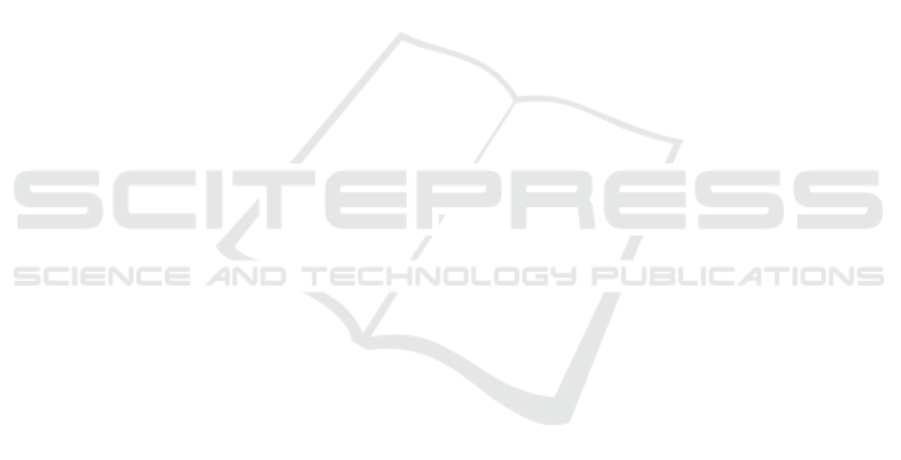
North American Chapter of the Association for Com-
putational Linguistics: Human Language Technolo-
gies, pages 624–643, Online. Association for Compu-
tational Linguistics.
Shen, J., Pang, R., Weiss, R. J., Schuster, M., Jaitly, N.,
Yang, Z., Chen, Z., Zhang, Y., Wang, Y., Skerrv-Ryan,
R., Saurous, R. A., Agiomvrgiannakis, Y., and Wu, Y.
(2018). Natural tts synthesis by conditioning wavenet
on mel spectrogram predictions. volume 2018-April.
Shu, K., Cui, L., Wang, S., Lee, D., and Liu, H. (2019). dE-
FEND: Explainable fake news detection. In Proceed-
ings of the 25th ACM SIGKDD International Confer-
ence on Knowledge Discovery & Data Mining, KDD
’19, page 395–405, New York, NY, USA. Association
for Computing Machinery.
Shu, K., Mahudeswaran, D., Wang, S., Lee, D., and Liu,
H. (2018). Fakenewsnet: A data repository with
news content, social context and dynamic informa-
tion for studying fake news on social media. ArXiv,
abs/1809.01286.
Silva, A., Han, Y., Luo, L., Karunasekera, S., and Leckie,
C. (2021). Propagation2vec: Embedding partial prop-
agation networks for explainable fake news early
detection. Information Processing & Management,
58(5):102618.
Singh, V. K., Ghosh, I., and Sonagara, D. (2021). Detecting
fake news stories via multimodal analysis. Journal of
the Association for Information Science and Technol-
ogy, 72(1):3–17.
Singhal, S., Shah, R. R., Chakraborty, T., Kumaraguru, P.,
and Satoh, S. (2019). Spotfake: A multi-modal frame-
work for fake news detection. In 2019 IEEE fifth inter-
national conference on multimedia big data (BigMM),
pages 39–47. IEEE.
Suresh, H. and Guttag, J. (2021). A framework for un-
derstanding sources of harm throughout the machine
learning life cycle. In Equity and access in algorithms,
mechanisms, and optimization, pages 1–9.
Szczepa
´
nski, M., Pawlicki, M., Kozik, R., and Chora
´
s, M.
(2021). New explainability method for bert-based
model in fake news detection. Scientific Reports,
11(1):1–13.
Tan, R., Plummer, B. A., and Saenko, K. (2020). Detect-
ing cross-modal inconsistency to defend against neu-
ral fake news.
