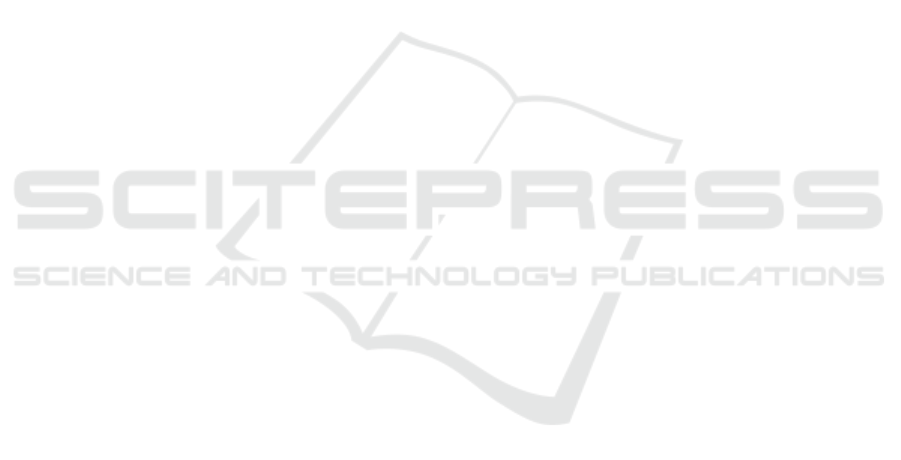
of different spatial complexity. We confirmed that the
proposed weighting function is superior to MLE, al-
though it is not as good as the optimal weighting func-
tion, by employing the number of rectangular regions
according to the depth complexity.
In this study, we theoretically evaluated the pro-
posed estimation method and assumed that the optical
flow noise is ideal white Gaussian noise. The actual
optical flow noise detected has spatial correlation, and
for practical evaluation, it will be necessary to first
detect optical flow in real images with an appropriate
algorithm and then confirm the effectiveness of the
proposed method on them.
REFERENCES
Bickel, P., Klaassen, C. A. J., Ritov, Y., and Wellner, J. A.
(1993). Efficient and adaptive estimation for semi-
parametric models. The Johns Hopkins University
Press, Baltimore and London.
Chaplot, D. S., Salakhutdinov, R., Gupta, A., and Gupta, S.
(2020). Neural topological slam for visual navigation.
In CVPR 2020, pages 12875–12884. IEEE.
Chen, B., Huang, K., Raghupathi, S., Chandratreya, I., Du,
Q., and Lipson, H. (2022). Automated discovery of
fundamental variables hidden in experimental data.
Nature Computational Science, 2:433–442.
Friston, K. J. (2010). The free-energy principle: A unified
brain theory? Nature Review Neuroscience, 11:127–
138.
Friston, K. J., FitzGerald, T., Rigoli, F., Schwartenbeck, P.,
and Pezzulo, G. (2016a). Active inference and learn-
ing. Neuroscience & Biobehavioral Reviews, 68:862–
879.
Friston, K. J., Rosch, R., Parr, T., Price, C., and Bowman, H.
(2016b). Deep temporal models and active inference.
Neuroscience & Biobehavioral Reviews, 77:486–501.
Huang, C. T. (2019). Empirical bayesian light-field stereo
matching by robust pseudo random field modeling.
IEEE Transactions on Pattern Analysis and Machine
Intelligence, 41:552–565.
Hui, T. W. and Chung, R. (2015). Determination shape and
motion from monocular camera: A direct approach
using normal flows. Pattern Recognition, 48(2):422–
437.
Jonschkowsk, R., Stone, A., Barron, J. T., Gordon, A.,
Konolige, K., and Angelova, A. (2020). What matters
in unsupervised optical flow. In ECCV 2020, pages
557–572. Springer.
Maritz, J. S. (2018). Empirical Bayes Methods with Appli-
cations. Chapman and Hall/CRC, Boca Raton, 2nd
edition.
Piloto, L., A.‘Weinstein, Battaglia, P., and Botvinick, M.
(2022). Intuitive physics learning in a deep-learning
model inspired by developmental psychology. Nature
Human Behaviour, 6:1257–1267.
Ranjan, A., Jampani, V., Balles, L., Kim, K., Sun, D., Wulff,
J., and Black, M. J. (2019). Competitive collaboration:
Joint unsupervised learning of depth, camera motion,
optical flow and motion segmentation. In CVPR 2019,
pages 12240–12249. IEEE.
Sekkati, H. and Mitiche, A. (2007). A variational method
for the recovery of dense 3d structure from motion.
Robotics and Autonomous Systems, 55:597–607.
Sroubek, F., Soukup, J., and Zitov
´
a, B. (2016). Varia-
tional bayesian image reconstruction with an uncer-
tainty model for measurement localization. In Euro-
pean Signal Processing Conference. IEEE.
Stone, A., Maurer, D., Ayvaci, A., Angelova, A., and Jon-
schkowski, R. (2021). Smurf: Self-teaching multi-
frame unsupervised raft with full-image warping. In
CVPR 2021, pages 3887–3896. IEEE.
Sumikura, S., Shibuya, M., and Sakurada, K. (2019). Open-
vslam: A versatile visual slam framework. In 27th
ACM International Conference on Multimedia, pages
2292–2295. ACM.
Tagawa, N. (2010). Depth perception model based on fixa-
tional eye movements using bayesian statistical infer-
ence. In International conference on Pattern Recogni-
tion. IEEE.
Tagawa, N., Kawaguchi, J., Naganuma, S., and Okubo, K.
(2008). Direct 3-d shape recovery from image se-
quence based on multi-scale bayesian network. In In-
ternational conference on pattern recognition. IEEE.
Tagawa, N. and Naganuma, S. (2009). Pattern Recognition,
chapter Structure and motion from image sequences
based on multi-scale Bayesian network, pages 73–96.
InTech, Croatia.
Tagawa, N., Toriu, T., and Endoh, T. (1993). Un-biased
linear algorithm for recovering three-dimensional mo-
tion from optical flow. IEICE Trans. Inf. & Sys., E76-
D(10):1263–1275.
Tagawa, N., Toriu, T., and Endoh, T. (1994a). Estimation of
3-d motion from optical flow with unbiased objective
function. IEICE Trans. Inf. & Sys., E77-D(11):1148–
1161.
Tagawa, N., Toriu, T., and Endoh, T. (1994b). An objec-
tive function for 3-d motion estimation from optical
flow with lower error variance than maximum likeli-
hood estimator. In International conference on Image
Processing. IEEE.
Tagawa, N., Toriu, T., and Endoh, T. (1996). 3-d motion
estimation from optical flow with low computational
cost and small variance. IEICE Trans. Inf. & Sys.,
E79-D(3):230–241.
Tateno, K., Tombari, F., Laina, I., and Navab, N. (2017).
Cnn-slam: Real-time dense monocular slam with
learned depth prediction. In CVPR 2017, pages 6243–
6252. IEEE.
Yin, Z. and Shi, J. (2018). Geonet: Unsupervised learn-
ing of dense depth, optical flow and camera pose. In
CVPR 2018, pages 1983–1992. IEEE.
Yuille, A. and Kersten, D. (2006). Vision as bayesian in-
ference: analysis by synthesis? Trends in Cognitive
Sciences, 10:301–308.
On Computing Three-Dimensional Camera Motion from Optical Flow Detected in Two Consecutive Frames
941