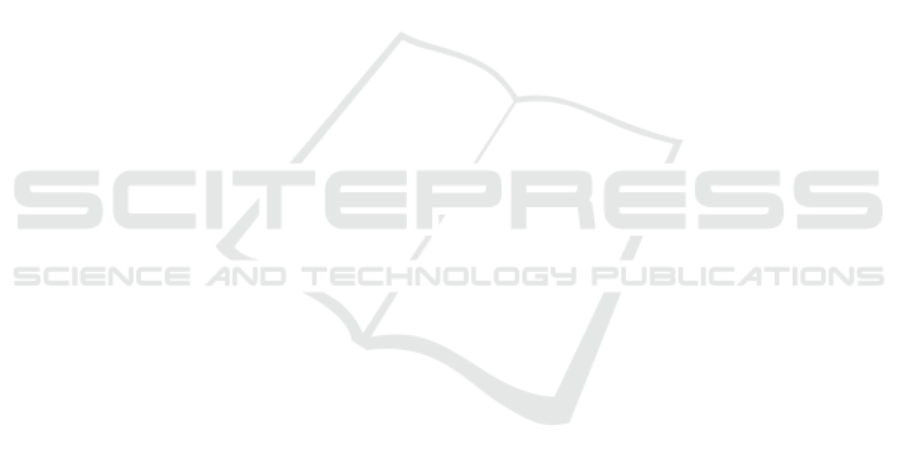
tric human-object interaction tasks. Future work are
related to the improvement of these services as well
as the integration of the next-active object detection
service.
ACKNOWLEDGEMENTS
This research has been supported by Next Vision s.r.l.,
by the project MISE - PON I&C 2014-2020 - Pro-
getto ENIGMA - Prog n. F/190050/02/X44 – CUP:
B61B19000520008 and by MEGABIT - PIAno di in-
CEntivi per la RIcerca di Ateneo 2020/2022 (PIAC-
ERI) – linea di intervento 2, DMI - University of Cata-
nia.
REFERENCES
Brachmann, E. and Rother, C. (2018). Learning less is more
- 6d camera localization via 3d surface regression. In
Proceedings of the IEEE Conference on Computer Vi-
sion and Pattern Recognition (CVPR).
Colombo, S., Lim, Y., and Casalegno, F. (2019). Deep vi-
sion shield: Assessing the use of hmd and wearable
sensors in a smart safety device. In ACM PETRA.
Cucchiara, R. and Bimbo, A. D. (2014). Visions for aug-
mented cultural heritage experience. IEEE MultiMe-
dia, 21(1):74–82.
Damen, D., Leelasawassuk, T., Haines, O., Calway, A., and
Mayol-Cuevas, W. (2014). You-do, i-learn: Discover-
ing task relevant objects and their modes of interaction
from multi-user egocentric video. In BMVC.
Farinella, G. M., Signorello, G., Battiato, S., Furnari, A.,
Ragusa, F., Leonardi, R., Ragusa, E., Scuderi, E.,
Lopes, A., Santo, L., and Samarotto, M. (2019). Vedi:
Vision exploitation for data interpretation. In ICIAP.
Furnari, A., Battiato, S., and Farinella, G. M. (2018).
Personal-location-based temporal segmentation of
egocentric video for lifelogging applications. Journal
of Visual Communication and Image Representation,
52:1–12.
Girshick, R. (2015). Fast R-CNN. In ICCV.
Gkioxari, G., Girshick, R. B., Doll
´
ar, P., and He, K. (2018).
Detecting and recognizing human-object interactions.
CVPR, pages 8359–8367.
Gupta, S. and Malik, J. (2015). Visual semantic role label-
ing. ArXiv, abs/1505.04474.
Gurevich, P., Lanir, J., Cohen, B., and Stone, R. (2012).
Teleadvisor: a versatile augmented reality tool for re-
mote assistance. Proceedings of the SIGCHI Confer-
ence on Human Factors in Computing Systems.
Hoffer, E. and Ailon, N. (2015). Deep metric learning us-
ing triplet network. In Feragen, A., Pelillo, M., and
Loog, M., editors, Similarity-Based Pattern Recogni-
tion, pages 84–92. Springer International Publishing.
Leonardi, R., Ragusa, F., Furnari, A., and Farinella, G. M.
(2022). Egocentric human-object interaction detection
exploiting synthetic data.
Lin, T. Y., Maire, M., Belongie, S., Bourdev, L., Girshick,
R., Hays, J., Perona, P., Ramanan, D., Zitnick, C. L.,
and Doll
´
ar, P. (2014). Microsoft coco: Common ob-
jects in context.
Nagarajan, T., Feichtenhofer, C., and Grauman, K. (2019).
Grounded human-object interaction hotspots from
video. In ICCV, pages 8687–8696.
Nagarajan, T., Li, Y., Feichtenhofer, C., and Grauman, K.
(2020). Ego-topo: Environment affordances from
egocentric video. ArXiv, abs/2001.04583.
Osti, F., de Amicis, R., Sanchez, C. A., Tilt, A. B., Prather,
E., and Liverani, A. (2021). A vr training system
for learning and skills development for construction
workers. Virtual Reality, 25:523–538.
Ragusa, F., Furnari, A., Battiato, S., Signorello, G., and
Farinella, G. M. (2020). EGO-CH: Dataset and fun-
damental tasks for visitors behavioral understanding
using egocentric vision. Pattern Recognition Letters.
Ragusa, F., Furnari, A., and Farinella, G. M. (2022). Mec-
cano: A multimodal egocentric dataset for humans be-
havior understanding in the industrial-like domain.
Ragusa, F., Furnari, A., Livatino, S., and Farinella, G. M.
(2021). The meccano dataset: Understanding human-
object interactions from egocentric videos in an
industrial-like domain. In IEEE Winter Conference
on Application of Computer Vision (WACV).
Rebol, M., Hood, C., Ranniger, C., Rutenberg, A., Sikka,
N., Horan, E. M., G
¨
utl, C., and Pietroszek, K. (2021).
Remote assistance with mixed reality for procedural
tasks. 2021 IEEE Conference on Virtual Reality and
3D User Interfaces Abstracts and Workshops (VRW),
pages 653–654.
Redmon, J. and Farhadi, A. (2018). Yolov3: An incremental
improvement. CoRR, abs/1804.02767.
Ren, S., He, K., Girshick, R., and Sun, J. (2015). Faster R-
CNN: Towards real-time object detection with region
proposal networks. In NeurIPS, pages 91–99.
Seidenari, L., Baecchi, C., Uricchio, T., Ferracani, A.,
Bertini, M., and Bimbo, A. D. (2017). Deep art-
work detection and retrieval for automatic context-
aware audio guides. ACM Transactions on Multime-
dia Computing, Communications, and Applications,
13(3s):35.
Shan, D., Geng, J., Shu, M., and Fouhey, D. (2020). Under-
standing human hands in contact at internet scale. In
CVPR.
Sorko, S. R. and Brunnhofer, M. (2019). Potentials of aug-
mented reality in training. Procedia Manufacturing.
Sun, L., Osman, H. A., and Lang, J. (2021). An aug-
mented reality online assistance platform for repair
tasks. ACM Transactions on Multimedia Computing,
Communications, and Applications (TOMM), 17:1 –
23.
Taira, H., Okutomi, M., Sattler, T., Cimpoi, M., Pollefeys,
M., Sivic, J., Pajdla, T., and Torii, A. (2018). Inloc: In-
door visual localization with dense matching and view
ENIGMA: Egocentric Navigator for Industrial Guidance, Monitoring and Anticipation
701