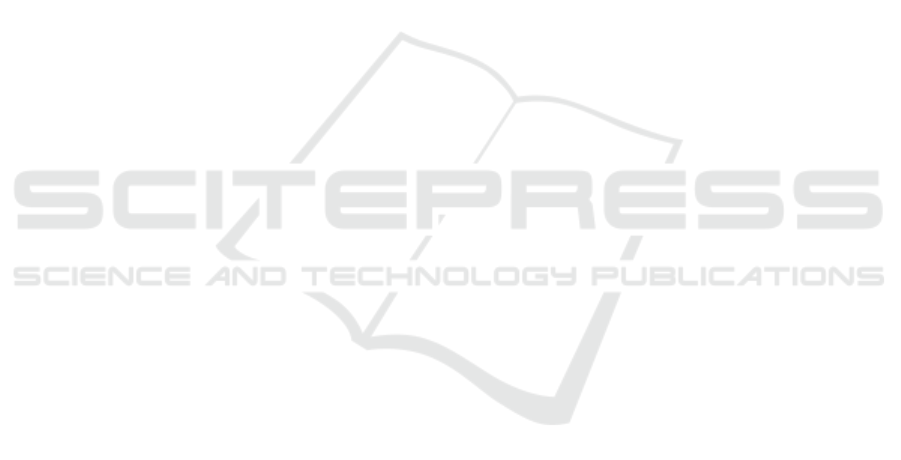
of the IEEE international conference on computer vi-
sion, pages 2470–2478.
Gkioxari, G., Girshick, R., and Malik, J. (2015b). Contex-
tual action recognition with r* cnn. In Proceedings
of the IEEE international conference on computer vi-
sion, pages 1080–1088.
He, K., Zhang, X., Ren, S., and Sun, J. (2016). Deep resid-
ual learning for image recognition. In Proceedings of
the IEEE conference on computer vision and pattern
recognition, pages 770–778.
Ji, J., Desai, R., and Niebles, J. C. (2021). Detecting human-
object relationships in videos. In Proceedings of the
IEEE/CVF International Conference on Computer Vi-
sion, pages 8106–8116.
Kim, B., Lee, J., Kang, J., Kim, E.-S., and Kim, H. J.
(2021). Hotr: End-to-end human-object interaction
detection with transformers. In Proceedings of the
IEEE/CVF Conference on Computer Vision and Pat-
tern Recognition, pages 74–83.
Kim, B., Mun, J., On, K.-W., Shin, M., Lee, J., and Kim, E.-
S. (2022). Mstr: Multi-scale transformer for end-to-
end human-object interaction detection. In Proceed-
ings of the IEEE/CVF Conference on Computer Vision
and Pattern Recognition, pages 19578–19587.
Li, Y.-L., Liu, X., Wu, X., Li, Y., Qiu, Z., Xu, L., Xu, Y.,
Fang, H.-S., and Lu, C. (2022a). Hake: A knowledge
engine foundation for human activity understanding.
Li, Y.-L., Xu, L., Liu, X., Huang, X., Xu, Y., Wang, S.,
Fang, H.-S., Ma, Z., Chen, M., and Lu, C. (2020).
Pastanet: Toward human activity knowledge engine.
In CVPR.
Li, Z., Zou, C., Zhao, Y., Li, B., and Zhong, S. (2022b). Im-
proving human-object interaction detection via phrase
learning and label composition. In Proceedings of
the AAAI Conference on Artificial Intelligence, vol-
ume 36, pages 1509–1517.
Liao, Y., Liu, S., Wang, F., Chen, Y., Qian, C., and Feng,
J. (2020). Ppdm: Parallel point detection and match-
ing for real-time human-object interaction detection.
In Proceedings of the IEEE/CVF Conference on Com-
puter Vision and Pattern Recognition, pages 482–490.
Lin, T.-Y., Goyal, P., Girshick, R., He, K., and Doll
´
ar, P.
(2017). Focal loss for dense object detection. In
Proceedings of the IEEE international conference on
computer vision, pages 2980–2988.
Lu, C., Su, H., Li, Y., Lu, Y., Yi, L., Tang, C.-K., and
Guibas, L. J. (2018). Beyond holistic object recogni-
tion: Enriching image understanding with part states.
In CVPR.
Ma, X., Nie, W., Yu, Z., Jiang, H., Xiao, C., Zhu, Y., Zhu,
S.-C., and Anandkumar, A. (2022). Relvit: Concept-
guided vision transformer for visual relational reason-
ing. arXiv preprint arXiv:2204.11167.
Rezatofighi, H., Tsoi, N., Gwak, J., Sadeghian, A., Reid, I.,
and Savarese, S. (2019). Generalized intersection over
union: A metric and a loss for bounding box regres-
sion. In Proceedings of the IEEE/CVF conference on
computer vision and pattern recognition, pages 658–
666.
Sunkesula, S. P. R., Dabral, R., and Ramakrishnan, G.
(2020). Lighten: Learning interactions with graph and
hierarchical temporal networks for hoi in videos. In
Proceedings of the 28th ACM International Confer-
ence on Multimedia, pages 691–699.
Tamura, M., Ohashi, H., and Yoshinaga, T. (2021). Qpic:
Query-based pairwise human-object interaction detec-
tion with image-wide contextual information. In Pro-
ceedings of the IEEE/CVF Conference on Computer
Vision and Pattern Recognition, pages 10410–10419.
Yuan, H., Wang, M., Ni, D., and Xu, L. (2022). De-
tecting human-object interactions with object-guided
cross-modal calibrated semantics. arXiv preprint
arXiv:2202.00259.
Zhang, A., Liao, Y., Liu, S., Lu, M., Wang, Y., Gao, C., and
Li, X. (2021a). Mining the benefits of two-stage and
one-stage hoi detection. Advances in Neural Informa-
tion Processing Systems, 34:17209–17220.
Zhang, F. Z., Campbell, D., and Gould, S. (2021b). Spa-
tially conditioned graphs for detecting human-object
interactions. In Proceedings of the IEEE/CVF In-
ternational Conference on Computer Vision, pages
13319–13327.
Zhou, D., Liu, Z., Wang, J., Wang, L., Hu, T., Ding, E.,
and Wang, J. (2022). Human-object interaction de-
tection via disentangled transformer. In Proceedings
of the IEEE/CVF Conference on Computer Vision and
Pattern Recognition, pages 19568–19577.
Zhu, X., Su, W., Lu, L., Li, B., Wang, X., and Dai, J.
(2020). Deformable detr: Deformable transform-
ers for end-to-end object detection. arXiv preprint
arXiv:2010.04159.
Body Part Information Additional in Multi-decoder Transformer-Based Network for Human Object Interaction Detection
229