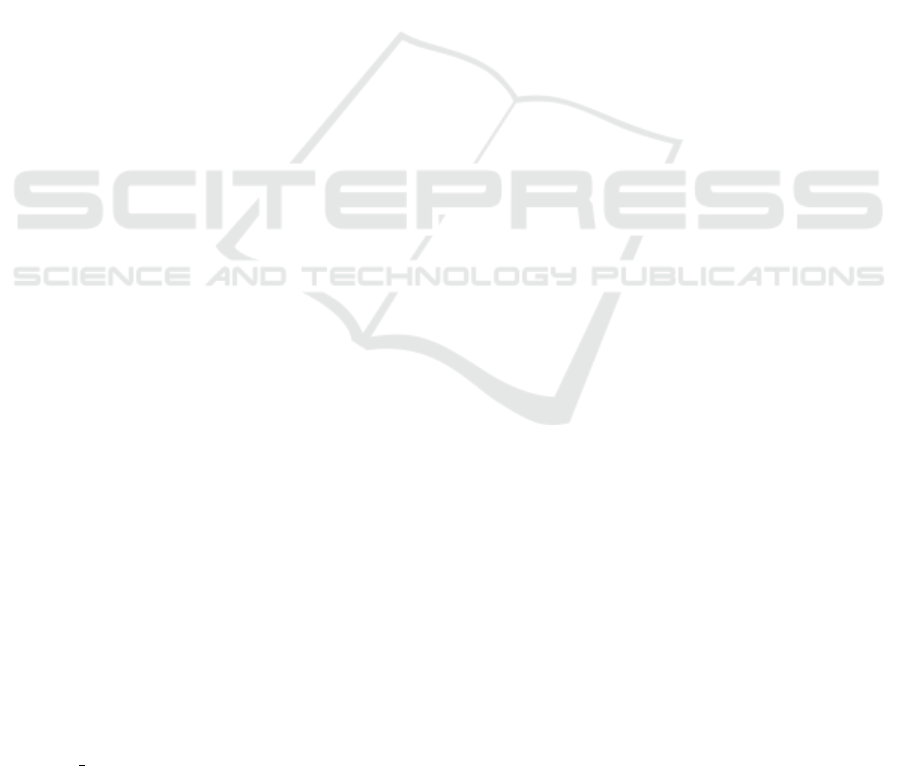
authors reviewed the manuscript. All authors read and
approved the final manuscript.
ACKNOWLEDGEMENTS
A special thanks to Rogia Kpanou for her inputs in
this work. We also acknowledge the support of Com-
pute Canada for providing additional computational
support and also Dr Jacques Corbeil’s Canada Re-
search Chair in Medical Genomics.
REFERENCES
Akiba, T., Sano, S., Yanase, T., Ohta, T., and Koyama, M.
(2019). Optuna: A next-generation hyperparameter
optimization framework. In Proceedings of the 25rd
ACM SIGKDD International Conference on Knowl-
edge Discovery and Data Mining.
Arnedos, M., Vicier, C., Loi, S., Lefebvre, C., Michiels,
S., Bonnefoi, H., and Andre, F. (2015). Precision
medicine for metastatic breast cancer—limitations
and solutions. Nature reviews Clinical oncology,
12(12):693–704.
Ayan, E. and
¨
Unver, H. M. (2018). Data augmentation
importance for classification of skin lesions via deep
learning. In 2018 Electric Electronics, Computer
Science, Biomedical Engineerings’ Meeting (EBBT),
pages 1–4. IEEE.
Beger, R. D. (2013). A review of applications of
metabolomics in cancer. Metabolites, 3(3):552–574.
Bengio, Y. (2009). Learning deep architectures for AI. Now
Publishers Inc.
Bersanelli, M., Mosca, E., Remondini, D., Giampieri,
E., Sala, C., Castellani, G., and Milanesi, L.
(2016). Methods for the integration of multi-omics
data: mathematical aspects. BMC bioinformatics,
17(2):167–177.
Borad, M. J. and LoRusso, P. M. (2017). Twenty-first cen-
tury precision medicine in oncology: genomic profil-
ing in patients with cancer. In Mayo Clinic Proceed-
ings, volume 92, pages 1583–1591. Elsevier.
Buitinck, L., Louppe, G., Blondel, M., Pedregosa, F.,
Mueller, A., Grisel, O., Niculae, V., Prettenhofer, P.,
Gramfort, A., Grobler, J., Layton, R., VanderPlas, J.,
Joly, A., Holt, B., and Varoquaux, G. (2013). API de-
sign for machine learning software: experiences from
the scikit-learn project. In ECML PKDD Workshop:
Languages for Data Mining and Machine Learning,
pages 108–122.
