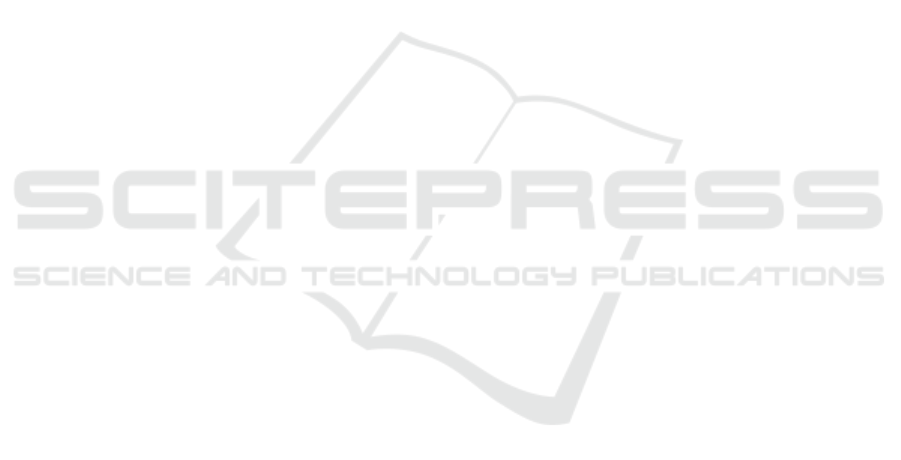
Harada, S., Hayashi, H., and Uchida, S. (2018). Biosig-
nal data augmentation based on generative adversarial
networks. volume 2018, pages 368–371.
Hazra, D. and Byun, Y.-C. (2020). SynSigGAN: Gener-
ative Adversarial Networks for Synthetic Biomedical
Signal Generation. Biology, 9(12):441.
Hernang
´
omez, R., Visentin, T., Servadei, L., Khod-
abakhshandeh, H., and Sta
´
nczak, S. (2022). Im-
proving Radar Human Activity Classification Using
Synthetic Data with Image Transformation. Sensors,
22(4):1519.
Jabbar, A., Li, X., and Omar, B. (2022). A Survey on Gen-
erative Adversarial Networks: Variants, Applications,
and Training. ACM Computing Surveys, 54(8):1–49.
Karras, T., Laine, S., and Aila, T. (2021). A style-based
generator architecture for generative adversarial net-
works. IEEE Transactions on Pattern Analysis and
Machine Intelligence, 43(12):4217–4228.
Kiranyaz, S., Devecioglu, O., Ince, T., Malik, J., Chowd-
hury, M., Hamid, T., Mazhar, R., Khandakar, A.,
Tahir, A., Rahman, T., and Gabbouj, M. (2022). Blind
ecg restoration by operational cycle-gans. IEEE Trans
Biomed Eng.
Kiyasseh, D., Tadesse, G. A., Nhan, L. N. T., Van Tan,
L., Thwaites, L., Zhu, T., and Clifton, D. (2020).
PlethAugment: GAN-Based PPG Augmentation
for Medical Diagnosis in Low-Resource Settings.
IEEE Journal of Biomedical and Health Informatics,
24(11):3226–3235.
Lo, J., Cardinell, J., Costanzo, A., and Sussman, D. (2021).
Medical Augmentation (Med-Aug) for Optimal Data
Augmentation in Medical Deep Learning Networks.
Sensors, 21(21):7018.
Lu, H., Han, H., and Zhou, S. K. (2021). Dual-GAN:
Joint BVP and Noise Modeling for Remote Physio-
logical Measurement. In 2021 IEEE/CVF Conference
on Computer Vision and Pattern Recognition (CVPR),
pages 12399–12408, Nashville, TN, USA. IEEE.
Luo, Y., Cai, X., Zhang, Y., and Xu, J. (2018). Multivariate
Time Series Imputation with Generative Adversarial
Networks. page 12.
Metz, L., Poole, B., Pfau, D., and Sohl-Dickstein, J. (2017).
Unrolled generative adversarial networks. In Interna-
tional Conference on Learning Representations.
Montero, A., Bonet-Carne, E., and Burgos-Artizzu, X. P.
(2021). Generative Adversarial Networks to Improve
Fetal Brain Fine-Grained Plane Classification. Sen-
sors, 21(23):7975.
