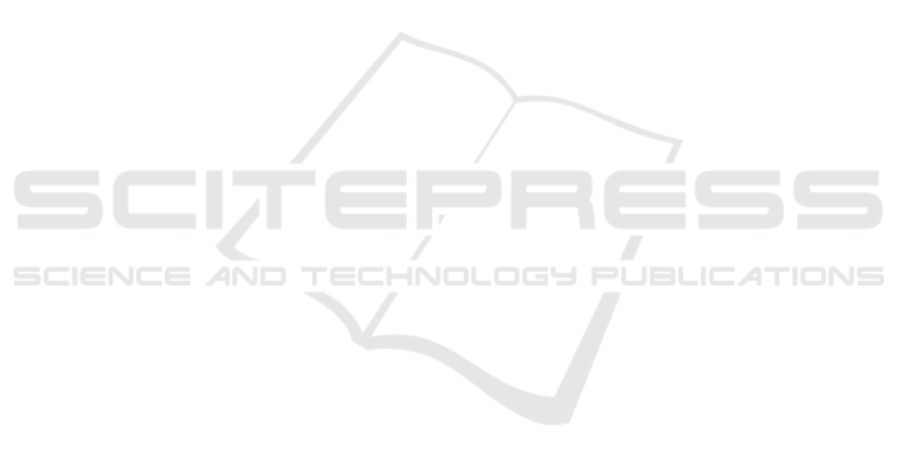
in the Metavision SDK is used. Figure 9 shows some
example images reconstructed with this method.
Evaluation of suitable classification approaches is
part of future work.
4 CONCLUSION & OUTLOOK
In this article, individual steps of a processing
pipeline for long-term monitoring of insects using a
DVS are presented. These steps within the pipeline
are examined using a small dataset. The results of the
tests have shown that a combination of filtering and
density-based clustering is a possibility to label larger
datasets that are needed for a more detailed investiga-
tion. In addition, it was found that neural networks
can be used to segment trajectories. Finally, neural
networks based on polarity images or simulated gray
scale images were favored for insect species classifi-
cation. In order to evaluate the individual steps more
precisely, a larger dataset will be recorded and anno-
tated in the next step.
REFERENCES
Alonso, I. and Murillo, A. C. (2019). EV-SegNet: Seman-
tic Segmentation for Event-Based Cameras. In 2019
IEEE/CVF Conference on Computer Vision and Pat-
tern Recognition Workshops (CVPRW), pages 1624–
1633.
Berger, D. R., Seung, H. S., and Lichtman, J. W. (2018).
VAST (Volume Annotation and Segmentation Tool):
Efficient Manual and Semi-Automatic Labeling of
Large 3D Image Stacks. Frontiers in neural circuits,
12:88.
Binas, J., Neil, D., Liu, S.-C., and Delbruck, T. (2017).
DDD17: End-To-End DAVIS Driving Dataset. In
ICML’17 Workshop on Machine Learning for Au-
tonomous Vehicles (MLAV 2017).
Bolten, T., Lentzen, F., Pohle-Fr
¨
ohlich, R., and T
¨
onnies, K.
(2022). Evaluation of Deep Learning based 3D-Point-
Cloud Processing Techniques for Semantic Segmen-
tation of Neuromorphic Vision Sensor Event-streams.
In Proceedings of the 17th International Joint Con-
ference on Computer Vision, Imaging and Computer
Graphics Theory and Applications - Volume 4: VIS-
APP, pages 168–179. INSTICC, SciTePress.
Bolten, T., Pohle-Fr
¨
ohlich, R., and T
¨
onnies, K. D. (2019).
Application of Hierarchical Clustering for Object
Tracking with a Dynamic Vision Sensor. In Ro-
drigues, J. e. a., editor, Computational Science – ICCS
2019, volume 11540 of Lecture Notes in Computer
Science, pages 164–176, Cham. Springer.
