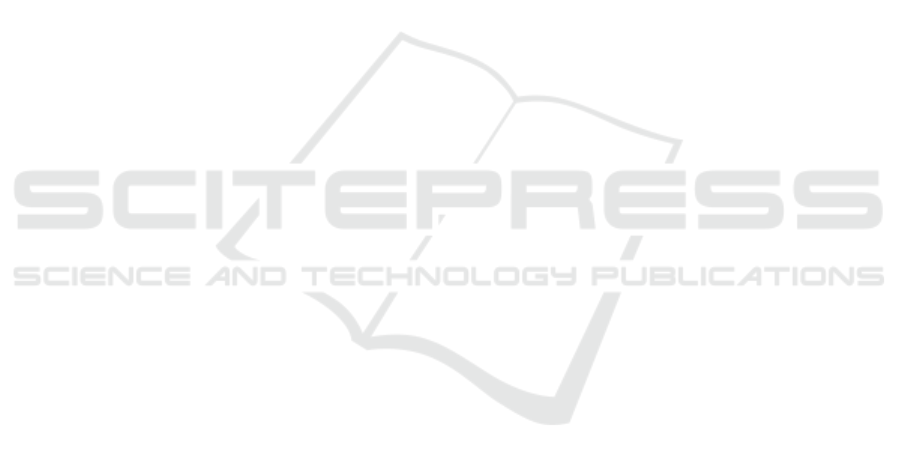
ropean General Data Protection Regulation (GDPR)
(European Parliament and Council of the European
Union, 2016), the Chinese Provisions on the Manage-
ment of Automotive Data Security (PMADS) (Pro-
visions, 2022), and the American California Con-
sumer Privacy Act (CCPA) (California State Legis-
lature, 2018).
The paper is structured as follows: Section 2 de-
scribes the related work, while Section 3 is the legal
background about privacy regulations. Section 4 is
the readability analysis of the privacy policies using
four different indexes. Section 5 contains an inves-
tigation of the policies concerning Articles 9 and 10
of the GDPR, which defines some special categories
of data that should be treated properly. Section 6 de-
scribes the dynamic dashboard with the comparison
among the carmakers’ privacy policies. Section 7 re-
ports the findings and possible future works.
2 RELATED WORK
In recent years, attention to privacy risk perception
and the analysis of privacy policies have been raised.
In (Fabian et al., 2017), the authors present a
large-scale study on the readability of nearly 50,000
privacy policies of English websites. From this work,
we inherit some of the applied readability indexes, but
our analysis is limited only to the automotive privacy
policies. Another significant work (Lawson et al.,
2015) on the privacy of connected vehicles was re-
leased in 2015, when the Canadian Freedom of Infor-
mation and Privacy Association (FIPA) wrote a year-
long study on privacy, consumer choice, and vehicle
technology. This work is a complete document with a
detailed description of every aspect and concern about
emerging connected vehicles, but, it is mainly focused
on the Canadian audience and it does not deal directly
with the privacy policies documents. However, this
guide provides a specific indication for our work, be-
cause it states that, during a purchasing decision, “it
would remain unrealistic to expect the average car
purchaser to be able to review and compare the pri-
vacy policies of various carmakers, dealers and other
relevant service providers.”. Our work can address
this issue because the dashboard compares the pri-
vacy policies of different carmakers. Another valu-
able work in the automotive field is the Pes
´
e survey
(Pes
´
e, 2019), where authors describe the automotive
privacy attacks and define a privacy score, quantify-
ing the risk associated with each vehicular sensor and
the related attack, but without directly analyzing the
privacy policies. Another relevant work is (Zaeem
et al., 2020), which states that privacy policies can
be lengthy and hard to comprehend. To address this
problem, researchers have utilized machine learning
to devise tools that automatically summarize online
privacy policies for web pages. In our work, with the
reading analysis, we verify the assumption that the
documents could be challenging to understand, and
we provide an intuitive instrument to compare car-
makers’ privacy policies and show the differences.
Regarding the comparison among the privacy reg-
ulation in automotive, significant work is (Michael
Tan and Thomas Kahl, 2022), where authors compare
PMADS and the GDPR with a specific focus on the
automotive industry. In our work, we add the CCPA
to consider also another significant area for the auto-
motive industry like the California and USA.
3 LEGAL BACKGROUND
Even if we do not address directly the legal aspects
of data collection, we need to identify some legal re-
quirements and possible constraints which can help us
to compare the privacy policies. We choose the Euro-
pean GDPR, the Chinese PMADS, and the American
CCPA, which, can be considered three of the most
significant legal documents for the protection of per-
sonal data in automotive.
The GDPR, effective in May 2018, is a legal
framework that sets guidelines for the collection and
processing of personal information for companies
and organizations that handle information of Euro-
pean Union (EU) citizens. The EU has drafted an-
other document that can be used to regulate data pri-
vacy: the more automotive-related Guidelines 1/2020
(EDPB, 2020) on processing personal data in the con-
text of connected vehicles and mobility-related ap-
plications, written by the European Data Protection
Board and published in early 2020. In particular, the
guidelines define the connected vehicles as “terminal
equipment” just like a computer, a smartphone, or a
smart TV and identify three special categories of data:
location, biometrics, and offenses.
The PMADS is a Chinese regulation that was is-
sued on August 2021 and entered into force on Octo-
ber 2021. It aims to regulate vehicle data processing
activities to protect the rights and interests of individ-
uals and organizations. It distinguishes between per-
sonal data, which includes any information that could
infer a person’s identity or behavior, and important
data, which includes data that may endanger national
security, for example, in military areas.
The other relevant regulation is the CCPA, which
is an advanced state statute to protect privacy rights
in California State and contains the broadest defini-
Vehicle Data Collection: A Privacy Policy Analysis and Comparison
627