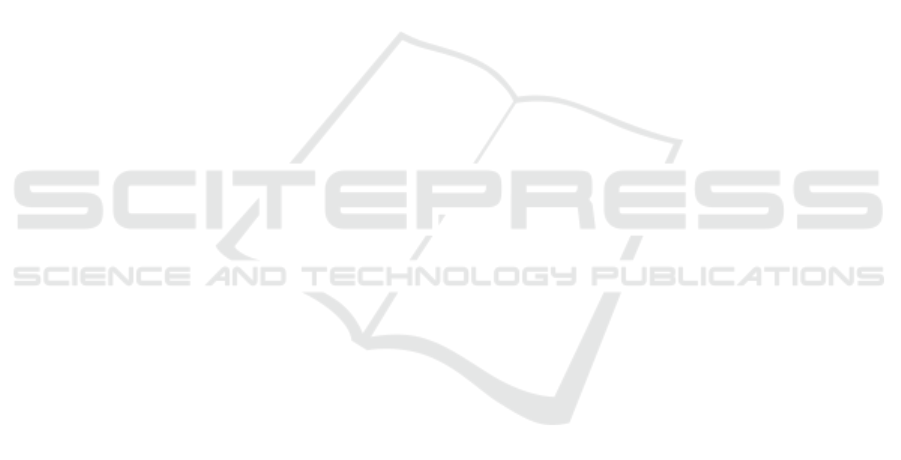
255–258, New York, NY, USA. Association for Com-
puting Machinery.
Funes Mora, K. A. and Odobez, J.-M. (2014). Geomet-
ric generative gaze estimation (g¡sup¿3¡/sup¿e) for
remote rgb-d cameras. In 2014 IEEE Conference
on Computer Vision and Pattern Recognition, pages
1773–1780.
Guestrin, E. and Eizenman, M. (2006). General theory
of remote gaze estimation using the pupil center and
corneal reflections. IEEE Transactions on Biomedical
Engineering, 53(6):1124–1133.
Hansen, D. W. and Ji, Q. (2010). In the eye of the beholder:
A survey of models for eyes and gaze. IEEE Trans-
actions on Pattern Analysis and Machine Intelligence,
32(3):478–500.
He, K., Zhang, X., Ren, S., and Sun, J. (2015). Deep
residual learning for image recognition. CoRR,
abs/1512.03385.
Heo, B., Yun, S., Han, D., Chun, S., Choe, J., and Oh, S. J.
(2021). Rethinking spatial dimensions of vision trans-
formers. In International Conference on Computer Vi-
sion (ICCV).
Hoppe, S., Loetscher, T., Morey, S. A., and Bulling, A.
(2018). Eye movements during everyday behavior
predict personality traits. Frontiers in Human Neu-
roscience, 12:105.
Huang, C.-M. and Mutlu, B. (2016). Anticipatory robot
control for efficient human-robot collaboration. In
2016 11th ACM/IEEE International Conference on
Human-Robot Interaction (HRI), pages 83–90.
Kellnhofer, P., Recasens, A., Stent, S., Matusik, W., and
Torralba, A. (2019). Gaze360: Physically uncon-
strained gaze estimation in the wild. In IEEE Inter-
national Conference on Computer Vision (ICCV).
King, D. E. (2009). Dlib-ml: A machine learning toolkit.
Journal of Machine Learning Research, 10:1755–
1758.
Kingma, D. P. and Ba, J. (2017). Adam: A method for
stochastic optimization.
Kirillov, A., Girshick, R. B., He, K., and Doll
´
ar, P. (2019).
Panoptic feature pyramid networks. 2019 IEEE/CVF
Conference on Computer Vision and Pattern Recogni-
tion (CVPR), pages 6392–6401.
Konrad, R., Angelopoulos, A., and Wetzstein, G. (2019).
Gaze-contingent ocular parallax rendering for virtual
reality. CoRR, abs/1906.09740.
Krafka, K., Khosla, A., Kellnhofer, P., Kannan, H., Bhan-
darkar, S. M., Matusik, W., and Torralba, A. (2016).
