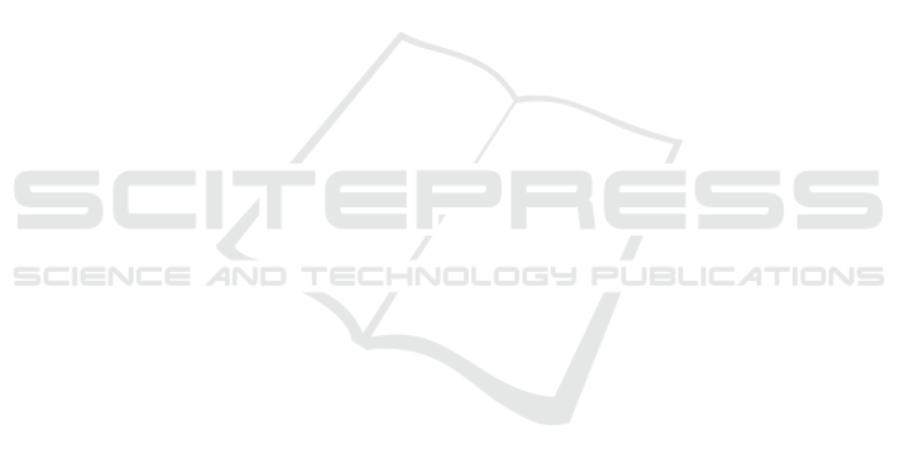
the rectangular shape of the original B-Scans.
Evaluation was done on the basis of Dice Score
which is a standard method of evaluating segmenta-
tion problems. Experimental results show that the
proposed model outperformed both the human ex-
perts’ annotation and the current state-of-the-art ar-
chitectures by a clear margin, even on a very small,
imbalanced and complex dataset with a high degree
of presence of pathology that severely affects the nor-
mal morphology of the retina.
The dataset was collected for 2 problems (layers
and fluid segmentation) which can be experimented
separately but we decided to do both jointly together
which is a more challenging task.
The CoNet can be directly applied to solve real
world problems and to monitor the progress of eye
diseases such as diabetic macular edema (DME), age-
related macular degeneration (AMD) and Glaucoma.
In the future we will evaluate the CoNet on other
benchmark datasets, and compare our results to other
state-of-the-art models. Also we plan to extend the
current 2D network to 3D.
REFERENCES
Abr
`
amoff, M. D., Garvin, M. K., and Sonka, M. (2010).
Retinal imaging and image analysis. IEEE reviews in
biomedical engineering, 3:169–208.
Bogunovi
´
c, H., Venhuizen, F., Klimscha, S., Apostolopou-
los, S., Bab-Hadiashar, A., Bagci, U., Beg, M. F.,
Bekalo, L., Chen, Q., Ciller, C., et al. (2019). Re-
touch: the retinal oct fluid detection and segmentation
benchmark and challenge. IEEE transactions on med-
ical imaging, 38(8):1858–1874.
Bresnick, G. H. (1986). Diabetic macular edema: a review.
Ophthalmology, 93(7):989–997.
Chen, L.-C., Papandreou, G., Kokkinos, I., Murphy, K., and
Yuille, A. L. (2017). Deeplab: Semantic image seg-
mentation with deep convolutional nets, atrous convo-
lution, and fully connected crfs. IEEE transactions on
pattern analysis and machine intelligence, 40(4):834–
848.
Chiu, S., Allingham, M., Mettu, P., Cousins, S., Izatt, J., and
Farsiu, S. (2015). Kernel regression based segmen-
tation of optical coherence tomography images with
diabetic macular edema. Biomedical Optics Express,
6.
Cicek, O., Abdulkadir, A., Lienkamp, S., Brox, T., and Ron-
neberger, O. (2016). 3D U-Net: Learning dense volu-
metric segmentation from sparse annotation.
