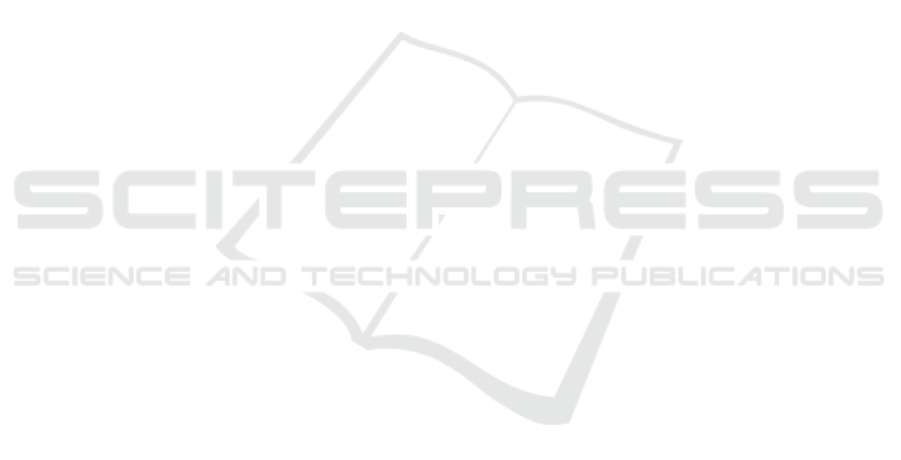
Hasan, R., Crandall, D., Fritz, M., and Kapadia, A. (2020).
Automatically Detecting Bystanders in Photos to Re-
duce Privacy Risks. In 2020 IEEE Symposium on Se-
curity and Privacy (SP), pages 318–335.
Hassanein, K. and Head, M. (2005). The impact of infusing
social presence in the web interface: An investigation
across product types. International Journal of Elec-
tronic Commerce, 10(2):31–55.
Ilia, P., Polakis, I., Athanasopoulos, E., Maggi, F., and Ioan-
nidis, S. (2015). Face/off: Preventing privacy leak-
age from photos in social networks. In Proceedings of
the 22nd ACM SIGSAC Conference on Computer and
Communications Security, pages 781–792.
Jarvenpaa, S. L., Tractinsky, N., and Saarinen, L. (1999).
Consumer Trust in an Internet Store: A Cross-Cultural
Validation. Journal of Computer-Mediated Communi-
cation, 5(2):JCMC526.
Kang, R., Brown, S., Dabbish, L., and Kiesler, S. (2014).
Privacy Attitudes of Mechanical Turk Workers and the
U.S. Public. In 10th Symposium On Usable Privacy
and Security ({SOUPS} 2014), pages 37–49.
Kehr, F., Kowatsch, T., Wentzel, D., and Fleisch, E. (2015).
Blissfully ignorant: The effects of general privacy
concerns, general institutional trust, and affect in the
privacy calculus: Privacy calculus: Dispositions and
affect. Information Systems Journal, 25(6):607–635.
Kim, K. J., Park, E., and Sundar, S. S. (2013). Caregiving
role in human–robot interaction: A study of the medi-
ating effects of perceived benefit and social presence.
Computers in Human Behavior, 29(4):1799–1806.
Kock, N. and Hadaya, P. (2018). Minimum sample size
estimation in PLS-SEM: The inverse square root and
gamma-exponential methods. Information systems
journal, 28(1):227–261.
Korayem, M., Templeman, R., Chen, D., Crandall, D., and
Kapadia, A. (2016). Enhancing Lifelogging Privacy
by Detecting Screens. In Proceedings of the 2016 CHI
Conference on Human Factors in Computing Systems,
CHI ’16, pages 4309–4314, New York, NY, USA. As-
sociation for Computing Machinery.
Li, F., Sun, Z., Li, A., Niu, B., Li, H., and Cao, G. (2019).
HideMe: Privacy-Preserving Photo Sharing on Social
Networks. In IEEE INFOCOM 2019 - IEEE Confer-
ence on Computer Communications, pages 154–162.