Cao, K., Bai, X., Hong, Y., and Wan, L. (2020).
Unsupervised topological alignment for single-cell
multi-omics integration. Bioinformatics, 36(Supple-
ment 1):i48–i56.
Chaudhary, K., Poirion, O. B., Lu, L., and Garmire, L. X.
(2018). Deep learning–based multi-omics integration
robustly predicts survival in liver cancer. Clinical
Cancer Research, 24(6):1248–1259.
Coller, H. A. (2014). Is cancer a metabolic disease? The
American Journal of Pathology, 184(1):4–17.
Dias-Audibert, F. L., Navarro, L. C., de Oliveira, D. N., De-
lafiori, J., Melo, C. F. O. R., Guerreiro, T. M., Rosa,
F. T., Petenuci, D. L., Watanabe, M. A. E., Velloso,
L. A., et al. (2020). Combining machine learning
and metabolomics to identify weight gain biomarkers.
Frontiers in bioengineering and biotechnology, 8:6.
Garali, I., Adanyeguh, I. M., Ichou, F., Perlbarg, V., Seyer,
A., Colsch, B., Moszer, I., Guillemot, V., Durr, A.,
Mochel, F., et al. (2018). A strategy for multimodal
data integration: application to biomarkers identifica-
tion in spinocerebellar ataxia. Briefings in bioinfor-
matics, 19(6):1356–1369.
Goldman, M. J., Craft, B., Hastie, M., Repe
ˇ
cka, K., Mc-
Dade, F., Kamath, A., Banerjee, A., Luo, Y., Rogers,
D., Brooks, A. N., et al. (2020). Visualizing and in-
terpreting cancer genomics data via the xena platform.
Nature biotechnology, 38(6):675–678.
Haas, R., Zelezniak, A., Iacovacci, J., Kamrad, S.,
Townsend, S., and Ralser, M. (2017). Designing and
interpreting ‘multi-omic’experiments that may change
our understanding of biology. Current Opinion in Sys-
tems Biology, 6:37–45.
Khan, A. and Lee, B. (2021). Gene transformer: Transform-
ers for the gene expression-based classification of lung
cancer subtypes. arXiv preprint arXiv:2108.11833.
Kim, M. and Tagkopoulos, I. (2018). Data integration
and predictive modeling methods for multi-omics
datasets. Molecular omics, 14(1):8–25.
Kingma, D. P. and Welling, M. (2013). Auto-encoding vari-
ational bayes. arXiv preprint arXiv:1312.6114.
Kothari, C., Osseni, M. A., Agbo, L., Ouellette, G.,
D
´
eraspe, M., Laviolette, F., Corbeil, J., Lambert, J.-P.,
Diorio, C., and Durocher, F. (2020). Machine learning
analysis identifies genes differentiating triple negative
breast cancers. Scientific reports, 10(1):1–15.
Li, H., Siddiqui, O., Zhang, H., and Guan, Y. (2019).
Joint learning improves protein abundance prediction
in cancers. BMC biology, 17(1):1–14.
Lima, A. R., de Lourdes Bastos, M., Carvalho, M., and
de Pinho, P. G. (2016). Biomarker discovery in hu-
man prostate cancer: an update in metabolomics stud-
ies. Translational oncology, 9(4):357–370.
Lipinski, K. A., Barber, L. J., Davies, M. N., Ashenden,
M., Sottoriva, A., and Gerlinger, M. (2016). Cancer
evolution and the limits of predictability in precision
cancer medicine. Trends in cancer, 2(1):49–63.
Ma, A., McDermaid, A., Xu, J., Chang, Y., and Ma, Q.
(2020). Integrative methods and practical challenges
for single-cell multi-omics. Trends in Biotechnology.
Mamoshina, P., Volosnikova, M., Ozerov, I. V., Putin, E.,
Skibina, E., Cortese, F., and Zhavoronkov, A. (2018).
Machine learning on human muscle transcriptomic
data for biomarker discovery and tissue-specific drug
target identification. Frontiers in genetics, 9:242.
Masci, J., Meier, U., Cires¸an, D., and Schmidhuber, J.
(2011). Stacked convolutional auto-encoders for hi-
MOT: A Multi-Omics Transformer for Multiclass Classification Tumour Types Predictions
259