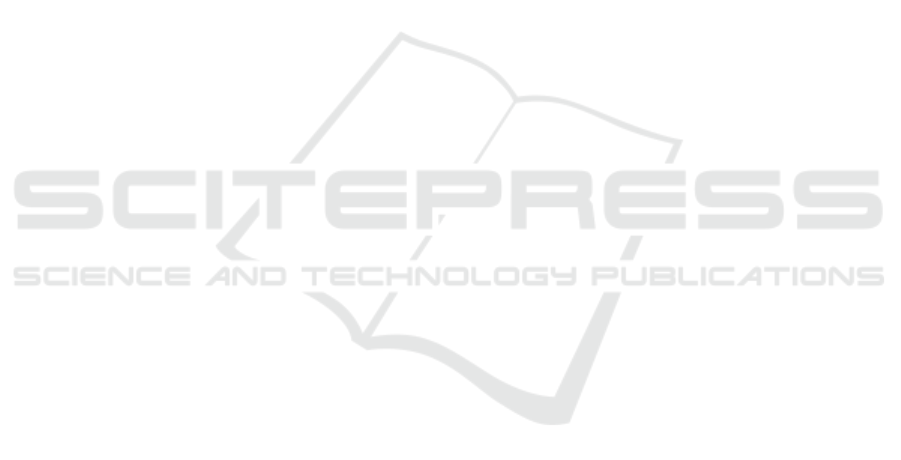
7 CONCLUSIONS
We proposed Sequential Quantum Enhanced Train-
ing (SEQUENT), a two-step transfer learning proce-
dure applied to training hybrid QML algorithms com-
bined with an adapted hybrid architecture to allow
for tracing both the classical and quantum impact on
the overall performance. Furthermore, we showed
the need for said adaptions by formally pointing out
weaknesses of the DQC, the current state-of-the-art
approach to this regard. Finally, we showed that SE-
QUENT yields competitive results for two representa-
tive benchmark datasets compared to DQCs and clas-
sical neural networks. Thus, we provided proof-of-
concept for both the proposed reduced architecture
and the adapted transfer learning training procedure.
However, whilst SEQUENT theoretically is appli-
cable to any kind of VQC, we only considered the
simple architecture with fixed angle embeddings and
δ entangling layers as proposed by (Mari et al., 2020).
Furthermore, we only supplied preliminary experi-
mental implications and did not yet test any high di-
mensional real-world applications. Overall, we do not
expect superior results that outperform state-of-the-
art approaches in the first place, as viable circuit ar-
chitectures for quantum machine learning are still an
active and fast-moving field of research.
Thus, both the real-world applicability and the de-
velopment of circuit architectures that indeed offer
a benefit over classical ones should undergo further
research attention. To empower real-world applica-
tions, the use of hybrid quantum methods should also
be kept in mind when pre-training large classification
models like Resnet. Also, applying more advanced
techniques to train the pre-processing or compression
layer to take full advantage of the chosen quantum
circuit should be examined. Therefore, auto-encoder
architectures might be applicable to train a more gen-
eralized mapping from the classical input-space to the
quantum-space. Overall, we believe, that applying
the proposed concepts and building upon SEQUENT,
both valuable hybrid applications and beneficial quan-
tum circuit architectures can be found.
ACKNOWLEDGEMENTS
This work is part of the Munich Quantum Valley,
which is supported by the Bavarian state govern-
ment with funds from the Hightech Agenda Bayern
Plus and was partially funded by the German BMWK
Project PlanQK (01MK20005I).
REFERENCES
Abohashima, Z., Elhosen, M., Houssein, E. H., and Mo-
hamed, W. M. (2020). Classification with quan-
tum machine learning: A survey. arXiv preprint
arXiv:2006.12270.
Acar, E. and Yilmaz, I. (2021). Covid-19 detection on
ibm quantum computer with classical-quantum trans-
ferlearning. Turkish Journal of Electrical Engineering
and Computer Sciences, 29(1):46–61.
Arute, F., Arya, K., Babbush, R., Bacon, D., Bardin, J. C.,
Barends, R., Biswas, R., Boixo, S., Brandao, F. G.,
Buell, D. A., et al. (2019). Quantum supremacy using
a programmable superconducting processor. Nature,
574(7779):505–510.
Bergholm, V., Izaac, J., Schuld, M., Gogolin, C., Alam,
M. S., Ahmed, S., Arrazola, J. M., Blank, C., Delgado,
A., Jahangiri, S., et al. (2018). Pennylane: Automatic
differentiation of hybrid quantum-classical computa-
tions. arXiv preprint arXiv:1811.04968.
Biamonte, J., Wittek, P., Pancotti, N., Rebentrost, P., Wiebe,
N., and Lloyd, S. (2017). Quantum machine learning.
Nature, 549(7671):195–202.
Bishop, C. M. and Nasrabadi, N. M. (2006). Pattern recog-
nition and machine learning, volume 4. Springer.
Cerezo, M., Arrasmith, A., Babbush, R., Benjamin, S. C.,
Endo, S., Fujii, K., McClean, J. R., Mitarai, K., Yuan,
X., Cincio, L., et al. (2021). Variational quantum al-
gorithms. Nature Reviews Physics, 3(9):625–644.
Donahue, J., Jia, Y., Vinyals, O., Hoffman, J., Zhang, N.,
Tzeng, E., and Darrell, T. (2014). Decaf: A deep con-
volutional activation feature for generic visual recog-
nition. In International conference on machine learn-
ing, pages 647–655. PMLR.
Dong, D., Chen, C., Li, H., and Tarn, T.-J. (2008). Quan-
tum reinforcement learning. IEEE Transactions on
Systems, Man, and Cybernetics, Part B (Cybernetics),
38(5):1207–1220.
Farhi, E., Goldstone, J., and Gutmann, S. (2014). A
quantum approximate optimization algorithm. arXiv
preprint arXiv:1411.4028.
Girshick, R. B., Donahue, J., Darrell, T., and Malik, J.
(2014). Rich feature hierarchies for accurate object
detection and semantic segmentation. 2014 IEEE
Conference on Computer Vision and Pattern Recog-
nition, pages 580–587.
Gokhale, A., Pande, M. B., and Pramod, D. (2020). Im-
plementation of a quantum transfer learning approach
to image splicing detection. International Journal of
Quantum Information, 18(05):2050024.
Grover, L. K. (1996). A fast quantum mechanical algorithm
for database search. In Proceedings of the twenty-
eighth annual ACM symposium on Theory of comput-
ing, pages 212–219.
Havl
´
ı
ˇ
cek, V., C
´
orcoles, A. D., Temme, K., Harrow, A. W.,
Kandala, A., Chow, J. M., and Gambetta, J. M. (2019).
Supervised learning with quantum-enhanced feature
spaces. Nature, 567(7747):209–212.
He, K., Zhang, X., Ren, S., and Sun, J. (2016). Deep resid-
ICAART 2023 - 15th International Conference on Agents and Artificial Intelligence
750