Lin, T.-Y., Maire, M., Belongie, S., Hays, J., Perona, P., Ra-
manan, D., Doll
´
ar, P., and Zitnick, C. L. (2014). Mi-
crosoft COCO: Common Objects in Context. In Fleet,
D., Pajdla, T., Schiele, B., and Tuytelaars, T., edi-
tors, Computer Vision – ECCV 2014, Lecture Notes
in Computer Science, pages 740–755. Springer Inter-
national Publishing.
Lindgaard, G., Fernandes, G., Dudek, C., and Brown, J.
(2006). Attention web designers: You have 50 mil-
liseconds to make a good first impression! Behaviour
& information technology, 25(2):115–126.
Mozafari, N., Weiger, W., and Hammerschmidt, M. (2021).
That’s so Embarrassing! When Not to Design for So-
cial Presence in Human-Chatbot Interactions.
Oh, C. S., Bailenson, J. N., and Welch, G. F. (2018). A Sys-
tematic Review of Social Presence: Definition, An-
tecedents, and Implications. Frontiers in Robotics and
AI, 5.
Phelan, C., Lampe, C., and Resnick, P. (2016). It’s Creepy,
But it Doesn’t Bother Me. In Proceedings of the 2016
CHI Conference on Human Factors in Computing Sys-
tems, pages 5240–5251. Association for Computing
Machinery.
Ray, S., Danks, N. P., Valdez, A. C., Estrada, J. M. V., Uan-
horo, J., Nakayama, J., Koyan, L., Burbach, L., Bejar,
A. H. C., and Adler, S. (2022). Seminr: Building and
Estimating Structural Equation Models.
Redmiles, E. M., Kross, S., and Mazurek, M. L. (2019).
How well do my results generalize? comparing secu-
rity and privacy survey results from mturk, web, and
telephone samples. In 2019 IEEE Symposium on Se-
curity and Privacy (SP), pages 1326–1343.
Sarstedt, M., Hair, J. F., Ringle, C. M., Thiele, K. O., and
Gudergan, S. P. (2016). Estimation issues with PLS
and CBSEM: Where the bias lies! Journal of Business
Research, 69(10):3998–4010.
Schaub, F., Marella, A., Kalvani, P., Ur, B., Pan, C., Forney,
E., and Cranor, L. F. (2016). Watching Them Watch-
ing Me: Browser Extensions Impact on User Privacy
Awareness and Concern. In Proceedings 2016 Work-
shop on Usable Security. Internet Society.
Slovic, P., Finucane, M., Peters, E., and MacGregor, D. G.
(2002). Rational actors or rational fools: Implications
of the affect heuristic for behavioral economics. The
Journal of Socio-Economics, 31(4):329–342.
Smith, H. J., Milberg, S. J., and Burke, S. J. (1996).
Information Privacy: Measuring Individuals’ Con-
cerns about Organizational Practices. MIS Quarterly,
20(2):167–196.
Spiekermann, S., Korunovska, J., and Bauer, C. (2012).
Psychology of ownership and asset defense: Why
people value their personal information beyond pri-
vacy. Available at SSRN 2148886.
Spiel, K., Haimson, O. L., and Lottridge, D. (2019). How
to do better with gender on surveys: A guide for HCI
researchers. Interactions, 26(4):62–65.
Staiano, J., Oliver, N., Lepri, B., de Oliveira, R., Caraviello,
M., and Sebe, N. (2014). Money walks: A human-
centric study on the economics of personal mobile
data. In Proceedings of the 2014 ACM International
Joint Conference on Pervasive and Ubiquitous Com-
puting, pages 583–594. ACM.
Xu, H., Dinev, T., Smith, J., and Hart, P. (2011). Infor-
mation Privacy Concerns: Linking Individual Percep-
tions with Institutional Privacy Assurances. Journal
of the Association for Information Systems, 12(12).
Xu, H., Gupta, S., Rosson, M., and Carroll, J. (2012). Mea-
suring Mobile Users’ Concerns for Information Pri-
vacy. In ICIS.
Zhang, B. and Xu, H. (2016). Privacy Nudges for Mo-
bile Applications: Effects on the Creepiness Emotion
and Privacy Attitudes. In Proceedings of the 19th
ACM Conference on Computer-Supported Coopera-
tive Work & Social Computing, CSCW ’16, pages
1676–1690. Association for Computing Machinery.
ICISSP 2023 - 9th International Conference on Information Systems Security and Privacy
676