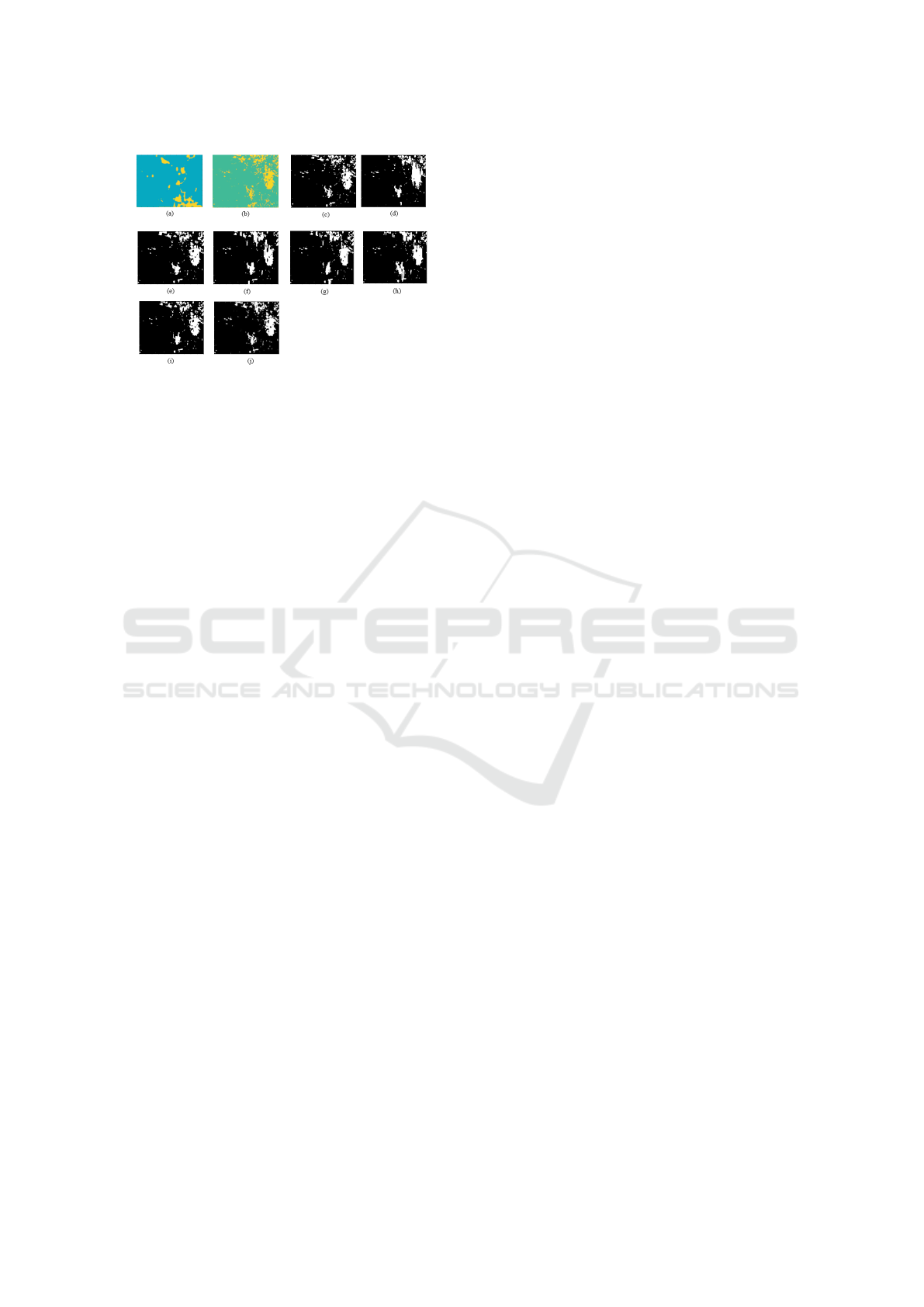
Figure 9: Change detection map results of California data:
(a), (b), (c), (d), (e), (f), (g), (h), (i), (j) represent respec-
tively the first image, the second image, the ground truth,
the results of the change detection of (Shi et al., 2021),
(Brahim et al., 2021), (Zhang et al., 2020), (Dong et al.,
2020), and (Chen et al., 2022), the method Shearlet+CNN,
and the proposed method. Where white and black pixels are
respectively (Changed, Unchanged).