Nguyen, H., Zhuang, D., Wu, P.-Y., and Chang, M.
(2020). AutoGAN-based dimension reduction for pri-
vacy preservation. Neurocomputing, 384:94–103.
Ning, X., Yao, L., Wang, X., Benatallah, B., Zhang, S.,
and Zhang, X. (2018). Data-Augmented Regression
with Generative Convolutional Network. In Hacid,
H., Cellary, W., Wang, H., Paik, H.-Y., and Zhou,
R., editors, Web Information Systems Engineering –
WISE 2018, volume 11234, pages 301–311. Springer
International Publishing, Cham. Series Title: Lecture
Notes in Computer Science.
Piacentino, E., Guarner, A., and Angulo, C. (2021). Gen-
erating synthetic ecgs using gans for anonymizing
healthcare data. Electronics, 10(4):389.
Ping, H., Stoyanovich, J., and Howe, B. (2017). Data-
synthesizer: Privacy-preserving synthetic datasets. In
Proceedings of the 29th International Conference on
Scientific and Statistical Database Management, SS-
DBM ’17, New York, NY, USA. Association for Com-
puting Machinery.
Reiter, J. P. (2005). Using cart to generate partially synthetic
public use microdata. Journal of Official Statistics,
21:441–462.
Sabry, F., Eltaras, T., Labda, W., Alzoubi, K., and Malluhi,
Q. (2022a). Machine learning for healthcare wearable
devices: The big picture. Journal of Healthcare Engi-
neering.
Sabry, F., Eltaras, T., Labda, W., Hamza, F., Alzoubi, K.,
and Malluhi, Q. (2022b). Towards on-device dehy-
dration monitoring using machine learning from wear-
able device’s data. Sensors, 22(5).
Thambawita, V., Hicks, S., Isaksen, J., Stensen, M., Hau-
gen, T., Kanters, J., Parasa, S., de Lange, T., Johansen,
H., Johansen, D., Hammer, H., Halvorsen, P., and
Riegler, M. (2021). Deepsynthbody: the beginning
of the end for data deficiency in medicine. pages 1–8.
Um, T. T., Pfister, F. M. J., Pichler, D., Endo, S., Lang, M.,
Hirche, S., Fietzek, U., and Kuli
´
c, D. (2017). Data
Augmentation of Wearable Sensor Data for Parkin-
son’s Disease Monitoring using Convolutional Neural
Networks. In Proceedings of the 19th ACM Interna-
tional Conference on Multimodal Interaction, pages
216–220. arXiv:1706.00527 [cs].
van der Maaten, L. and Hinton, G. (2008). Visualizing data
using t-sne. Journal of Machine Learning Research,
9(86):2579–2605.
Xin, B., Yang, W., Geng, Y., Chen, S., Wang, S., and
Huang, L. (2020). Private FL-GAN: Differential Pri-
vacy Synthetic Data Generation Based on Federated
Learning. In ICASSP 2020 - 2020 IEEE International
Conference on Acoustics, Speech and Signal Process-
ing (ICASSP), pages 2927–2931, Barcelona, Spain.
IEEE.
Xu, L., Skoularidou, M., Cuesta-Infante, A., and Veera-
machaneni, K. (2019). Modeling Tabular data using
Conditional GAN. arXiv, page 11.
Yoon, J., Jarrett, D., and Schaar, M. (2019). Time-
series generative adversarial networks. In Wallach,
H., Larochelle, H., Beygelzimer, A., d'Alch
´
e-Buc, F.,
Fox, E., and Garnett, R., editors, Advances in Neural
Information Processing Systems, volume 32. Curran
Associates, Inc.
Zargari, A. H. A., Aqajari, S. A. H., Khodabandeh,
H., Rahmani, A. M., and Kurdahi, F. (2021).
An Accurate Non-accelerometer-based PPG Mo-
tion Artifact Removal Technique using CycleGAN.
arXiv:2106.11512 [cs]. arXiv: 2106.11512.
Zhang, J., Cormode, G., Procopiuc, C. M., Srivastava, D.,
and Xiao, X. (2017). Privbayes: Private data release
via bayesian networks. ACM Trans. Database Syst.,
42(4).
BIOSIGNALS 2023 - 16th International Conference on Bio-inspired Systems and Signal Processing
104