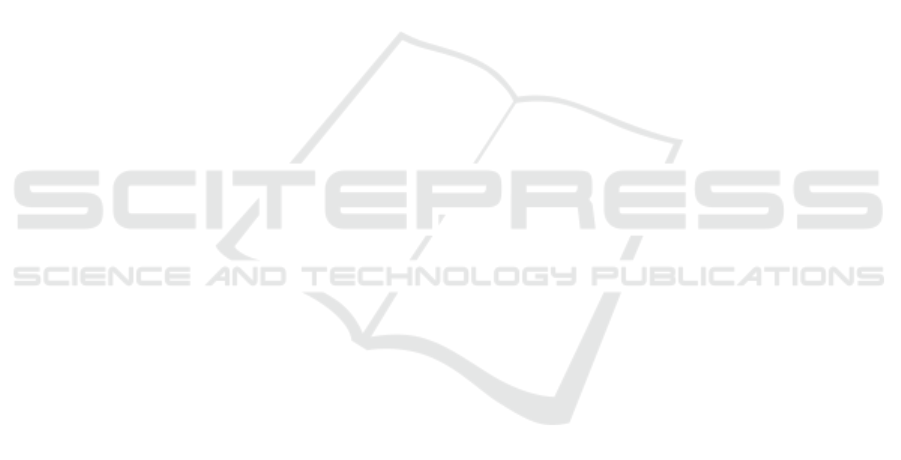
issues reported by the app user while checking if user
complaints have evolved. These two new metrics play
a crucial role in providing a dynamic view of how
users’ opinions evolve, therefore discovering event-
driven trends and life spans of different versions.
The findings obtained by Br-APPS allowed its
stakeholders to direct their development efforts
towards the main issues reported by users. Br-
APPS was able to save them time and effort in
discovering and understanding users’ opinions so that
governments could harness the potential of digital
technology and data to improve outcomes for all.
ACKNOWLEDGMENTS
This work has been supported by Minist
´
erio da
Economia (ME) - Secretaria de Governo Digital
(SGD) Transformac¸
˜
ao Digital de Servic¸os P
´
ublicos
do Governo Brasileiro.
REFERENCES
Blei, D. M., Ng, A. Y., and Jordan, M. I. (2003).
Latent Dirichlet Allocation. The Journal of Machine
Learning Research, 3:993–1022.
Chandy, R. and Gu, H. (2012). Identifying spam in the IOS
app store. WebQuality ’12, page 56–59, New York,
NY, USA. Association for Computing Machinery.
Chen, N., Lin, J., Hoi, S. C. H., Xiao, X., and Zhang,
B. (2014). Ar-miner: Mining informative reviews
for developers from mobile app marketplace. In
Proceedings of the 36th International Conference on
Software Engineering, ICSE 2014, page 767–778,
New York, NY, USA. Association for Computing
Machinery.
Chia, P. H., Yamamoto, Y., and Asokan, N. (2012). Is
this app safe? a large scale study on application
permissions and risk signals. In Proceedings of the
21st International Conference on World Wide Web,
WWW ’12, page 311–320, New York, NY, USA.
Association for Computing Machinery.
Fu, B., Lin, J., Li, L., Faloutsos, C., Hong, J., and Sadeh,
N. (2013). Why people hate your app: Making
sense of user feedback in a mobile app store. KDD
’13, New York, NY, USA. Association for Computing
Machinery.
Iacob, C. and Harrison, R. (2013). Retrieving and analyzing
mobile apps feature requests from online reviews. In
2013 10th Working Conference on Mining Software
Repositories (MSR), pages 41–44.
Li, F., Huang, M., Yang, Y., and Zhu, X. (2011). Learning
to identify review spam. In Proceedings of the Twenty-
Second International Joint Conference on Artificial
Intelligence - Volume Volume Three, IJCAI’11, page
2488–2493. AAAI Press.
Matos, E., B. B. Lanza, B., and D. Lara, R. (2021).
Mobile government in states: Exploratory research
on the development of mobile apps by the brazilian
subnational government. In DG.O2021: The
22nd Annual International Conference on Digital
Government Research, DG.O’21, page 351–362,
New York, NY, USA. Association for Computing
Machinery.
Mikolov, T., Chen, K., Corrado, G., and Dean, J. (2013).
Efficient estimation of word representations in vector
space. arXiv:1301.3781v3.
Minelli, R. and Lanza, M. (2013). Software analytics
for mobile applications–insights & lessons learned.
In 2013 17th European Conference on Software
Maintenance and Reengineering, pages 144–153.
Moody, C. E. (2016). Mixing dirichlet topic
models and word embeddings to make lda2vec.
arXiv:1605.02019.
Mukherjee, A., Liu, B., and Glance, N. (2012). Spotting
fake reviewer groups in consumer reviews. In
Proceedings of the 21st International Conference
on World Wide Web, WWW ’12, page 191–200,
New York, NY, USA. Association for Computing
Machinery.
Pagano, D. and Maalej, W. (2013). User feedback in the
appstore: An empirical study. In 2013 21st IEEE
International Requirements Engineering Conference
(RE), pages 125–134.
Panichella, S., Di Sorbo, A., Guzman, E., Visaggio, C. A.,
Canfora, G., and Gall, H. C. (2015). How can
i improve my app? classifying user reviews for
software maintenance and evolution. In 2015 IEEE
International Conference on Software Maintenance
and Evolution (ICSME), pages 281–290.
Phong, M. V., Nguyen, T. T., Pham, H. V., and Nguyen,
T. T. (2015). Mining user opinions in mobile app
reviews: A keyword-based approach (t). In 2015 30th
IEEE/ACM International Conference on Automated
Software Engineering (ASE), pages 749–759.
Sharma, S. K., Al-Badi, A., Rana, N. P., and Al-Azizi, L.
(2018). Mobile applications in government services
(mg-app) from user’s perspectives: A predictive
modelling approach. Government Information
Quarterly, 35(4):557–568.
Xie, S., Wang, G., Lin, S., and Yu, P. S. (2012). Review
spam detection via temporal pattern discovery. In
Proceedings of the 18th ACM SIGKDD International
Conference on Knowledge Discovery and Data
Mining, KDD ’12, page 823–831, New York, NY,
USA. Association for Computing Machinery.
A User-Centered Approach to Analyze Public Service Apps Based on Reviews
459