Where,TP, TN, FP and FN represent respectively
true-positive, true-negative, false-positive and false-
negative (Brahim et al., 2021). The data results are
presented in Table 1 and the TP, TN, FP, FN are illus-
trated in Table 2.
In table 1, the difference values between the ac-
curacy result given by our method and other existing
methods in the first data are equal to 4.51%, 0.18%,
1.76%, 0.03%, 1.41%, and 5.2%, respectively. How-
ever, in the second data, the difference values are
respectively equal to 2.52%, 0.29%, 1.7%, 0.05%,
1.39%, and 6.74%. As regards the third data, the
difference values are respectively equal to 3.99%,
0.44%, 0.04%, 2.74%, 1.49%, and 3.75%. Using Ta-
ble 1, we conclude that the value of the difference be-
tween the accuracy result given by the two methods
DSAMNET (Shi et al., 2021), MSF-Net (Chen et al.,
2022), and the proposed method in each data is im-
portant because in the two works (Shi et al., 2021) and
(Chen et al., 2022) the input images were not prepro-
cessed so the result of change detection was not bet-
ter. For the two methods based on Shearlet+DI+CNN
(Brahim et al., 2021) and Shearlet+CNN, the value
of the difference between the accuracy result given
by the two methods and the proposed method was
low. However, in the first method based on Shear-
let+DI+CNN (Brahim et al., 2021), the input data of
the CNN were the subtraction of the two smoothed
images using the Shearlet transform. In this shearlet,
the authors used the OTSU to classify all the coeffi-
cients but in the second method, Shearlet+CNN, we
utilized K-means to classify all the coefficients with-
out losing the data and we generated the subtraction
of the features for more convolutional layers of CNN.
When the number of convolutional layers increases,
the features become more detailed. So the result ac-
curacy is increased compared to the result of Shear-
let+DI+CNN (Brahim et al., 2021). The following
Recall results obtained in the first and second data of
MSF-Net (Chen et al., 2022) and DSAMNET (Shi
et al., 2021) were low compared to the other meth-
ods and the proposed method with a value difference
respectively equal to 5.17%, 0.34%, 0.89%, 0.18%,
0.67%, and 5.19% in the first data. In the second
data, the value difference was respectively equal to
1.46%, 0.19%, 1.067%, 0.07%,0.85%, and 2.46%. In
the third data, the following Recall results of Shear-
let+DI+CNN (Brahim et al., 2021) was better com-
pared to the proposed method because in the Shear-
let+DI+CNN method (Brahim et al., 2021) the clas-
sifier of the coefficients in shearlet is OTSU so the
data change can be lost. Then, The result of Shear-
let+DI+CNN (Brahim et al., 2021) increased com-
pared to the proposed method with a value equal to
0.4%, which is not a very important difference. The
F1-score result in the first data, the second data and
the third data was low compared to the proposed
method. Obviously, the results improved the values,
which were respectively equal, in the first data, to
5.05%, 0.17%, 0.9%, 0.01%, 0.44%, and 5.78%. In
the second data, the difference values were respec-
tively equal to 1.55%, 0.17%, 1.06%, 0.037%, 0.85%,
and 2.83%. However, in the third data, they were
equal to 17.05%, 2.59%,10.88%, 1.23%, 7.2%, and
15.58%. The accuracy result and the F1-score re-
sult of the proposed method increased compared to
the result of method Shearlet+CNN. Therefore, The
Resnet152 network played an important role in the
change detection. Table 1 shows that, in each data, the
accuracy result of the proposed method was consider-
ably higher compared to that of the other methods.
In Table 2, the result of FN of the proposed
method in the first data decreased compared to other
methods. For example, the difference value pro-
vided by the method Shearlet+DI+CNN (Brahim et
al., 2021) and the introduced method was equal to
0.35% in the first data, and equal to 0.19%, in the
second data. However, in the third data, the FN re-
sult increased, because the Recall result of the Shear-
let+DI+CNN method (Brahim et al., 2021) was su-
perior to the Recall result of the proposed method.
Consequently, the TP result of the Shearlet+DI+CNN
method (Brahim et al., 2021) was important compared
to the result of the proposed method. The degrada-
tion value was not important so the accuracy value
of the proposed method was better. Using the pro-
posed method, the FP result in three data was better
and increased compared to the result of the proposed
VISAPP 2023 - 18th International Conference on Computer Vision Theory and Applications
432