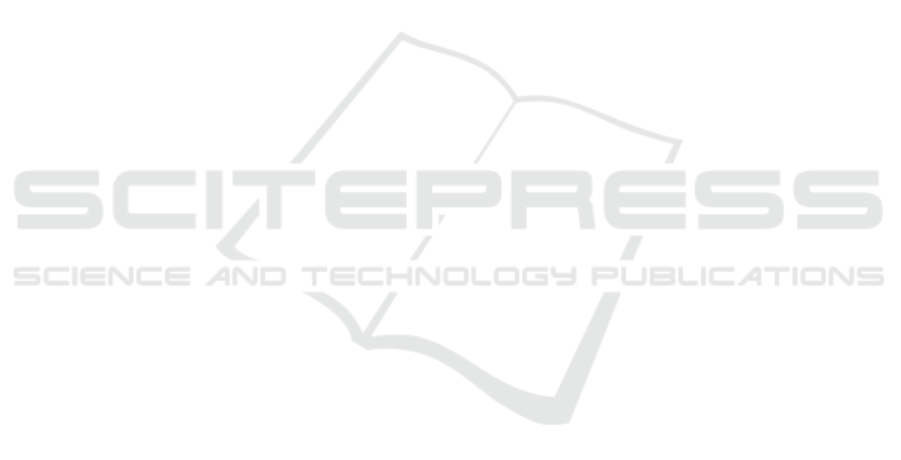
REFERENCES
Abdul, A., Vermeulen, J., Wang, D., Lim, B. Y., and
Kankanhalli, M. (2018). Trends and trajectories for
explainable, accountable and intelligible systems: An
HCI research agenda. In Proceedings of the 2018 CHI
Conference on Human Factors in Computing Systems,
pages 582:1–582:18, New York, NY, USA. ACM.
Bae, J., Helldin, T., Riveiro, M., Nowaczyk, S., Bouguelia,
M.-R., and Falkman, G. (2020). Interactive clustering:
A comprehensive review. ACM Computing Surveys,
53(1):1:1–1:39.
Basu, S., Banerjee, A., and Mooney, R. J. (2004). Active
semi-supervision for pairwise constrained clustering.
In Proceedings of the 2004 SIAM International Con-
ference on Data Mining, pages 333–344, Lake Buena
Vista, Florida, USA. Society for Industrial and Ap-
plied Mathematics.
Bezdek, J. C. (1981). Pattern Recognition with Fuzzy Ob-
jective Function Algorithms. Advanced Applications
in Pattern Recognition. Springer US, New York, NY,
USA.
Bilenko, M., Basu, S., and Mooney, R. J. (2004). Integrat-
ing constraints and metric learning in semi-supervised
clustering. In 21st International Conference on Ma-
chine Learning, page 11, Banff, Alberta, Canada.
ACM Press.
Blotevogel, H. H., Danielzyk, R., and M
¨
unter, A. (2014).
Spatial planning in germany. In Spatial planning sys-
tems and practices in Europe. Routledge Taylor &
Francis Group, London, UK and New York, NY, USA.
Briassoulis, H. (1997). How the others plan: Exploring
the shape and forms of informal planning. Journal
of Planning Education and Research, 17(2):105–117.
Caruana, R., Elhawary, M., Nguyen, N., and Smith, C.
(2006). Meta clustering. In 6th International Con-
ference on Data Mining, pages 107–118, Hong Kong,
China. IEEE.
Coden, A., Danilevsky, M., Gruhl, D., Kato, L., and Na-
garajan, M. (2017). A method to accelerate human in
the loop clustering. In Proceedings of the 2017 SIAM
International Conference on Data Mining, Proceed-
ings, pages 237–245, Houston, Texas, USA. Society
for Industrial and Applied Mathematics.
Doshi-Velez, F. and Kim, B. (2017). Towards a rigorous
science of interpretable machine learning.
