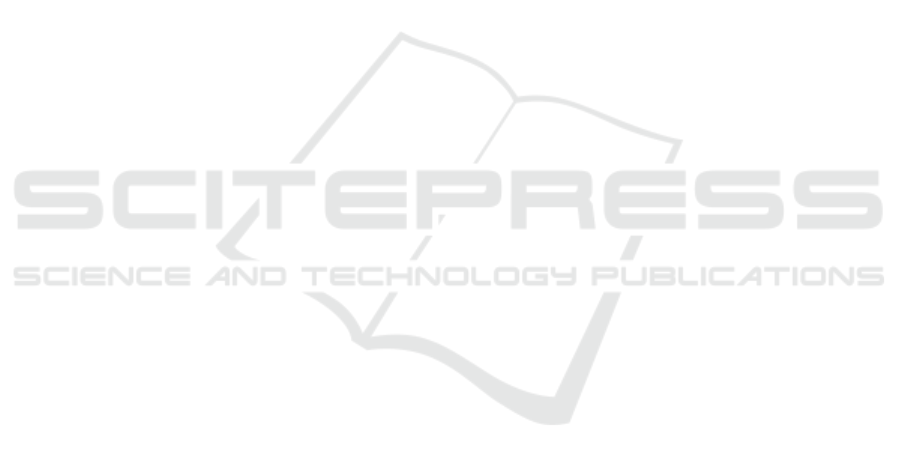
contribution measures in terms of number of words
added or modified in a text. Ultimately, it would be
worthwhile to study how to enable teachers to take
actions from useful visualizations of indicators and
strategies, and evaluate their acceptability, usability
and utility.
ACKNOWLEDGMENTS
The project is co-financed by the Brittany Region
within the research program SAD and the Finist
`
ere
Department Council within the research program
APRE. We thank all the teachers having participated
to the study.
REFERENCES
Baker, M. (1994). A model for negotiation in teaching-
learning dialogues. Journal of Interactive Learning
Research, 5(2):199.
Chen, J., Wang, M., Kirschner, P. A., and Tsai, C.-C.
(2018). The role of collaboration, computer use, learn-
ing environments, and supporting strategies in cscl:
A meta-analysis. Review of Educational Research,
88(6):799–843.
d’Ham, C., Wajeman, C., Girault, I., and Marzin-Janvier,
P. (2019). LabNbook, plateforme num
´
erique support
des p
´
edagogies actives et collaboratives en sciences
exp
´
erimentales. In Broisin, J., Sanchez, E., Yessad,
A., and Chenevotot, F., editors, EIAH 2019 : En-
vironnement Informatiques pour l’Apprentissage Hu-
main, Actes de la 9
`
eme Conf
´
erence sur les Environ-
nements Informatiques pour l’Apprentissage Humain,
pages 49–60, Paris, France.
Dillenbourg, P. (1999). What do you mean by collaborative
learning?, page 1–19. Elsevier, Oxford.
Dillenbourg, P. and Michael, B. (1996). Negotiation spaces
in human-computer collaborative learning. In Pro-
ceedings of the International Conference on Cooper-
ative Systems, page 12–14.
Hoffmann, C., Mandran, N., d’Ham, C., Rebaudo, S., and
Haddouche, M. A. (2022). Development of actionable
insights for regulating students’ collaborative writ-
ing of scientific texts. In Educating for a New Fu-
ture: Making Sense of Technology-Enhanced Learn-
ing Adoption, Lecture Notes in Computer Science,
page 534–541, Cham. Springer International Publish-
ing.
Larsen-Ledet, I. and Korsgaard, H. (2019). Territorial func-
tioning in collaborative writing. Computer Supported
Cooperative Work (CSCW), 28(3):391–433.
Li, M. and Zhu, W. (2016). Explaining dynamic interac-
tions in wiki-based collaborative writing. Language
Learning & Technology, 21(2):96–120.
Lowry, P. B., Curtis, A., and Lowry, M. R. (2004). Building
a taxonomy and nomenclature of collaborative writ-
ing to improve interdisciplinary research and prac-
tice. The Journal of Business Communication (1973),
41(1):66–99.
Mandran, N., Vermeulen, M., and Prior, E. (2022). Thedre’s
framework: Empowering phd candidates to efficiently
implement design-based research. Education and In-
formation Technologies, pages 1–24.
Olson, J. S., Wang, D., Olson, G. M., and Zhang, J. (2017).
How people write together now: Beginning the in-
vestigation with advanced undergraduates in a project
course. ACM Transactions on Computer-Human In-
teraction, 24(1):4:1–4:40.
Onrubia, J. and Engel, A. (2009). Strategies for collab-
orative writing and phases of knowledge construc-
tion in cscl environments. Computers & Education,
53(4):1256–1265.
Posner, I. R. and Baecker, R. M. (1992). How people write
together (groupware). In Proceedings of the Twenty-
Fifth Hawaii International Conference on System Sci-
ences, volume 4, page 127–138. IEEE.
Ratcliff, J. W. and Metzener, D. E. (1988). Pattern-
matching-the gestalt approach. Dr Dobbs Journal,
13(7):46.
Sadvilkar, N. and Neumann, M. (2020). PySBD: Pragmatic
sentence boundary disambiguation. In Proceedings
of Second Workshop for NLP Open Source Software
(NLP-OSS), pages 110–114, Online. Association for
Computational Linguistics.
Storch, N. (2013). Collaborative Writing in L2 Classrooms.
Multilingual Matters, Bristol, Blue Ridge Summit.
Sun, Z., Lin, C.-H., Wu, M., Zhou, J., and Luo, L. (2018).
A tale of two communication tools: Discussion-forum
and mobile instant-messaging apps in collaborative
learning. British Journal of Educational Technology,
49(2):248–261.
Sundgren, M. and Jaldemark, J. (2020). Visualizing online
collaborative writing strategies in higher education
group assignments. The International Journal of In-
formation and Learning Technology, 37(5):351–373.
Wang, D., Tan, H., and Lu, T. (2017). Why users do
not want to write together when they are writing
together: Users’ rationales for today’s collaborative
writing practices. Proceedings of the ACM on Human-
Computer Interaction, 1(CSCW):1–18.
Wang, F. and Hannafin, M. J. (2005). Design-based
research and technology-enhanced learning environ-
ments. Educational technology research and develop-
ment, 53(4):5–23.
Zhang, M. and Chen, W. (2022). Assessing collaborative
writing in the digital age: An exploratory study. Jour-
nal of Second Language Writing, 57:100868.
Zhang, M., Gibbons, J., and Li, M. (2021). Computer-
mediated collaborative writing in l2 classrooms: A
systematic review. Journal of Second Language Writ-
ing, 54:100854.
CSEDU 2023 - 15th International Conference on Computer Supported Education
502