Bolten, T., Pohle-Fr
¨
ohlich, R., and T
¨
onnies, K. D. (2021).
DVS-OUTLAB: A Neuromorphic Event-Based Long
Time Monitoring Dataset for Real-World Outdoor
Scenarios. In Proceedings of the IEEE/CVF Con-
ference on Computer Vision and Pattern Recognition
(CVPR) Workshops, pages 1348–1357.
de Tournemire, P., Nitti, D., Perot, E., Migliore, D., and
Sironi, A. (2020). A Large Scale Event-based Detec-
tion Dataset for Automotive. arXiv, abs/2001.08499.
Ester, M., Kriegel, H.-P., Sander, J., Xu, X., et al. (1996). A
Density-Based Algorithm for Discovering Clusters in
Large Spatial Databases with Noise. In Proceedings
of the Second International Conference on Knowledge
Discovery and Data Mining, pages 226–231.
Gallego, G., Delbr
¨
uck, T., Orchard, G., Bartolozzi, C.,
Taba, B., Censi, A., Leutenegger, S., Davison, A. J.,
Conradt, J., Daniilidis, K., and Scaramuzza, D.
(2022). Event-Based Vision: A Survey. IEEE Trans-
actions on Pattern Analysis and Machine Intelligence,
44(1):154–180.
Greenewalt, C. H. (1962). Dimensional relationships for
flying animals. Smithsonian miscellaneous collec-
tions.
Hackel, T., Wegner, J. D., and Schindler, K. (2016). Con-
tour Detection in Unstructured 3D Point Clouds. In
Proceedings of the IEEE conference on computer vi-
sion and pattern recognition, pages 1610–1618.
Hallmann, C. A., Sorg, M., Jongejans, E., Siepel, H.,
Hofland, N., Schwan, H., Stenmans, W., M
¨
uller,
A., Sumser, H., H
¨
orren, T., et al. (2017). More
than 75 percent decline over 27 years in total fly-
ing insect biomass in protected areas. PloS one,
12(10):e0185809.
Han, J., Yang, Y., Zhou, C., Xu, C., and Shi, B. (2021).
EvIntSR-Net: Event Guided Multiple Latent Frames
Reconstruction and Super-resolution. In Proceedings
of the IEEE/CVF International Conference on Com-
puter Vision, pages 4882–4891.
Hu, Y., Binas, J., Neil, D., Liu, S.-C., and Delbruck, T.
(2020). DDD20 End-to-End Event Camera Driving
Dataset: Fusing Frames and Events with Deep Learn-
ing for Improved Steering Prediction. In 2020 IEEE
23rd International Conference on Intelligent Trans-
portation Systems (ITSC), pages 1–6.
Qi, C. R., Yi, L., Su, H., and Guibas, L. J. (2017). Point-
Net++: Deep Hierarchical Feature Learning on Point
Sets in a Metric Space. Advances in neural informa-
tion processing systems, 30.
Rodr
´
ıguez-Gomez, J., Egu
´
ıluz, A. G., Mart
´
ınez-de Dios,
J., and Ollero, A. (2020). Asynchronous event-based
clustering and tracking for intrusion monitoring in
UAS. In 2020 IEEE International Conference on
Robotics and Automation (ICRA), pages 8518–8524.
Ronneberger, O., Fischer, P., and Brox, T. (2015). U-Net:
Convolutional Networks for Biomedical Image Seg-
mentation. In International Conference on Medical
image computing and computer-assisted intervention,
pages 234–241. Springer.
W
¨
agele, J. W., Bodesheim, P., Bourlat, S. J., Denzler,
J., Diepenbroek, M., Fonseca, V., Frommolt, K.-H.,
Geiger, M. F., Gemeinholzer, B., Gl
¨
ockner, F. O., et al.
(2022). Towards a multisensor station for automated
biodiversity monitoring. Basic and Applied Ecology,
59:105–138.
Concept Study for Dynamic Vision Sensor Based Insect Monitoring
417