Gleicher, M., Albers, D., Walker, R., Jusufi, I., Hansen,
C. D., and Roberts, J. C. (2011). Visual comparison
for information visualization. Information Visualiza-
tion, 10(4):289–309.
High-Level Expert Group on AI (2019). Ethics guidelines
for trustworthy AI. Report, European Commission,
Brussels.
Kaufman, L. and Rousseeuw, P. J. (1987). Clustering by
means of medoids. In Statistical Data Analysis Based
on the L1-Norm and Related Methods, pages 405–
416. Elsevier Science, Amsterdam, North-Holland;
New York, NY., USA.
Kim, B., Khanna, R., and Koyejo, O. O. (2016). Exam-
ples are not enough, learn to criticize! Criticism for
interpretability. In Advances in Neural Information
Processing Systems 29, pages 2280–2288. Curran As-
sociates, Inc., Barcelona, Spain.
Kulesza, T., Burnett, M., Wong, W.-K., and Stumpf, S.
(2015). Principles of explanatory debugging to per-
sonalize interactive machine learning. In Proceed-
ings of the 20th International Conference on Intelli-
gent User Interfaces, pages 126–137, Atlanta, Geor-
gia, USA. ACM.
Kulesza, T., Stumpf, S., Burnett, M., and Kwan, I. (2012).
Tell me more? the effects of mental model sound-
ness on personalizing an intelligent agent. In Proceed-
ings of the SIGCHI Conference on Human Factors in
Computing Systems, pages 1–10, Austin, Texas, USA.
ACM.
Kulesza, T., Stumpf, S., Burnett, M., Yang, S., Kwan, I.,
and Wong, W.-K. (2013). Too much, too little, or just
right? Ways explanations impact end users’ mental
models. In 2013 IEEE Symposium on Visual Lan-
guages and Human Centric Computing, pages 3–10,
San Jose, CA, USA. IEEE.
Lipton, Z. C. (2018). The mythos of model interpretability:
In machine learning, the concept of interpretability is
both important and slippery. Queue, 16(3):31–57.
Lloyd, S. P. (1982). Least squares quantization in
PCM. IEEE Transactions on Information Theory,
28(2):129–137.
MacQueen, J. (1967). Some methods for classification and
analysis of multivariate observations. In Proceed-
ings of the Fifth Berkeley Symposium on Mathemat-
ical Statistics and Probability, Volume 1: Statistics,
pages 281–297, Berkeley, CA, USA. The Regents of
the University of California.
Meil
˘
a, M. (2003). Comparing clusterings by the varia-
tion of information. In Learning Theory and Kernel
Machines, Lecture Notes in Computer Science, pages
173–187, Berlin, Heidelberg. Springer.
Meil
ˇ
a, M. (2005). Comparing clusterings: An axiomatic
view. In Proceedings of the 22nd International Con-
ference on Machine Learning, pages 577–584, Bonn,
Germany. ACM Press.
Meil
˘
a, M. (2007). Comparing clusterings—an informa-
tion based distance. Journal of Multivariate Analysis,
98(5):873–895.
Meil
˘
a, M. and Heckerman, D. (2001). An experimental
comparison of model-based clustering methods. Ma-
chine Learning, 42(1):9–29.
Miller, T. (2019). Explanation in artificial intelligence: In-
sights from the social sciences. Artificial Intelligence,
267:1–38.
Pahl-Weber, E. and Henckel, D., editors (2008). The
planning system and planning terms in Germany.
Academy for Spatial Research and Planning, Hanover,
DE.
Rand, W. M. (1971). Objective criteria for the evaluation of
clustering methods. Journal of the American Statisti-
cal Association, 66(336):846–850.
Schubert, E. and Rousseeuw, P. J. (2019). Faster k-
medoids clustering: Improving the PAM, CLARA,
and CLARANS algorithms. In Similarity Search and
ICEIS 2023 - 25th International Conference on Enterprise Information Systems
254