Eye tracking for everyone. CoRR, abs/1606.05814.
L R D, M. and Biswas, P. (2021). Appearance-based gaze
estimation using attention and difference mechanism.
In 2021 IEEE/CVF Conference on Computer Vision
and Pattern Recognition Workshops (CVPRW), pages
3137–3146.
Lecun, Y., Bottou, L., Bengio, Y., and Haffner, P. (1998).
Gradient-based learning applied to document recogni-
tion. Proceedings of the IEEE, 86(11):2278–2324.
Li, P., Hou, X., Duan, X., Yip, H., Song, G., and Liu, Y.
(2019). Appearance-based gaze estimator for natural
interaction control of surgical robots. IEEE Access,
7:25095–25110.
Lorenz., O. and Thomas., U. (2019a). Real time eye gaze
tracking system using cnn-based facial features for hu-
man attention measurement. In Proceedings of the
14th International Joint Conference on Computer Vi-
sion, Imaging and Computer Graphics Theory and
Applications - Volume 5: VISAPP,, pages 598–606.
INSTICC, SciTePress.
Lorenz., O. and Thomas., U. (2019b). Real time eye gaze
tracking system using cnn-based facial features for hu-
man attention measurement. In Proceedings of the
14th International Joint Conference on Computer Vi-
sion, Imaging and Computer Graphics Theory and
Applications - Volume 5: VISAPP,, pages 598–606.
INSTICC, SciTePress.
Lu, F., Sugano, Y., Okabe, T., and Sato, Y. (2014). Adap-
tive linear regression for appearance-based gaze esti-
mation. IEEE Transactions on Pattern Analysis and
Machine Intelligence.
Martinez, F., Carbone, A., and Pissaloux, E. (2012). Gaze
estimation using local features and non-linear regres-
sion. In 2012 19th IEEE International Conference on
Image Processing, pages 1961–1964.
Nakazawa, A. and Nitschke, C. (2012). Point of gaze esti-
mation through corneal surface reflection in an active
illumination environment. In Fitzgibbon, A., Lazeb-
nik, S., Perona, P., Sato, Y., and Schmid, C., edi-
tors, Computer Vision – ECCV 2012, pages 159–172,
Berlin, Heidelberg. Springer Berlin Heidelberg.
Park, S., Mello, S. D., Molchanov, P., Iqbal, U., Hilliges,
O., and Kautz, J. (2019). Few-shot adaptive gaze esti-
mation.
Patney, A., Salvi, M., Kim, J., Kaplanyan, A., Wyman, C.,
Benty, N., Luebke, D., and Lefohn, A. (2016). To-
wards foveated rendering for gaze-tracked virtual re-
ality. ACM Trans. Graph., 35(6).
Simonyan, K. and Zisserman, A. (2014). Very deep con-
volutional networks for large-scale image recognition.
CoRR, abs/1409.1556.
Smith, B., Yin, Q., Feiner, S., and Nayar, S. (2013).
Gaze Locking: Passive Eye Contact Detection for Hu-
man?Object Interaction. In ACM Symposium on User
Interface Software and Technology (UIST), pages
271–280.
Tan, K.-H., Kriegman, D. J., and Ahuja, N. (2002).
Appearance-based eye gaze estimation. In Proceed-
ings of the Sixth IEEE Workshop on Applications of
Computer Vision, WACV ’02, page 191, USA. IEEE
Computer Society.
Valenti, R., Sebe, N., and Gevers, T. (2012). Combining
head pose and eye location information for gaze es-
timation. IEEE Transactions on Image Processing,
21(2):802–815.
Vaswani, A., Shazeer, N., Parmar, N., Uszkoreit, J.,
Jones, L., Gomez, A. N., Kaiser, L., and Polo-
sukhin, I. (2017). Attention is all you need. CoRR,
abs/1706.03762.
Wang, H., Dong, X., Chen, Z., and Shi, B. E. (2015). Hy-
brid gaze/eeg brain computer interface for robot arm
VISAPP 2023 - 18th International Conference on Computer Vision Theory and Applications
548