020 25th inter-
national conference on pattern recognition (ICPR),
pages 1236–1242. IEEE.
Hu, J., Wang, H., Wang, J., Wang, Y., He, F., and Zhang, J.
(2021). Sa-net: A scale-attention network for medical
image segmentation. PloS one, 16(4):e0247388.
Irshad, S. and Akram, M. U. (2014). Classification of reti-
nal vessels into arteries and veins for detection of hy-
pertensive retinopathy. In 2014 Cairo International
Biomedical Engineering Conference (CIBEC), pages
133–136. IEEE.
Kolb, H. (2012). Simple anatomy of the retina.
Li, L., Verma, M., Nakashima, Y., Nagahara, H., and
Kawasaki, R. (2020). Iternet: Retinal image seg-
mentation utilizing structural redundancy in vessel
networks. In Proceedings of the IEEE/CVF winter
conference on applications of computer vision, pages
3656–3665.
Lin, T.-Y., Goyal, P., Girshick, R., He, K., and Doll
´
ar, P.
(2017). Focal loss for dense object detection. In
Proceedings of the IEEE international conference on
computer vision, pages 2980–2988.
Long, J., Shelhamer, E., and Darrell, T. (2015). Fully con-
volutional networks for semantic segmentation. In
Proceedings of the IEEE conference on computer vi-
sion and pattern recognition, pages 3431–3440.
Luo, W., Li, Y., Urtasun, R., and Zemel, R. (2016). Under-
standing the effective receptive field in deep convolu-
tional neural networks. Advances in neural informa-
tion processing systems, 29.
Ma, N., Zhang, X., Zheng, H.-T., and Sun, J. (2018). Shuf-
flenet v2: Practical guidelines for efficient cnn archi-
tecture design. In Proceedings of the European con-
ference on computer vision (ECCV), pages 116–131.
Mou, L., Zhao, Y., Fu, H., Liu, Y., Cheng, J., Zheng, Y.,
Su, P., Yang, J., Chen, L., Frangi, A. F., et al. (2021).
Cs2-net: Deep learning segmentation of curvilinear
structures in medical imaging. Medical image anal-
ysis, 67:101874.
Owen, C. G., Rudnicka, A. R., Mullen, R., Barman, S. A.,
Monekosso, D., Whincup, P. H., Ng, J., and Paterson,
C. (2009). Measuring retinal vessel tortuosity in 10-
year-old children: validation of the computer-assisted
image analysis of the retina (caiar) program. Inves-
tigative ophthalmology & visual science, 50(5):2004–
2010.
Pandey, A. (2018). Depth-wise convolution and depth-wise
separable convolution.
Payne, A. J., Kaja, S., Naumchuk, Y., Kunjukunju, N.,
and Koulen, P. (2014). Antioxidant drug therapy ap-
proaches for neuroprotection in chronic diseases of
the retina. International journal of molecular sci-
ences, 15(2):1865–1886.
Ronneberger, O., Fischer, P., and Brox, T. (2015). U-net:
Convolutional networks for biomedical image seg-
mentation. In International Conference on Medical
image computing and computer-assisted intervention,
pages 234–241. Springer.
Salmon, J. F. (2019). Kanski’s Clinical Ophthalmology E-
Book: A Systematic Approach. Elsevier Health Sci-
ences.
Shi, W. and Caballero, J. (1874). Ferenc husz
´
a r, johannes
totz, andrew p aitken, rob bishop, daniel rueckert, and
zehan wang. 2016. real-time single image and video
super-resolution using an efficient sub-pixel convolu-
tional neural network. In Conf. on computer vision
and pattern recognition (CVPR), volume 1883.
Staal, J., Abr
`
amoff, M. D., Niemeijer, M., Viergever, M. A.,
and Van Ginneken, B. (2004). Ridge-based vessel seg-
mentation in color images of the retina. IEEE trans-
actions on medical imaging, 23(4):501–509.
Walker, H. K., Hall, W. D., and Hurst, J. W. (1990). Clinical
methods: the history, physical, and laboratory exami-
nations.
Yang, X., Li, Z., Guo, Y., and Zhou, D. (2022). Dcu-net:
a deformable convolutional neural network based on
cascade u-net for retinal vessel segmentation. Multi-
media Tools and Applications, 81(11):15593–15607.
Zahangir Alom, M., Hasan, M., Yakopcic, C., Taha, T. M.,
and Asari, V. K. (2018). Recurrent residual convo-
lutional neural network based on u-net (r2u-net) for
medical image segmentation. arXiv e-prints, pages
arXiv–1802.
Zhang, X., Zhou, X., Lin, M., and Sun, J. (2018). Shuf-
flenet: An extremely efficient convolutional neural
network for mobile devices. In Proceedings of the
IEEE conference on computer vision and pattern
recognition, pages 6848–6856.
Zhou, Y., Yu, H., and Shi, H. (2021). Study group learning:
Improving retinal vessel segmentation trained with
noisy labels. In International Conference on Medi-
cal Image Computing and Computer-Assisted Inter-
vention, pages 57–67. Springer.
Zhuang, J. (2018). Laddernet: Multi-path networks based
on u-net for medical image segmentation. arXiv
preprint arXiv:1810.07810.
Zuiderveld, K. (1994). Contrast limited adaptive histogram
equalization. Graphics gems, pages 474–485.
EFL-Net: An Efficient Lightweight Neural Network Architecture for Retinal Vessel Segmentation
927