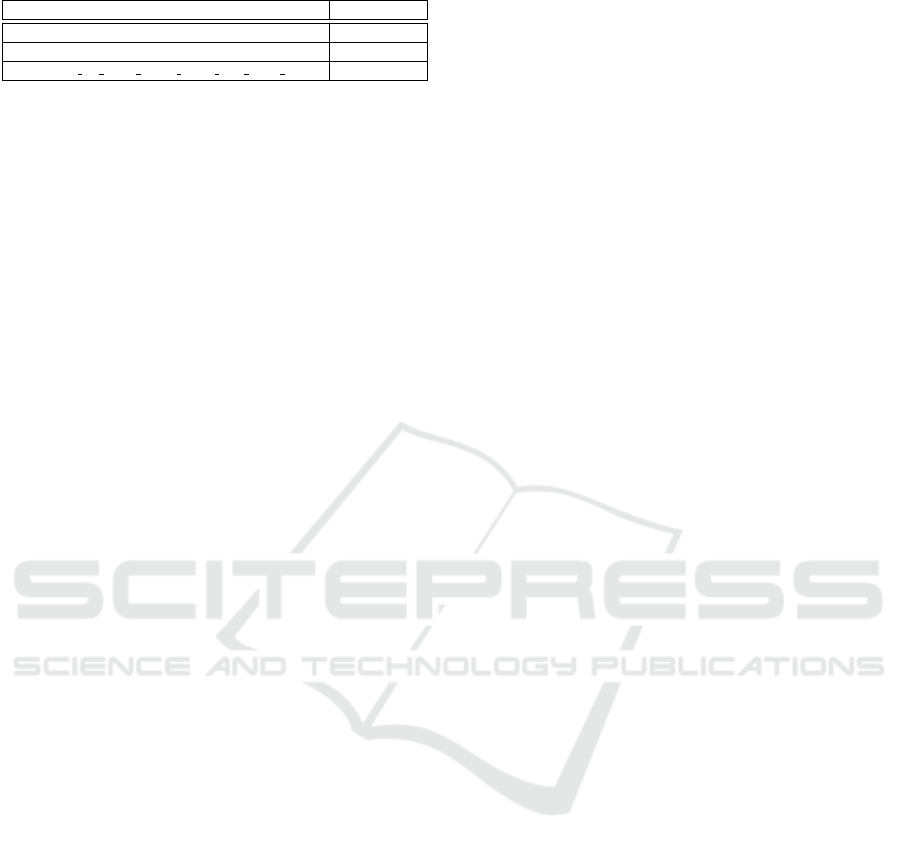
Table 3: Results of TabooLM Based on Various Models.
Model Games Won
facebook/bart-large-mnli 27
bart-large-mnli-yahoo-answers 38
DeBERTa v3 large mnli- fever anli ling- wanli 53
7 CONCLUSION
We have shown TabooLM, a system that takes queries
in a city-hint format and utilizes language models in
a zero-shot setting to return ranked lists of cities im-
plied by the given hints. Given a list of hints, it it-
erates from top to bottom and matches those hints
with popular cities worldwide. Although it was built
explicitly for the Taboo challenge competition, the
system can be used with any task involving a word-
guessing problem.
Compared to previous work, the results provide
convincing evidence that our system can achieve
state-of-the-art results. In this regard, the results
suggest that solutions utilizing language models in
a zero-shot setting can be used to tackle challenging
NLP tasks. However, given that the computational in-
nards of these kinds of models are complex, further
gains could be achieved via transparent solutions that
employ additional semantic analysis of city-hint pairs.
Future studies could blend both modern and clas-
sic AI in order to build transparent hybrid solutions.
Among possible directions, systems that construct the
building of knowledge graphs from language models
could offer a better solution.
REFERENCES
Brown, T., Mann, B., Ryder, N., Subbiah, M., Kaplan, J. D.,
Dhariwal, P., Neelakantan, A., Shyam, P., Sastry, G.,
Askell, A., et al. (2020). Language models are few-
shot learners. Advances in neural information pro-
cessing systems, 33:1877–1901.