Tenney, I., Wexler, J., Bastings, J., Bolukbasi, T., Coenen,
A., Gehrmann, S., Jiang, E., Pushkarna, M., Rade-
baugh, C., Reif, E., and Yuan, A. (2020). The lan-
guage interpretability tool: Extensible, interactive vi-
sualizations and analysis for NLP models. In Proceed-
ings of the 2020 Conference on Empirical Methods
in Natural Language Processing: System Demonstra-
tions, pages 107–118, Online. Association for Com-
putational Linguistics.
Thorne, J., Vlachos, A., Christodoulopoulos, C., and Mit-
tal, A. (2018). FEVER: a large-scale dataset for fact
extraction and VERification. In Proceedings of the
2018 Conference of the North American Chapter of
the Association for Computational Linguistics: Hu-
man Language Technologies, Volume 1 (Long Papers),
pages 809–819, New Orleans, Louisiana. Association
for Computational Linguistics.
Thyagharajan, K. and Kalaiarasi, G. (2021). A review on
near-duplicate detection of images using computer vi-
sion techniques. Archives of Computational Methods
in Engineering, 28(3):897–916.
Tschiatschek, S., Singla, A., Rodriguez, M. G., Merchant,
A., and Krause, A. (2018). Fake news detection in
social networks via crowd signals.
van der Waa, J., Nieuwburg, E., Cremers, A., and Neer-
incx, M. (2021). Evaluating xai: A comparison of
rule-based and example-based explanations. Artificial
Intelligence, 291:103404.
Vilone, G. and Longo, L. (2021). Notions of explainability
and evaluation approaches for explainable artificial in-
telligence. Information Fusion, 76:89–106.
Vogel, I. and Jiang, P. (2019). Fake news detection with
the new german dataset “germanfakenc”. In Dig-
ital Libraries for Open Knowledge: 23rd Interna-
tional Conference on Theory and Practice of Digital
Libraries, TPDL 2019, Oslo, Norway, September 9-
12, 2019, Proceedings, page 288–295, Berlin, Heidel-
berg. Springer-Verlag.
Wadhwa, S., Khetan, V., Amir, S., and Wallace, B. (2022).
Redhot: A corpus of annotated medical questions, ex-
periences, and claims on social media.
Wang, W. Y. (2017). “liar, liar pants on fire”: A new bench-
mark dataset for fake news detection. In Proceed-
ings of the 55th Annual Meeting of the Association for
Computational Linguistics (Volume 2: Short Papers),
pages 422–426, Vancouver, Canada. Association for
Computational Linguistics.
Wiegreffe, S., Hessel, J., Swayamdipta, S., Riedl, M., and
Choi, Y. (2022). Reframing human-AI collaboration
for generating free-text explanations. In Proceedings
of the 2022 Conference of the North American Chap-
ter of the Association for Computational Linguistics:
Human Language Technologies, pages 632–658, Seat-
tle, United States. Association for Computational Lin-
guistics.
Yang, F., Pentyala, S. K., Mohseni, S., Du, M., Yuan, H.,
Linder, R., Ragan, E. D., Ji, S., and Hu, X. B. (2019).
Xfake: Explainable fake news detector with visual-
izations. In The World Wide Web Conference, WWW
’19, page 3600–3604, New York, NY, USA. Associa-
tion for Computing Machinery.
Yao, B. M., Shah, A., Sun, L., Cho, J.-H., and Huang, L.
(2022). End-to-end multimodal fact-checking and ex-
planation generation: A challenging dataset and mod-
els. arXiv preprint arXiv:2205.12487.
Zellers, R., Holtzman, A., Rashkin, H., Bisk, Y., Farhadi,
A., Roesner, F., and Choi, Y. (2019). Defending
against neural fake news. In Advances in Neural In-
formation Processing Systems, volume 32. Curran As-
sociates, Inc.
Zhao, H., Zhou, W., Chen, D., Wei, T., Zhang, W., and
Yu, N. (2021). Multi-attentional deepfake detection.
In Proceedings of the IEEE/CVF conference on com-
puter vision and pattern recognition, pages 2185–
2194.
Fighting Disinformation: Overview of Recent AI-Based Collaborative Human-Computer Interaction for Intelligent Decision Support
Systems
277