Dodo, B. I., Li, Y., Kaba, D., and Liu, X. (2019a). Retinal
layer segmentation in optical coherence tomography
images. IEEE Access, 7:152388–152398.
Dodo, B. I., Li, Y., and Liu, X. (2019b). Level set segmenta-
tion of retinal oct images. In International Conference
on Bioimaging. Czech Republic.
Fang, L., Cunefare, D., Wang, C., Guymer, R. H., Li, S.,
and Farsiu, S. (2017). Automatic segmentation of
nine retinal layer boundaries in oct images of non-
exudative amd patients using deep learning and graph
search. Biomedical optics express, 8(5):2732–2744.
Fauw, J., Ledsam, J., Romera-Paredes, B., Nikolov, S.,
Tomasev, N., Blackwell, S., Askham, H., Glorot, X.,
ODonoghue, B., Visentin, D., Driessche, G., Lak-
shminarayanan, B., Meyer, C., Mackinder, F., Bou-
ton, S., Ayoub, K., Chopra, R., King, D., Karthike-
salingam, A., and Ronneberger, O. (2018). Clinically
applicable deep learning for diagnosis and referral in
retinal disease. Nature Medicine, 24.
Fernandez, D. C. (2005). Delineating fluid-filled region
boundaries in optical coherence tomography images
of the retina. IEEE transactions on medical imaging,
24(8):929–945.
Garvin, M. K., Abramoff, M. D., Wu, X., Russell, S. R.,
Burns, T. L., and Sonka, M. (2009). Automated 3-
d intraretinal layer segmentation of macular spectral-
domain optical coherence tomography images. IEEE
transactions on medical imaging, 28(9):1436–1447.
Girish, G., Thakur, B., Chowdhury, S. R., Kothari, A. R.,
and Rajan, J. (2018). Segmentation of intra-retinal
cysts from optical coherence tomography images
using a fully convolutional neural network model.
IEEE journal of biomedical and health informatics,
23(1):296–304.
Gopinath, K. and Sivaswamy, J. (2018). Segmentation of
retinal cysts from optical coherence tomography vol-
umes via selective enhancement. IEEE journal of
biomedical and health informatics, 23(1):273–282.
Huang, D., Swanson, E. A., Lin, C. P., Schuman, J. S., Stin-
son, W. G., Chang, W., Hee, M. R., Flotte, T., Gregory,
K., Puliafito, C. A., et al. (1991). Optical coherence
tomography. science, 254(5035):1178–1181.
Kaba, D., Salazar-Gonzalez, A. G., Li, Y., Liu, X., and
Serag, A. (2013). Segmentation of retinal blood ves-
sels using gaussian mixture models and expectation
maximisation. In International Conference on Health
Information Science, pages 105–112.
Kaba, D., Wang, C., Li, Y., Salazar-Gonzalez, A., Liu, X.,
and Serag, A. (2014). Retinal blood vessels extrac-
tion using probabilistic modelling. Health Informa-
tion Science and Systems, 2(1):2.
Kaba, D., Wang, Y., Wang, C., Liu, X., Zhu, H., Salazar-
Gonzalez, A. G., and Li., Y. (2015). Retina layer
segmentation using kernel graph cuts and continuous
max-flow. Optics Express, 23(6):7366–7384.
Kles, J. B. M.-K. A. and Anderson, P. W. (2007). How the
diabetic eye loses vision.
Lee, C. S., Tyring, A. J., Deruyter, N. P., Wu, Y., Rokem,
A., and Lee, A. Y. (2017). Deep-learning based, au-
tomated segmentation of macular edema in optical
coherence tomography. Biomedical optics express,
8(7):3440–3448.
Loo, J., Fang, L., Cunefare, D., Jaffe, G. J., and Farsiu, S.
(2018). Deep longitudinal transfer learning-based au-
tomatic segmentation of photoreceptor ellipsoid zone
defects on optical coherence tomography images of
BIOIMAGING 2023 - 10th International Conference on Bioimaging
136