Chowdhery, A., Narang, S., Devlin, J., Bosma, M., Mishra,
G., Roberts, A., Barham, P., Chung, H. W., Sut-
ton, C., Gehrmann, S., et al. (2022). Palm: Scal-
ing language modeling with pathways. arXiv preprint
arXiv:2204.02311.
Dankers, V., Bilgin, A., and Fern
´
andez, R. (2017). Mod-
elling word associations with word embeddings for a
guesser agent in the taboo city challenge competition.
Devlin, J., Chang, M.-W., Lee, K., and Toutanova, K.
(2018). Bert: Pre-training of deep bidirectional trans-
formers for language understanding. arXiv preprint
arXiv:1810.04805.
He, P., Gao, J., and Chen, W. (2021). Debertav3: Improving
deberta using electra-style pre-training with gradient-
disentangled embedding sharing.
He, P., Liu, X., Gao, J., and Chen, W. (2020). Deberta:
Decoding-enhanced bert with disentangled attention.
Howard, J. and Ruder, S. (2018). Universal language model
fine-tuning for text classification.
Isaak, N. and Michael, L. (2016). Tackling the Winograd
Schema Challenge Through Machine Logical Infer-
ences. In Pearce, D. and Pinto, H. S., editors, STAIRS,
volume 284 of Frontiers in Artificial Intelligence and
Applications, pages 75–86. IOS Press.
Isaak, N. and Michael, L. (2017a). How the Availability of
Training Material Affects Performance in the Wino-
grad Schema Challenge. In Proceedings of the (IJCAI
2017) 3rd Workshop on Cognitive Knowledge Acqui-
sition and Applications (Cognitum 2017).
Isaak, N. and Michael, L. (2017b). Tackling the taboo chal-
lenge with machine logical inferences. page 46.
Koksal, A. (2017). Skip-gram model for simulation of taboo
game.
Laurer, M., van Atteveldt, W., Casas, A., and Welbers, K.
(2022). Less annotating, more classifying–addressing
the data scarcity issue of supervised machine learning
with deep transfer learning and bert-nli.
Liu, Y., Ott, M., Goyal, N., Du, J., Joshi, M., Chen, D.,
Levy, O., Lewis, M., Zettlemoyer, L., and Stoyanov,
V. (2019). Roberta: A robustly optimized bert pre-
training approach. arXiv preprint arXiv:1907.11692.
MacCartney, B. (2009). Natural language inference. Stan-
ford University.
McCarthy, J., Minsky, M. L., Rochester, N., and Shannon,
C. E. (2006). A Proposal for the Dartmouth Summer
Research Project on Artificial Intelligence, August 31,
1955. AI magazine, 27(4):12–12.
Michael, L. (2013). Machines with Websense. In Proc. of
11th International Symposium on Logical Formaliza-
tions of Commonsense Reasoning (Commonsense 13).
Peters, M. E., Neumann, M., Iyyer, M., Gardner, M., Clark,
C., Lee, K., and Zettlemoyer, L. (2018). Deep contex-
tualized word representations.
Radford, A., Wu, J., Child, R., Luan, D., Amodei, D., and
Sutskever, I. (2019). Language models are unsuper-
vised multitask learners.
Romera-Paredes, B. and Torr, P. (2015). An embarrassingly
simple approach to zero-shot learning. In Bach, F.
and Blei, D., editors, Proceedings of the 32nd Inter-
national Conference on Machine Learning, volume 37
of Proceedings of Machine Learning Research, pages
2152–2161, Lille, France. PMLR.
Rovatsos, M., Gromann, D., and Bella, G. (2018).
The Taboo Challenge Competition. AI Magazine,
39(1):84–87.
Wang, C., Liu, X., and Song, D. (2020). Language models
are open knowledge graphs.
Wei, J., Bosma, M., Zhao, V. Y., Guu, K., Yu, A. W., Lester,
B., Du, N., Dai, A. M., and Le, Q. V. (2021). Fine-
tuned language models are zero-shot learners.
Xian, Y., Schiele, B., and Akata, Z. (2017). Zero-shot
learning-the good, the bad and the ugly. In Proceed-
ings of the IEEE conference on computer vision and
pattern recognition, pages 4582–4591.
ICAART 2023 - 15th International Conference on Agents and Artificial Intelligence
828